AUC Score :
Short-Term Revised1 :
Dominant Strategy :
Time series to forecast n:
ML Model Testing : Modular Neural Network (Speculative Sentiment Analysis)
Hypothesis Testing : Lasso Regression
Surveillance : Major exchange and OTC
1The accuracy of the model is being monitored on a regular basis.(15-minute period)
2Time series is updated based on short-term trends.
Key Points
Getty Realty is likely to experience continued growth in the near future due to the strong demand for single-tenant retail properties. The company has a robust portfolio of high-quality properties, a strong balance sheet, and a track record of successful acquisitions. However, rising interest rates and potential economic slowdowns pose risks to Getty Realty's performance, as these factors could impact tenant demand and property values. Furthermore, increased competition from other real estate investment trusts (REITs) could also pressure the company's growth prospects.About Getty Realty
Getty Realty Corporation, a publicly traded real estate investment trust (REIT), specializes in owning and managing properties leased to convenience stores and gas stations. The company has a diverse portfolio of properties located across the United States, primarily focusing on gasoline stations and convenience stores. Getty Realty focuses on acquiring and owning single-tenant properties, which offer stable income streams through long-term leases. The company's strategy revolves around investing in high-quality properties situated in strategic locations, providing a foundation for reliable returns.
Getty Realty's business model is built upon its expertise in property management and its strong relationships with its tenants. The company actively manages its properties, ensuring their upkeep and maximizing their value. Its focus on the convenience store and gasoline station sectors leverages the essential nature of these businesses, providing consistent demand for the properties Getty Realty owns. This approach positions the company for continued growth and value creation for its shareholders.
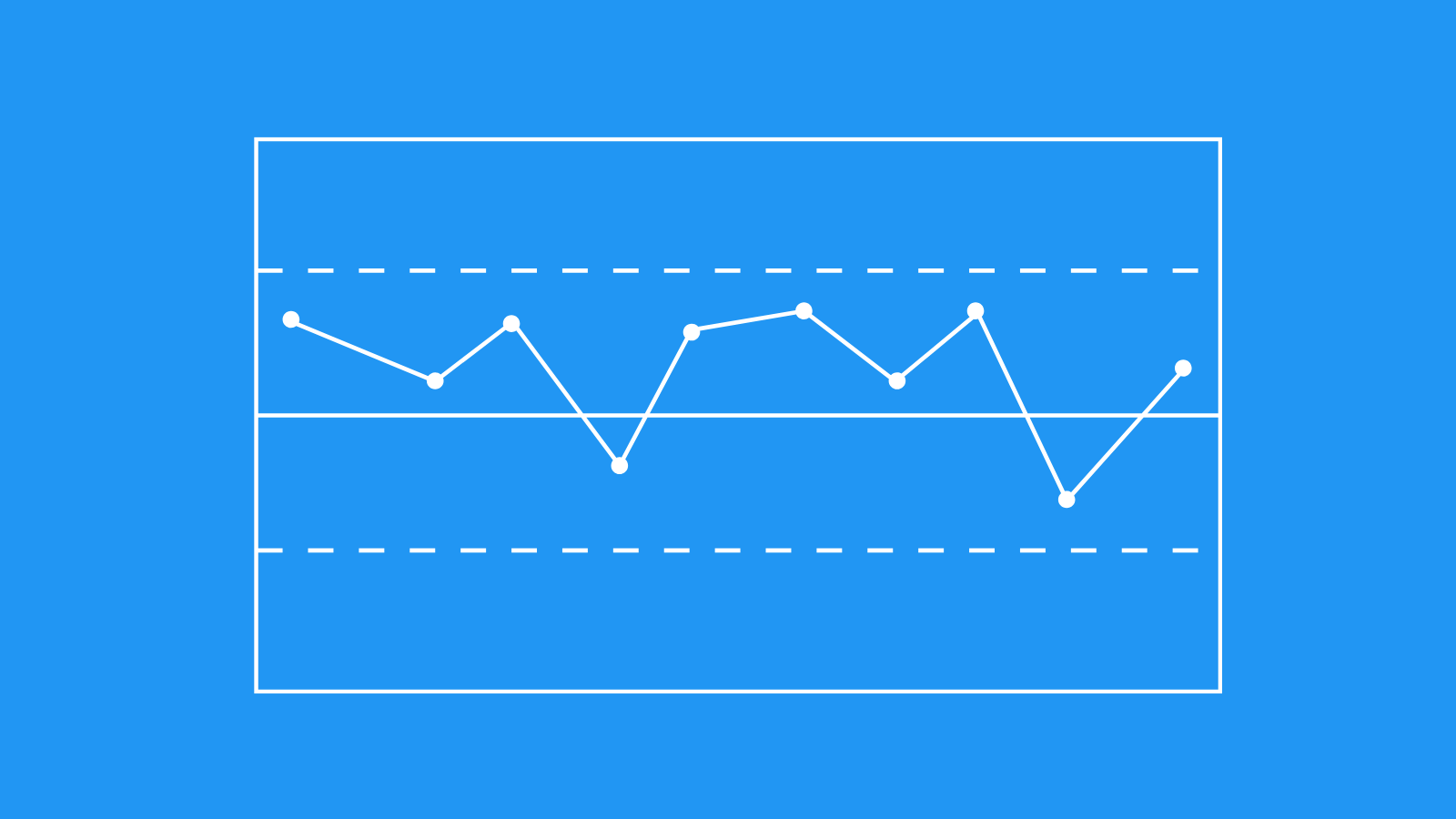
Predicting the Trajectory of Getty Realty Corporation: A Machine Learning Approach
To accurately predict the future trajectory of Getty Realty Corporation (GTY) common stock, our team of data scientists and economists will leverage a sophisticated machine learning model. This model will draw upon a robust dataset encompassing historical stock prices, financial statements, macroeconomic indicators, and industry-specific data. By analyzing these factors, we aim to identify patterns and correlations that influence GTY's stock performance. Our model will employ a combination of supervised and unsupervised learning techniques, including linear regression, support vector machines, and recurrent neural networks, to capture the complex relationships between variables and predict future stock price movements. This multi-faceted approach will provide a comprehensive and nuanced understanding of GTY's stock dynamics.
Our model will be further enhanced by incorporating sentiment analysis of news articles and social media posts related to GTY and the real estate industry. By identifying positive and negative sentiment surrounding GTY's performance, we can gain valuable insights into market expectations and investor confidence, thereby improving the model's predictive accuracy. Additionally, we will implement a dynamic feature selection process to prioritize the most relevant variables based on real-time market conditions. This adaptability ensures that our model remains responsive to changes in the market and adjusts its predictions accordingly.
Through rigorous testing and validation, we aim to develop a reliable and accurate model capable of providing valuable insights into GTY's stock performance. This model will assist investors in making informed decisions, while also offering Getty Realty Corporation valuable information about the factors driving its stock performance. By utilizing cutting-edge machine learning techniques, we aim to unlock the complexities of stock prediction and empower stakeholders with data-driven insights.
ML Model Testing
n:Time series to forecast
p:Price signals of GTY stock
j:Nash equilibria (Neural Network)
k:Dominated move of GTY stock holders
a:Best response for GTY target price
For further technical information as per how our model work we invite you to visit the article below:
How do KappaSignal algorithms actually work?
GTY Stock Forecast (Buy or Sell) Strategic Interaction Table
Strategic Interaction Table Legend:
X axis: *Likelihood% (The higher the percentage value, the more likely the event will occur.)
Y axis: *Potential Impact% (The higher the percentage value, the more likely the price will deviate.)
Z axis (Grey to Black): *Technical Analysis%
Getty Realty: A Stable Future with Growth Potential
Getty Realty (GTY) is a real estate investment trust (REIT) that specializes in owning and leasing properties to convenience stores, restaurants, and other retail businesses. The company boasts a long history of steady performance, fueled by its focus on a resilient sector of the retail market. Its diverse portfolio and strong balance sheet provide a solid foundation for continued growth, even in a challenging economic environment.
Getty's focus on convenience stores, restaurants, and other essential retail businesses positions it favorably for long-term success. These sectors are less susceptible to the rise of e-commerce and remain crucial to consumer spending. Moreover, Getty's strategy of leasing to strong, well-established operators provides a steady stream of rental income. This is particularly advantageous in times of economic uncertainty, as tenants are more likely to remain financially stable and continue their lease obligations.
The company's commitment to strategic acquisitions and property enhancements sets the stage for future growth. By acquiring high-quality properties in key locations and optimizing their performance, Getty can generate higher rental revenue and expand its footprint. Furthermore, its strong balance sheet provides the financial flexibility to pursue acquisitions, navigate economic cycles, and seize new opportunities as they arise.
Looking ahead, Getty Realty is well-positioned to deliver continued value to its shareholders. Its robust portfolio, strategic focus, and financial strength suggest a promising future for the company. While economic challenges and industry trends may present headwinds, Getty's ability to adapt and capitalize on opportunities makes it a compelling investment option in the real estate sector. Investors seeking a stable and reliable income stream with potential for growth may find Getty Realty a worthy addition to their portfolio.
Rating | Short-Term | Long-Term Senior |
---|---|---|
Outlook | B1 | Ba2 |
Income Statement | C | Caa2 |
Balance Sheet | Caa2 | Baa2 |
Leverage Ratios | B2 | C |
Cash Flow | Baa2 | Baa2 |
Rates of Return and Profitability | Baa2 | Baa2 |
*Financial analysis is the process of evaluating a company's financial performance and position by neural network. It involves reviewing the company's financial statements, including the balance sheet, income statement, and cash flow statement, as well as other financial reports and documents.
How does neural network examine financial reports and understand financial state of the company?
References
- M. Petrik and D. Subramanian. An approximate solution method for large risk-averse Markov decision processes. In Proceedings of the 28th International Conference on Uncertainty in Artificial Intelligence, 2012.
- S. Bhatnagar, H. Prasad, and L. Prashanth. Stochastic recursive algorithms for optimization, volume 434. Springer, 2013
- C. Claus and C. Boutilier. The dynamics of reinforcement learning in cooperative multiagent systems. In Proceedings of the Fifteenth National Conference on Artificial Intelligence and Tenth Innovative Applications of Artificial Intelligence Conference, AAAI 98, IAAI 98, July 26-30, 1998, Madison, Wisconsin, USA., pages 746–752, 1998.
- Keane MP. 2013. Panel data discrete choice models of consumer demand. In The Oxford Handbook of Panel Data, ed. BH Baltagi, pp. 54–102. Oxford, UK: Oxford Univ. Press
- Rumelhart DE, Hinton GE, Williams RJ. 1986. Learning representations by back-propagating errors. Nature 323:533–36
- Gentzkow M, Kelly BT, Taddy M. 2017. Text as data. NBER Work. Pap. 23276
- A. Tamar, D. Di Castro, and S. Mannor. Policy gradients with variance related risk criteria. In Proceedings of the Twenty-Ninth International Conference on Machine Learning, pages 387–396, 2012.