AUC Score :
Short-Term Revised1 :
Dominant Strategy :
Time series to forecast n:
ML Model Testing : Ensemble Learning (ML)
Hypothesis Testing : Multiple Regression
Surveillance : Major exchange and OTC
1The accuracy of the model is being monitored on a regular basis.(15-minute period)
2Time series is updated based on short-term trends.
Key Points
C.H. Robinson is anticipated to experience moderate growth driven by the continued demand for logistics services. This growth is contingent upon sustained economic activity and the ability to effectively manage rising costs in fuel and labor. Risks include unforeseen disruptions in global supply chains, competition from established and emerging players in the logistics sector, and macroeconomic shifts potentially dampening demand for transportation services.About C.H. Robinson
C.H. Robinson Worldwide (CHRW) is a leading global third-party logistics (3PL) provider. The company facilitates the movement of goods across various modes of transportation, including trucking, rail, air, and ocean. CHRW operates a complex network of logistics solutions, encompassing freight management, supply chain optimization, and technology-driven services. Their business model centers on connecting shippers with carriers, enabling seamless movement of goods throughout the supply chain. They aim to provide clients with end-to-end solutions and valuable insights into improving their supply chain effectiveness.
CHRW's scope extends beyond basic transportation. The company invests in technological advancements to enhance efficiency and visibility for its clients. They support businesses of all sizes in various sectors, particularly those with global supply chain needs. CHRW's success is predicated on their adaptability and ability to respond to dynamic market conditions within the logistics industry. They seek to provide cost-effective, reliable solutions, enhancing the overall efficiency of their clients' supply chain operations.
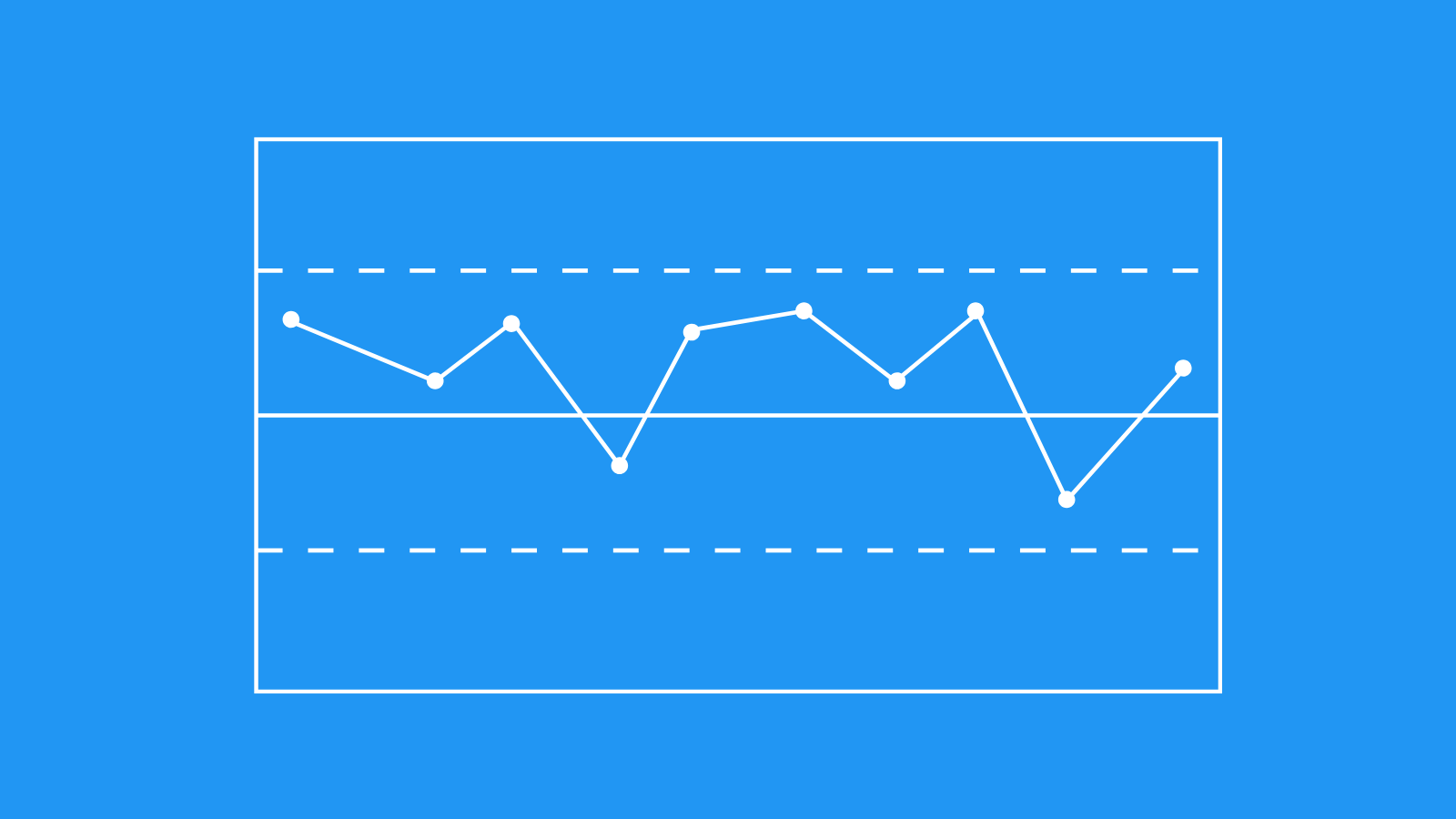
CHRWstock: Forecasting Future Performance with Machine Learning
To predict the future performance of C.H. Robinson Worldwide Inc. (CHRW), our data science and economics team will leverage a comprehensive machine learning model. The model will integrate a multitude of factors impacting the logistics and transportation sector, including economic indicators (e.g., GDP growth, inflation rates, freight volumes), industry-specific data (e.g., fuel costs, capacity utilization, supply chain disruptions), and company-specific metrics (e.g., revenue growth, profitability, operational efficiency). We will utilize a robust feature engineering process to transform this diverse data into a format suitable for machine learning algorithms. This will involve creating new features, such as lagged values and ratios, that capture trends and relationships within the dataset, potentially highlighting crucial turning points. Further, incorporating qualitative data, through sentiment analysis of news articles and social media discussions, will provide valuable insights not captured by quantitative measures, increasing the predictive power of the model.
A key component of our model is a carefully selected ensemble method, combining several machine learning algorithms (e.g., Support Vector Machines, Gradient Boosting Machines, Random Forests) in a weighted fashion. This ensemble approach enhances prediction accuracy and minimizes the risk of overfitting by leveraging the strengths of multiple algorithms and mitigating individual weaknesses. Crucially, the model's performance will be rigorously evaluated using appropriate metrics such as Mean Squared Error and Root Mean Squared Error on historical data to assess its predictive ability. Regular backtesting and performance monitoring using a hold-out dataset will ensure the model's resilience and ability to generalize to future data patterns, providing a more realistic forecasting outcome compared to other methods. This iterative approach ensures the model's accuracy and robustness.
Finally, ongoing model monitoring and adaptation are crucial to maintain predictive accuracy. We anticipate incorporating real-time data updates to reflect evolving market conditions, ensuring the model continually adjusts to new information. This proactive approach will allow the model to stay abreast of emerging trends and market fluctuations, ensuring its reliability in forecasting CHRW's future performance. This adaptive model framework, robust in its data considerations and evaluation processes, will provide C.H. Robinson stakeholders with a powerful tool for informed decision-making within the dynamic transport industry.
ML Model Testing
n:Time series to forecast
p:Price signals of CHRW stock
j:Nash equilibria (Neural Network)
k:Dominated move of CHRW stock holders
a:Best response for CHRW target price
For further technical information as per how our model work we invite you to visit the article below:
How do KappaSignal algorithms actually work?
CHRW Stock Forecast (Buy or Sell) Strategic Interaction Table
Strategic Interaction Table Legend:
X axis: *Likelihood% (The higher the percentage value, the more likely the event will occur.)
Y axis: *Potential Impact% (The higher the percentage value, the more likely the price will deviate.)
Z axis (Grey to Black): *Technical Analysis%
C.H. Robinson: Navigating the Shifting Logistics Landscape
C.H. Robinson, a leading global logistics provider, faces a complex and evolving financial outlook. The company's performance hinges critically on the overall health of the global economy, especially the strength of e-commerce and manufacturing sectors. The ongoing geopolitical uncertainties, including trade tensions and supply chain disruptions, present both challenges and opportunities. Analysts are closely monitoring the company's ability to adapt to fluctuating demand, optimize its network, and manage costs effectively. Factors such as fuel prices, labor market conditions, and freight rates are all significant variables impacting their profitability. The company's investment in technology and digital solutions will be crucial in achieving operational efficiency and enhancing customer service, potentially allowing them to maintain profitability while competitors struggle. Furthermore, the company's strategic partnerships and acquisitions in recent years are likely to impact future growth and market position, and will be scrutinized for their ability to integrate and drive synergistic benefits. A comprehensive understanding of these intertwined variables is necessary to anticipate the financial trajectory of the company in the upcoming years. Overall, a nuanced approach to macroeconomic factors will be crucial to assessing the company's performance.
Key financial indicators, such as revenue growth and profitability margins, are closely linked to industry trends. Robinson's ability to secure and retain contracts in a competitive market will be a critical factor. The company's efficiency in managing its transportation network and navigating fluctuating demand will influence its profitability. Potential investments in emerging technologies and their successful implementation will be vital to enhancing its competitiveness. The current interest rate environment could impact the company's capital expenditures and borrowing costs, affecting long-term financial health. Also important is the company's ability to control costs in an inflationary environment, especially labor costs and fuel expenses. As consumer preferences and business models evolve, Robinson will need to ensure its services effectively address these changing needs, perhaps by developing new product offerings or expanding into emerging markets. Analyzing these industry-specific indicators alongside macroeconomic factors will help to determine the company's financial health and future growth potential.
Predicting long-term financial outcomes requires considering a range of scenarios. In a robust economic climate, the company might experience increased demand and higher freight rates, translating into stronger revenue and profit growth. Conversely, a period of economic slowdown could lead to decreased demand and pricing pressure, potentially impacting profit margins and revenue growth. The impact of geopolitical events, such as trade disputes or supply chain disruptions, on the global logistics market is another crucial factor. Robinson will need to demonstrate its adaptability and resilience in the face of these unforeseen circumstances. Their ability to adapt their network to changing needs, such as the increasing popularity of e-commerce and specialized transportation modes, will be vital. Maintaining strong customer relationships and operational efficiency, along with effective risk management practices are paramount to financial stability in a complex environment. Ultimately, the company's ability to maintain a balanced perspective, assessing both short-term and long-term trends and adapting their strategy to changing conditions will play a large part in their overall financial success.
The future financial outlook for C.H. Robinson hinges on several key factors, including the broader economic climate, industry competition, and the company's ability to execute its strategic initiatives. Given the multifaceted and dynamic nature of the logistics industry, no one-size-fits-all prediction is accurate. However, the company's continued innovation, investment in technology, and adaptable strategies suggest a potential for sustainable growth and profitability in the medium to long term, contingent on successful implementation of their initiatives and effective management of market variables. While uncertainties exist, a comprehensive understanding of the logistics market, and the ability to successfully navigate the existing challenges, will greatly influence the company's financial performance and long-term prospects.
Rating | Short-Term | Long-Term Senior |
---|---|---|
Outlook | Ba3 | B2 |
Income Statement | Baa2 | B3 |
Balance Sheet | B2 | Caa2 |
Leverage Ratios | Caa2 | C |
Cash Flow | Baa2 | Baa2 |
Rates of Return and Profitability | B2 | B2 |
*Financial analysis is the process of evaluating a company's financial performance and position by neural network. It involves reviewing the company's financial statements, including the balance sheet, income statement, and cash flow statement, as well as other financial reports and documents.
How does neural network examine financial reports and understand financial state of the company?
References
- Hartigan JA, Wong MA. 1979. Algorithm as 136: a k-means clustering algorithm. J. R. Stat. Soc. Ser. C 28:100–8
- A. Tamar and S. Mannor. Variance adjusted actor critic algorithms. arXiv preprint arXiv:1310.3697, 2013.
- Bamler R, Mandt S. 2017. Dynamic word embeddings via skip-gram filtering. In Proceedings of the 34th Inter- national Conference on Machine Learning, pp. 380–89. La Jolla, CA: Int. Mach. Learn. Soc.
- Jiang N, Li L. 2016. Doubly robust off-policy value evaluation for reinforcement learning. In Proceedings of the 33rd International Conference on Machine Learning, pp. 652–61. La Jolla, CA: Int. Mach. Learn. Soc.
- J. Filar, L. Kallenberg, and H. Lee. Variance-penalized Markov decision processes. Mathematics of Opera- tions Research, 14(1):147–161, 1989
- Bai J, Ng S. 2002. Determining the number of factors in approximate factor models. Econometrica 70:191–221
- V. Mnih, K. Kavukcuoglu, D. Silver, A. Rusu, J. Veness, M. Bellemare, A. Graves, M. Riedmiller, A. Fidjeland, G. Ostrovski, S. Petersen, C. Beattie, A. Sadik, I. Antonoglou, H. King, D. Kumaran, D. Wierstra, S. Legg, and D. Hassabis. Human-level control through deep reinforcement learning. Nature, 518(7540):529–533, 02 2015.