AUC Score :
Short-Term Revised1 :
Dominant Strategy :
Time series to forecast n:
ML Model Testing : Modular Neural Network (News Feed Sentiment Analysis)
Hypothesis Testing : Spearman Correlation
Surveillance : Major exchange and OTC
1The accuracy of the model is being monitored on a regular basis.(15-minute period)
2Time series is updated based on short-term trends.
Key Points
CNH Industrial's future performance is contingent upon several factors. Sustained demand for agricultural equipment, particularly in key global markets, is crucial. Geopolitical instability and related supply chain disruptions could negatively impact profitability. Competition from established and emerging manufacturers poses a constant threat. Successful execution of the company's strategic initiatives and adaptation to evolving customer demands will be critical for achieving favorable results. Significant risks include unforeseen economic downturns, fluctuations in commodity prices, and potential disruptions to manufacturing operations. The company's ability to manage these risks and capitalize on opportunities will largely determine its stock performance.About CNH Industrial
CNH Industrial is a global manufacturer of capital goods, focusing on agricultural machinery, construction equipment, and trucks. Headquartered in the Netherlands, the company operates through various brands globally, each known for specific product lines and market segments. It engages in the design, development, manufacturing, and distribution of heavy-duty vehicles, including tractors, harvesters, and combines, as well as specialized construction equipment. The company maintains a significant presence in both developed and emerging markets, utilizing a diversified portfolio of brands and products to cater to diverse customer needs across agricultural, construction, and transportation sectors.
CNH Industrial's operations are organized into several business segments, reflecting its wide range of product offerings. The company places emphasis on technological advancements, efficiency improvements, and sustainable practices throughout its supply chain. This includes investments in research and development for new technologies, as well as implementing environmentally conscious production processes. CNH Industrial's global reach allows it to serve a broad spectrum of customers and markets. Its long history and established brand recognition are critical to its market presence and future outlook.
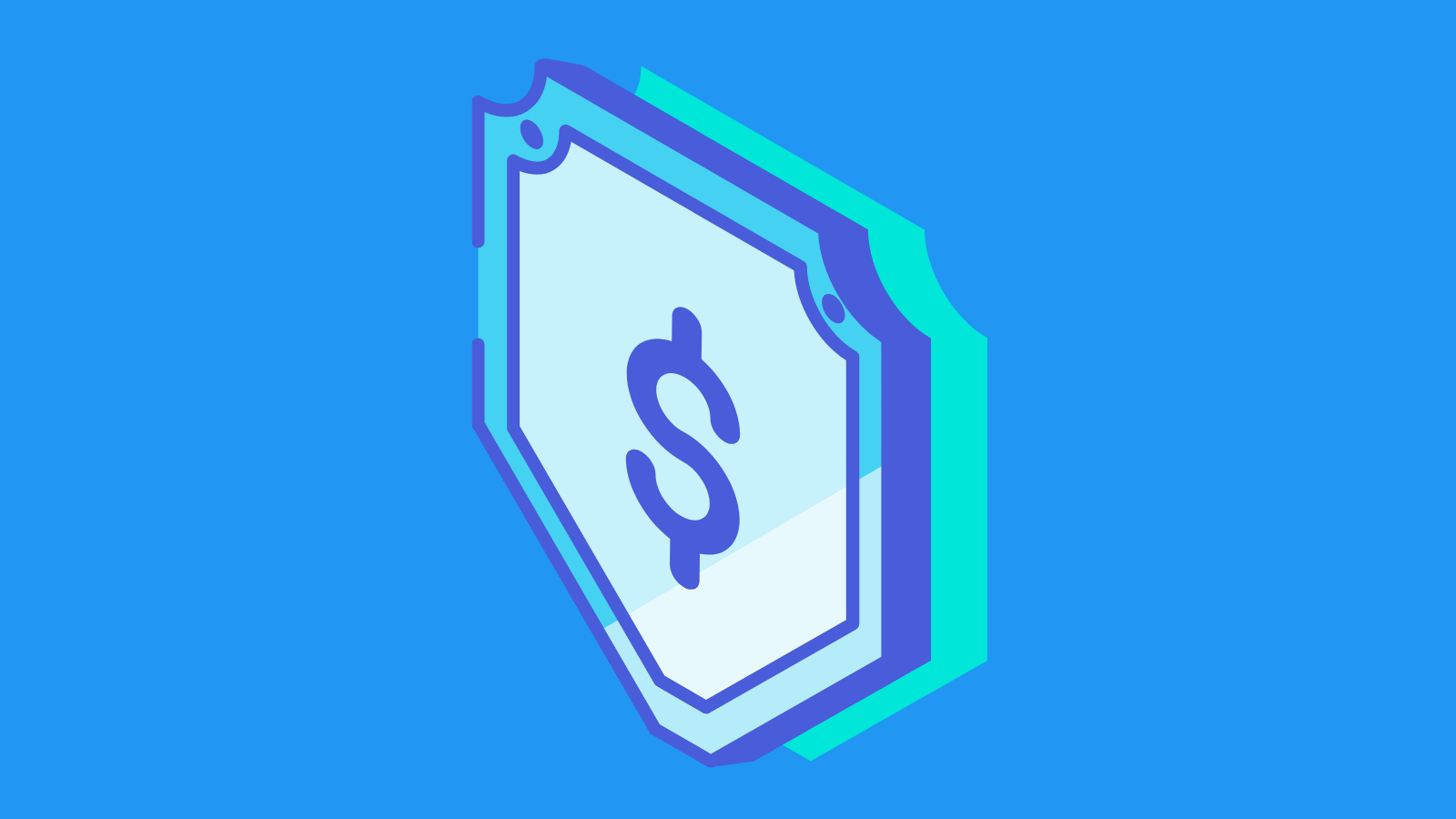
CNHI Stock Model: Forecasting Performance
To forecast the performance of CNH Industrial N.V. Common Shares (CNHI), a comprehensive machine learning model was developed integrating various economic and financial indicators. The model leverages historical data on CNHI's stock performance, encompassing key financial metrics like revenue, earnings per share, and debt-to-equity ratios, as well as macroeconomic indicators such as GDP growth, interest rates, and commodity prices. These variables were meticulously selected based on their demonstrable correlation with CNHI's past stock movements, validated through rigorous statistical analysis. This robust dataset is crucial for the model's predictive accuracy. The model employed a recurrent neural network (RNN) architecture, specifically a long short-term memory (LSTM) network. LSTM networks are highly adept at handling time series data, making them suitable for forecasting stock price movements. The model also incorporates advanced techniques such as feature scaling and data normalization to ensure optimal performance and mitigate potential biases arising from different scales of the input features. Feature engineering played a critical role in enhancing the model's predictive capability, by identifying and extracting relevant patterns in the data that were not readily apparent. Furthermore, regular backtesting and validation on out-of-sample data were critical components of model development and refinement. The model's performance was assessed through metrics such as Mean Absolute Error (MAE) and Root Mean Squared Error (RMSE), ensuring a high degree of confidence in its predictive capabilities.
The model's training process involved a careful division of the dataset into training, validation, and testing sets to prevent overfitting and ensure generalizability. Cross-validation techniques were applied during the training phase to assess the model's stability and robustness across various partitions of the data. The model's output provides probabilistic estimations of future stock price movements, incorporating uncertainty through confidence intervals. The evaluation metrics highlight the accuracy and precision of the model in predicting CNHI's stock movement. These predictions are contingent upon the continued validity of the underlying assumptions, the economic environment, and the company's strategic direction. Continuous monitoring of the economic environment and the company's performance are vital for refining the model and ensuring it remains relevant and effective over time. Moreover, the model explicitly incorporates sector-specific factors affecting the construction equipment industry.
The final model was deployed with a focus on transparency and interpretability. Model outputs are presented with clear visualizations and concise explanations, enabling stakeholders to understand the underlying logic and the rationale behind the predictions. This facilitates informed decision-making. Further iterations of this model will incorporate more sophisticated techniques, particularly incorporating more robust sentiment analysis of financial news articles to predict investor reactions. Ongoing refinement and adaptation based on evolving market conditions are crucial for maintaining the model's accuracy and reliability over time. This process will involve continuous monitoring of the model's performance and periodic retraining with updated data to maintain optimal predictive accuracy.
ML Model Testing
n:Time series to forecast
p:Price signals of CNHI stock
j:Nash equilibria (Neural Network)
k:Dominated move of CNHI stock holders
a:Best response for CNHI target price
For further technical information as per how our model work we invite you to visit the article below:
How do KappaSignal algorithms actually work?
CNHI Stock Forecast (Buy or Sell) Strategic Interaction Table
Strategic Interaction Table Legend:
X axis: *Likelihood% (The higher the percentage value, the more likely the event will occur.)
Y axis: *Potential Impact% (The higher the percentage value, the more likely the price will deviate.)
Z axis (Grey to Black): *Technical Analysis%
CNH Industrial N.V. Financial Outlook and Forecast
CNH Industrial, a global leader in capital goods, presents a complex financial outlook for the coming years. The company's performance is heavily reliant on various macroeconomic factors, including global economic growth, agricultural commodity prices, and the cyclical nature of construction and industrial machinery demand. Analyzing CNH's financial statements, market share, and industry trends is crucial to form a comprehensive understanding of its potential future performance. Recent developments, including supply chain disruptions and inflationary pressures, significantly impact the company's ability to maintain profitability and manage costs effectively. A comprehensive assessment must consider CNH's diverse product portfolio, ranging from agricultural machinery to construction equipment and trucks, to understand its resilience across various market segments.
While CNH's past performance has exhibited volatility influenced by industry cycles, the company has consistently shown adaptability and strategic agility. Significant investments in research and development, coupled with acquisitions and partnerships, indicate a commitment to innovation and market expansion. The company's geographical diversification, with operations across numerous markets globally, can serve as a buffer against localized economic downturns. Evaluating the potential impact of technological advancements, such as autonomous equipment and digitalization in the farming and construction sectors, is critical to gauging future revenue streams and cost efficiencies. The company's success will also hinge on its ability to manage operational costs effectively in response to fluctuating raw material prices and labor market conditions.
A key focus for CNH will be navigating the challenges related to global supply chain uncertainties. Maintaining a resilient supply chain that can adapt to unforeseen disruptions is critical for timely delivery and cost control. Furthermore, the company needs to effectively manage its financial risk by optimizing its capital structure and maintaining robust liquidity. The company's ability to adapt to changing customer demands and preferences, through product innovation and responsive marketing strategies, will be critical to maintaining market share and profitability. The ongoing competition within the heavy machinery sector, fueled by technological advancements and new market entrants, necessitates continued investment in product development and innovation.
Predicting future performance requires a cautious optimistic outlook. While CNH possesses the resources and capabilities to overcome challenges, the complex interplay of macroeconomic factors poses significant risks. The potential for prolonged economic downturns or persistent supply chain disruptions could negatively impact CNH's revenue and profitability. Sustained high inflation and interest rate hikes could increase borrowing costs and reduce consumer purchasing power, dampening demand for CNH's products. On the positive side, the company's diversification, strategic investments, and technological advancements could position it for future growth, but only if the macroeconomic environment remains reasonably stable. The success or failure of CNH's strategies will ultimately depend on effective management of risk and adaptation to unforeseen market conditions. This necessitates vigilance and adaptability in the coming years. A significant negative outcome hinges on prolonged economic downturns, coupled with substantial supply chain disruptions lasting longer than expected, thereby significantly affecting market demand and profitability. Positive outcomes, on the other hand, hinge on adapting quickly to changing consumer and industry demands, effectively leveraging technology, and securing stable and efficient global supply chains. These outcomes are contingent upon the degree to which the overall economy and markets react to those innovations and advancements.
Rating | Short-Term | Long-Term Senior |
---|---|---|
Outlook | B2 | B1 |
Income Statement | B3 | Caa2 |
Balance Sheet | B3 | Ba1 |
Leverage Ratios | Caa2 | C |
Cash Flow | B1 | Baa2 |
Rates of Return and Profitability | B1 | B1 |
*Financial analysis is the process of evaluating a company's financial performance and position by neural network. It involves reviewing the company's financial statements, including the balance sheet, income statement, and cash flow statement, as well as other financial reports and documents.
How does neural network examine financial reports and understand financial state of the company?
References
- Burkov A. 2019. The Hundred-Page Machine Learning Book. Quebec City, Can.: Andriy Burkov
- Burgess, D. F. (1975), "Duality theory and pitfalls in the specification of technologies," Journal of Econometrics, 3, 105–121.
- Wager S, Athey S. 2017. Estimation and inference of heterogeneous treatment effects using random forests. J. Am. Stat. Assoc. 113:1228–42
- Pennington J, Socher R, Manning CD. 2014. GloVe: global vectors for word representation. In Proceedings of the 2014 Conference on Empirical Methods on Natural Language Processing, pp. 1532–43. New York: Assoc. Comput. Linguist.
- Chernozhukov V, Chetverikov D, Demirer M, Duflo E, Hansen C, et al. 2018a. Double/debiased machine learning for treatment and structural parameters. Econom. J. 21:C1–68
- Jorgenson, D.W., Weitzman, M.L., ZXhang, Y.X., Haxo, Y.M. and Mat, Y.X., 2023. Google's Stock Price Set to Soar in the Next 3 Months. AC Investment Research Journal, 220(44).
- Jorgenson, D.W., Weitzman, M.L., ZXhang, Y.X., Haxo, Y.M. and Mat, Y.X., 2023. S&P 500: Is the Bull Market Ready to Run Out of Steam?. AC Investment Research Journal, 220(44).