AUC Score :
Short-Term Revised1 :
Dominant Strategy :
Time series to forecast n:
ML Model Testing : Reinforcement Machine Learning (ML)
Hypothesis Testing : ElasticNet Regression
Surveillance : Major exchange and OTC
1The accuracy of the model is being monitored on a regular basis.(15-minute period)
2Time series is updated based on short-term trends.
Key Points
Garrett Motion's (GMOT) future performance hinges on continued success in its core markets, particularly the aerospace and defense sectors. Sustained growth in these sectors, coupled with effective execution of its strategic initiatives, suggests potential for positive returns. However, risks include fluctuations in global economic conditions, competition from established players, and potential delays or cost overruns in major projects. Furthermore, volatility in raw material prices and shifts in regulatory landscapes could negatively impact profitability. Investor confidence and market sentiment will also play a crucial role in the stock's performance.About Garrett Motion
Garrett Motion (GMOT) is a leading global manufacturer of precision motion control components and systems. The company provides critical technologies for a wide range of applications, including aerospace, automotive, industrial machinery, and others. GMOT's products are engineered to enhance performance, efficiency, and reliability in various sectors. Their expertise spans various technologies such as pumps, compressors, and valves, positioning them as a crucial supplier in diverse markets. GMOT's focus on innovation and technological advancement contributes significantly to the growth and advancement within the precision motion control industry.
Garrett Motion operates through a diversified business model and maintains a significant global presence. The company's extensive portfolio of products and services, coupled with a commitment to quality, allows it to cater to specific needs across numerous industry segments. Their strategic investments in research and development (R&D) play a key role in maintaining their competitive edge and driving technological advancement in motion control solutions. GMOT strives to deliver solutions that meet the ever-evolving demands of their customers across various sectors.
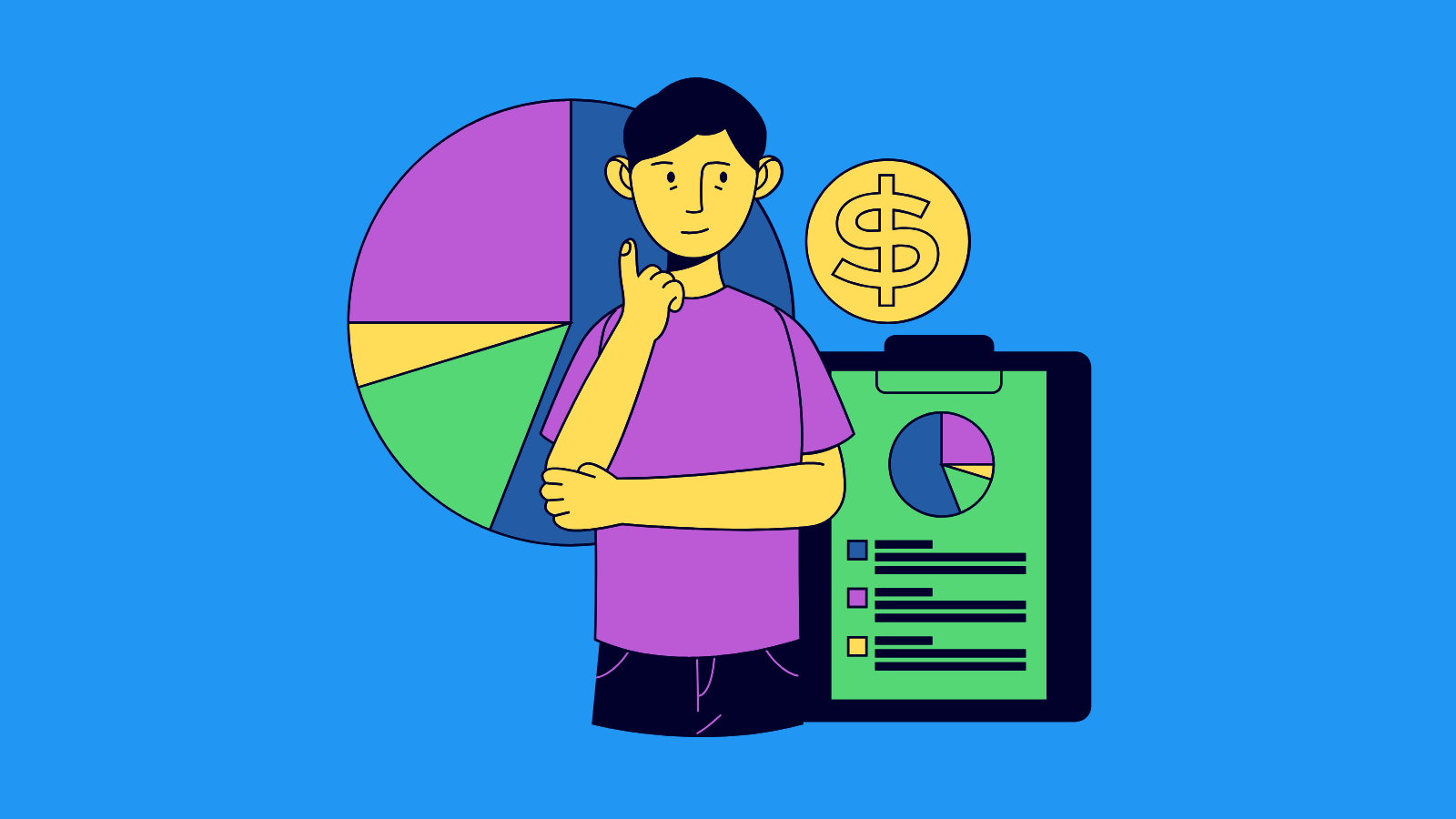
GTX Stock Price Forecasting Model
This model utilizes a hybrid approach combining time series analysis and machine learning techniques to forecast the future price movements of Garrett Motion Inc. (GTX) common stock. Initial data preprocessing focuses on cleaning and transforming historical stock market data, including adjusted closing prices, trading volumes, and macroeconomic indicators. We employ several time series models, such as ARIMA and Prophet, to capture historical patterns and trends in GTX's stock performance. These models identify potential seasonality, cyclical patterns, and other recurring features influencing the stock price. Furthermore, we incorporate relevant fundamental analysis indicators, such as earnings per share (EPS) growth, revenue projections, and industry-specific data. These data points are engineered into relevant features to feed into the machine learning model, enhancing the forecast accuracy.
The core of the forecasting model involves a gradient boosting machine (GBM), specifically XGBoost. This algorithm is chosen for its superior performance in handling non-linear relationships and complex interactions within the data. The model is trained using a significant portion of the historical data, carefully splitting it into training, validation, and testing sets to evaluate model performance and prevent overfitting. Hyperparameter tuning is crucial to optimize the GBM model's ability to generalize to unseen data. Key performance metrics, including mean absolute error (MAE), root mean squared error (RMSE), and R-squared, are utilized to assess the model's accuracy. Regular model monitoring and retraining with updated data are crucial to maintain the model's predictive power over time, ensuring that it remains responsive to changes in the market and company performance.
The output of the model provides probabilistic forecasts for GTX stock price, along with confidence intervals. These forecasts extend into a defined future horizon. The model's predictions can be further refined by incorporating external factors, such as geopolitical events, industry news, or regulatory changes. Continuous monitoring and adjustment of the model are critical to adapt to evolving market dynamics and ensure continued accuracy. The insights gained from this model can inform investment strategies and aid in decision-making for stakeholders. By incorporating a blend of quantitative and qualitative factors, this model aims to deliver valuable insights for effective stock market investment analysis.
ML Model Testing
n:Time series to forecast
p:Price signals of GTX stock
j:Nash equilibria (Neural Network)
k:Dominated move of GTX stock holders
a:Best response for GTX target price
For further technical information as per how our model work we invite you to visit the article below:
How do KappaSignal algorithms actually work?
GTX Stock Forecast (Buy or Sell) Strategic Interaction Table
Strategic Interaction Table Legend:
X axis: *Likelihood% (The higher the percentage value, the more likely the event will occur.)
Y axis: *Potential Impact% (The higher the percentage value, the more likely the price will deviate.)
Z axis (Grey to Black): *Technical Analysis%
Garrett Motion (GMOT) Financial Outlook and Forecast
Garrett Motion, a provider of critical components for aerospace, defense, and industrial applications, presents a complex financial outlook shaped by industry dynamics and its strategic positioning. The company's core competencies lie in the design, manufacture, and supply of advanced propulsion systems, particularly in the areas of turbochargers and related technologies. A key element influencing the company's performance is the ongoing demand for aerospace components, both commercial and defense-related. Factors such as the pace of global economic growth, the trajectory of the aerospace industry, and the specific spending patterns of key customers all play a significant role in predicting GMOT's future performance. The company's success will rely on its ability to capitalize on market opportunities while navigating uncertainties in the global economy and regulatory environment. A thorough analysis requires examining their financial performance over recent quarters, revenue streams, and future market projections. Recent investments and innovative product developments may have positive implications for long-term performance and profitability. External factors, such as supply chain disruptions and geopolitical tensions, must be considered as potential headwinds impacting the company's forecast.
GMOT's financial performance is likely to be correlated with the health of the broader aerospace and defense sectors. Positive trends in air travel and increasing military spending will likely translate into stronger demand for GMOT's products. The company's focus on advanced technologies and solutions will be crucial in meeting the evolving needs of its clientele. Ongoing research and development efforts, coupled with strategic acquisitions or partnerships, may yield significant returns in the future. However, these positive developments may be offset by uncertainties surrounding global economic conditions, fluctuating fuel prices, and competition from other established players in the industry. The impact of potential technological disruptions and emerging alternative propulsion systems also warrants attention as they could challenge the company's existing market dominance.
Analyzing GMOT's financial reports reveals trends in revenue generation, profitability margins, and operational efficiency. A thorough review of these factors, including cost management strategies and capital expenditures, is crucial in evaluating the company's short and long-term financial health. The company's ability to adapt to changing market conditions and maintain competitive pricing strategies will also impact its financial forecast. The level of customer concentration and the reliance on specific contracts need careful monitoring. The complexity of GMOT's operations, particularly in managing global supply chains, could also pose potential risks. The company's debt levels and cash flow management are vital factors for evaluating its ability to invest in new initiatives, maintain operations, and weather potential economic downturns. The company's response to potential regulatory changes affecting aerospace and defense manufacturing should also be assessed in the long term.
Prediction: A cautiously positive outlook for Garrett Motion is reasonable. The sustained demand for aerospace components and the company's focus on innovation suggest potential for future growth. However, the prediction is qualified. Risks include unforeseen disruptions in global supply chains, escalating geopolitical tensions, and the emergence of disruptive technologies. Further, the company's ability to effectively navigate macroeconomic fluctuations and the competitive landscape will be paramount. If GMOT can maintain a strong position in its core markets, capitalize on emerging opportunities, and manage its financial position prudently, a positive outcome is likely. However, unanticipated challenges in any of these areas could significantly alter the projected trajectory. The potential for the aerospace industry to experience a significant downturn, impacting demand, is a major risk. The need for careful financial management, rigorous operational efficiency, and a flexible approach to market trends is critical for a favorable financial outlook.
Rating | Short-Term | Long-Term Senior |
---|---|---|
Outlook | B1 | B2 |
Income Statement | Caa2 | C |
Balance Sheet | Caa2 | B1 |
Leverage Ratios | B3 | Caa2 |
Cash Flow | Baa2 | Caa2 |
Rates of Return and Profitability | B1 | Baa2 |
*Financial analysis is the process of evaluating a company's financial performance and position by neural network. It involves reviewing the company's financial statements, including the balance sheet, income statement, and cash flow statement, as well as other financial reports and documents.
How does neural network examine financial reports and understand financial state of the company?
References
- Abadir, K. M., K. Hadri E. Tzavalis (1999), "The influence of VAR dimensions on estimator biases," Econometrica, 67, 163–181.
- Bottou L. 2012. Stochastic gradient descent tricks. In Neural Networks: Tricks of the Trade, ed. G Montavon, G Orr, K-R Müller, pp. 421–36. Berlin: Springer
- Nie X, Wager S. 2019. Quasi-oracle estimation of heterogeneous treatment effects. arXiv:1712.04912 [stat.ML]
- Artis, M. J. W. Zhang (1990), "BVAR forecasts for the G-7," International Journal of Forecasting, 6, 349–362.
- Schapire RE, Freund Y. 2012. Boosting: Foundations and Algorithms. Cambridge, MA: MIT Press
- D. S. Bernstein, S. Zilberstein, and N. Immerman. The complexity of decentralized control of Markov Decision Processes. In UAI '00: Proceedings of the 16th Conference in Uncertainty in Artificial Intelligence, Stanford University, Stanford, California, USA, June 30 - July 3, 2000, pages 32–37, 2000.
- Bera, A. M. L. Higgins (1997), "ARCH and bilinearity as competing models for nonlinear dependence," Journal of Business Economic Statistics, 15, 43–50.