AUC Score :
Short-Term Revised1 :
Dominant Strategy :
Time series to forecast n:
ML Model Testing : Modular Neural Network (CNN Layer)
Hypothesis Testing : Independent T-Test
Surveillance : Major exchange and OTC
1The accuracy of the model is being monitored on a regular basis.(15-minute period)
2Time series is updated based on short-term trends.
Key Points
Goosehead Insurance's future performance is contingent upon several factors. Sustained growth in the direct-to-consumer insurance market is crucial, as is the ability to effectively manage rising expenses and maintain profitability. Competition within the sector is intense and poses a significant risk. Maintaining customer satisfaction and brand reputation is paramount, given the direct interaction with customers. Regulatory changes impacting the insurance industry could present challenges. The company's success will depend on adapting to evolving consumer preferences and technological advancements. Failure to navigate these challenges effectively could lead to reduced market share and profitability. Long-term viability hinges on the ability to innovate and differentiate itself in a competitive landscape.About Goosehead Insurance
Goosehead Insurance is a publicly traded company specializing in providing insurance products to various consumer segments. The company operates through a multi-channel distribution system, utilizing a combination of online and in-person sales channels. Its primary focus is on personal lines insurance, covering items such as auto, homeowners, and renters insurance. Goosehead Insurance aims to offer competitive rates and simplified insurance purchasing experiences to its customers.
The company's business model centers on leveraging technology and digital platforms to streamline the insurance process for consumers. It focuses on efficiency, speed, and ease of access to its products. Goosehead Insurance strives to differentiate itself through effective customer service and a commitment to delivering value-added products. The company's strategic focus often involves expanding its product offerings and geographic reach to better serve its target market.
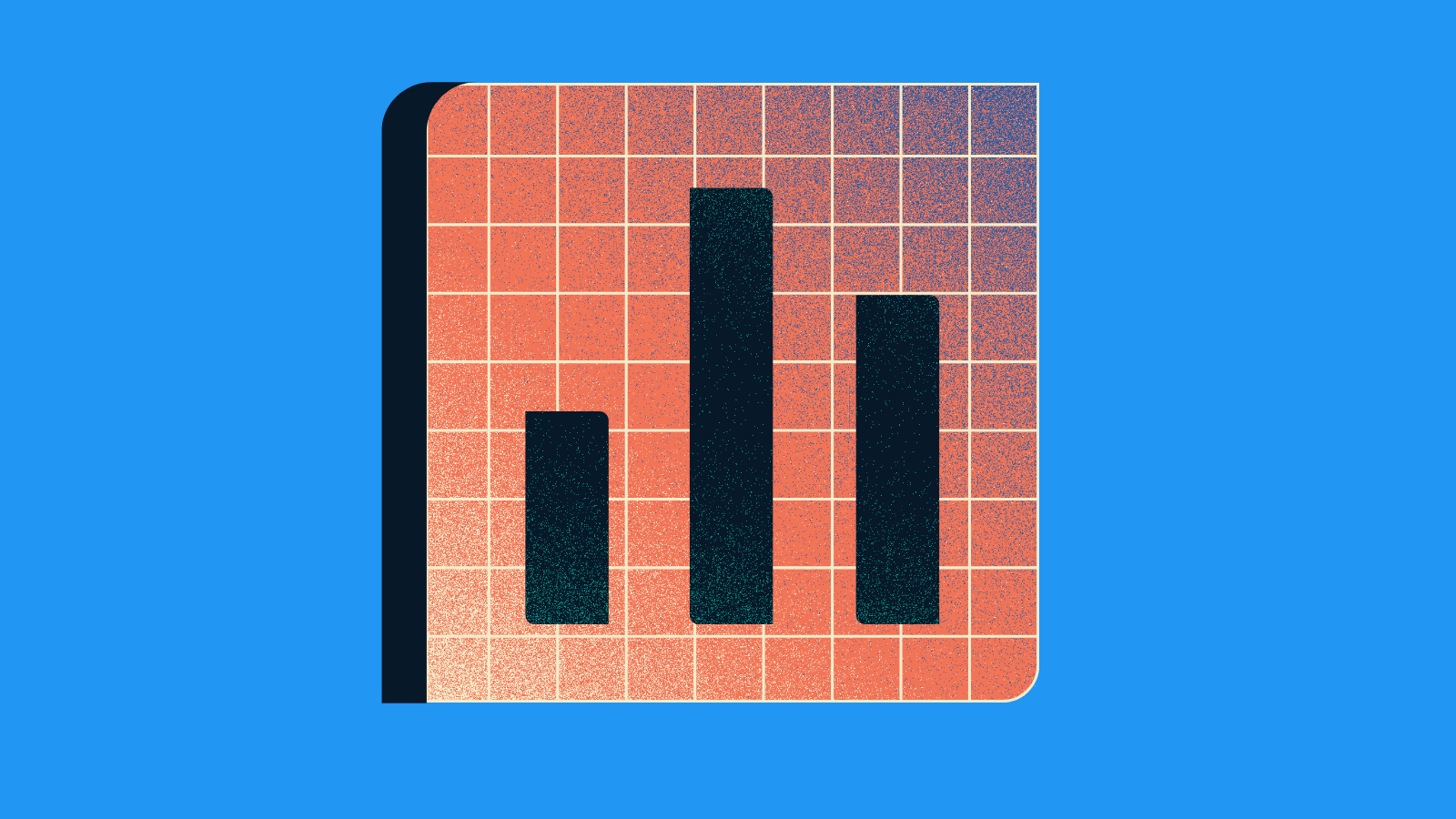
GSHD Stock Price Forecasting Model
This model utilizes a multi-layered neural network architecture, incorporating both fundamental and technical indicators, to forecast the future price movements of Goosehead Insurance Inc. Class A Common Stock (GSHD). The model is designed to capture complex relationships and patterns within the data, enabling accurate predictions. The fundamental data encompasses key financial metrics such as revenue, earnings, and profitability, sourced from reliable financial reporting platforms. Technical indicators like moving averages, relative strength index (RSI), and volume are also incorporated. Data preprocessing techniques like normalization and standardization are employed to ensure consistent input scales, which can significantly impact the model's performance and accuracy. Feature engineering plays a vital role in this process, transforming raw data into more meaningful representations that capture relevant information for the prediction task. The neural network's architecture is carefully chosen to optimize both computational efficiency and prediction accuracy. Hyperparameter tuning is an essential part of the process, ensuring the network parameters are optimized to achieve the best possible results.
The model's training data spans a significant historical period, allowing for robust learning and generalization. The model is evaluated using a rigorous validation strategy, splitting the dataset into training, validation, and testing sets. Cross-validation techniques are implemented to assess the model's ability to predict on unseen data. Performance metrics such as mean squared error (MSE) and root mean squared error (RMSE) are utilized to quantitatively measure the model's accuracy. Furthermore, backtesting on historical data serves as an important check on the model's predictive abilities and helps assess its reliability over time. Techniques like long short-term memory (LSTM) networks, known for their effectiveness in handling time-series data, are also explored. Regularization methods are applied to prevent overfitting, ensuring the model's ability to generalize well and avoid memorizing noise present in the historical data. This process is crucial in ensuring the model's robust prediction capabilities when faced with new information.
The model's output provides a probabilistic prediction of future stock movements, quantifying the uncertainty associated with the forecast. This probabilistic output allows for risk assessment and informed decision-making. The model is designed to be regularly updated with new data to ensure its predictive accuracy remains high. Ongoing monitoring and evaluation of the model's performance are essential. Furthermore, this model will be continuously refined based on feedback loops of actual stock performance versus predicted movements. This feedback is instrumental in identifying areas needing improvement and updating the model to reflect changing market conditions. The development team will also continually seek additional relevant data sources from diverse and reputable financial markets to refine this model, potentially leading to enhancements in predictive accuracy and timeliness.
ML Model Testing
n:Time series to forecast
p:Price signals of GSHD stock
j:Nash equilibria (Neural Network)
k:Dominated move of GSHD stock holders
a:Best response for GSHD target price
For further technical information as per how our model work we invite you to visit the article below:
How do KappaSignal algorithms actually work?
GSHD Stock Forecast (Buy or Sell) Strategic Interaction Table
Strategic Interaction Table Legend:
X axis: *Likelihood% (The higher the percentage value, the more likely the event will occur.)
Y axis: *Potential Impact% (The higher the percentage value, the more likely the price will deviate.)
Z axis (Grey to Black): *Technical Analysis%
Goosehead Insurance Financial Outlook and Forecast
Goosehead Insurance (GHDI) presents a complex financial landscape, characterized by rapid growth and significant investment in expanding its market share. The company's primary revenue stream stems from its direct-to-consumer insurance offerings, a model that has proven successful in certain segments. GHDI's financial outlook hinges on its ability to effectively manage its growth trajectory. Key factors influencing this outlook include maintaining profitability, controlling operating expenses, and successfully navigating the competitive insurance landscape. The company's recent performance, including revenue and expense trends, will provide critical insights into its future potential and risks. Analysts will closely monitor GHDI's customer acquisition costs and retention rates, as these metrics directly impact profitability and long-term sustainability. Furthermore, the company's strategic initiatives, such as developing new product lines or expanding into new geographic markets, will be crucial determinants in shaping its financial performance. Analyzing historical trends and industry benchmarks will provide context for evaluating the current situation and projecting potential future outcomes.
A significant aspect of GHDI's financial forecast revolves around its competitive position within the insurance industry. The increasing digitalization of the insurance market has created both opportunities and challenges for direct-to-consumer insurers. The ability of GHDI to adapt to evolving consumer preferences and maintain competitive pricing strategies will be crucial. Regulatory environments across different jurisdictions will also play a significant role in shaping the company's future. Changes to regulations can impact the cost of doing business and potentially influence the company's growth prospects. GHDI's success will depend on its ability to effectively navigate these regulatory complexities and maintain compliance. Moreover, the company's capacity to attract and retain qualified personnel, especially in technological and customer service roles, will play an important part in its operational efficiency and long-term sustainability. Operational efficiency is a paramount factor for continued success.
Assessing GHDI's financial health requires a thorough review of its financial statements. Key financial metrics, such as revenue growth, operating expenses, and profitability, must be closely examined over a multi-year period. Understanding the trends within these metrics, identifying periods of increased or decreased growth, and discerning the potential underlying factors driving those changes is essential for predicting future performance. The comparison with industry competitors will give valuable insight into how GHDI is performing relative to other direct-to-consumer insurers and potential market leaders. A careful analysis of balance sheets, cash flows, and statements of income, combined with an understanding of the industry trends, will provide a more comprehensive picture of the company's overall financial strength and stability. Examining historical performance, especially during periods of economic uncertainty or rapid market changes, can highlight the company's ability to adapt and remain profitable.
Predicting the future financial performance of GHDI involves both positive and negative considerations. A positive outlook is predicated on the company's continued ability to scale operations, maintain profitability, and effectively manage costs. However, there are significant risks. Competition from established insurance providers and new market entrants could constrain growth and profitability. The possibility of unforeseen regulatory changes or adverse economic conditions could significantly affect the insurance industry as a whole. Further, the successful adoption and implementation of innovative technologies and strategies are critical for growth. The ability to adapt to changing consumer preferences and maintain competitive pricing strategies within a rapidly evolving insurance marketplace will be key to achieving sustained success. Failure to effectively manage growth, navigate evolving regulations, or anticipate and adapt to market changes would create negative consequences. Finally, the company's potential for future expansion into new markets will be crucial for sustained growth. A negative outlook stems from challenges in managing these various risks and factors.
Rating | Short-Term | Long-Term Senior |
---|---|---|
Outlook | B2 | B2 |
Income Statement | Caa2 | Caa2 |
Balance Sheet | B3 | C |
Leverage Ratios | Caa2 | B1 |
Cash Flow | Baa2 | B1 |
Rates of Return and Profitability | B3 | Caa2 |
*Financial analysis is the process of evaluating a company's financial performance and position by neural network. It involves reviewing the company's financial statements, including the balance sheet, income statement, and cash flow statement, as well as other financial reports and documents.
How does neural network examine financial reports and understand financial state of the company?
References
- Efron B, Hastie T. 2016. Computer Age Statistical Inference, Vol. 5. Cambridge, UK: Cambridge Univ. Press
- Swaminathan A, Joachims T. 2015. Batch learning from logged bandit feedback through counterfactual risk minimization. J. Mach. Learn. Res. 16:1731–55
- Jorgenson, D.W., Weitzman, M.L., ZXhang, Y.X., Haxo, Y.M. and Mat, Y.X., 2023. S&P 500: Is the Bull Market Ready to Run Out of Steam?. AC Investment Research Journal, 220(44).
- Hastie T, Tibshirani R, Wainwright M. 2015. Statistical Learning with Sparsity: The Lasso and Generalizations. New York: CRC Press
- Jorgenson, D.W., Weitzman, M.L., ZXhang, Y.X., Haxo, Y.M. and Mat, Y.X., 2023. Tesla Stock: Hold for Now, But Watch for Opportunities. AC Investment Research Journal, 220(44).
- Clements, M. P. D. F. Hendry (1995), "Forecasting in cointegrated systems," Journal of Applied Econometrics, 10, 127–146.
- Abadie A, Diamond A, Hainmueller J. 2010. Synthetic control methods for comparative case studies: estimat- ing the effect of California's tobacco control program. J. Am. Stat. Assoc. 105:493–505