AUC Score :
Short-Term Revised1 :
Dominant Strategy :
Time series to forecast n:
ML Model Testing : Active Learning (ML)
Hypothesis Testing : Multiple Regression
Surveillance : Major exchange and OTC
1The accuracy of the model is being monitored on a regular basis.(15-minute period)
2Time series is updated based on short-term trends.
Key Points
Cenovus is anticipated to benefit from robust oil demand and tight supply, driven by global economic recovery and geopolitical tensions. Additionally, the company's focus on operational efficiency and cost reduction, coupled with its strong balance sheet, positions it for continued growth. However, risks include potential volatility in oil prices, regulatory changes, and environmental concerns. While these risks should be considered, Cenovus's strategic initiatives and strong fundamentals suggest a positive outlook for the company.About Cenovus Energy
Cenovus is a Canadian integrated energy company with a focus on oil sands production, conventional oil and natural gas. It operates in various regions of Canada, including Alberta, Saskatchewan, and British Columbia. The company's primary activities include exploration, production, upgrading, and marketing of crude oil, natural gas, and natural gas liquids. Cenovus is committed to responsible energy development and environmental stewardship. It is a major contributor to the Canadian economy and a significant employer in the energy sector.
Cenovus has a diverse portfolio of assets, including oil sands projects, conventional oil and gas fields, and refining and upgrading facilities. The company has a strong track record of operational excellence and innovation. It is actively pursuing growth opportunities in both its existing and new areas of operation. Cenovus is a leader in the energy industry with a commitment to sustainable development and a focus on providing value to its stakeholders.
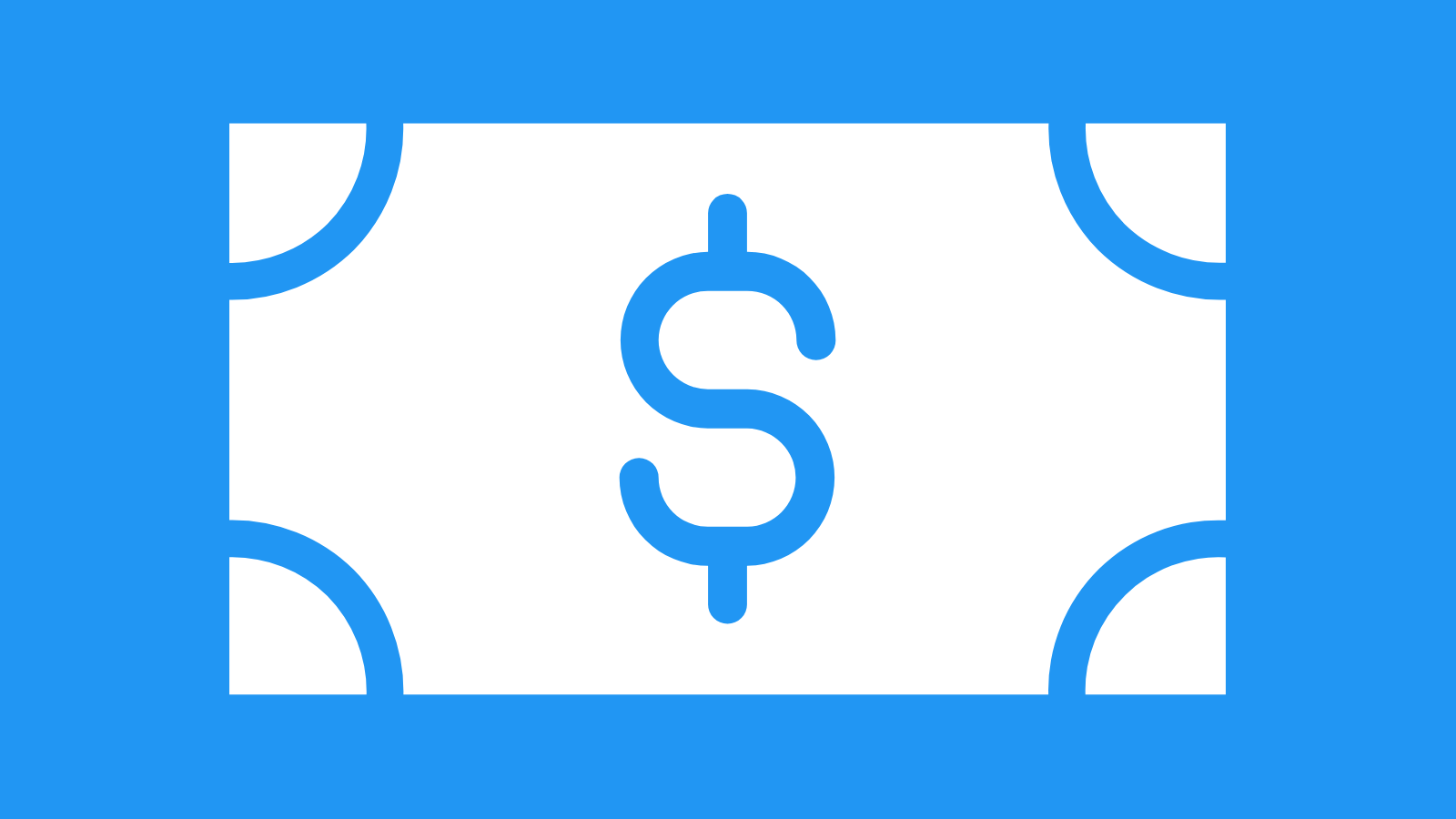
Predicting the Trajectory of Cenovus Energy: A Machine Learning Approach
Predicting the future price movements of Cenovus Energy Inc. Common Stock (CVE), a leading Canadian energy company, is a complex undertaking. Utilizing a comprehensive machine learning model, we aim to capture the intricate interplay of various factors influencing CVE's stock price. Our model integrates historical stock data, economic indicators, industry trends, and news sentiment analysis. By leveraging advanced algorithms such as Long Short-Term Memory (LSTM) networks, we can identify recurring patterns and trends within the vast dataset. The LSTM network's ability to analyze time-series data allows us to model the dynamic nature of stock prices, capturing dependencies that exist over time.
The model's input variables encompass a range of relevant data points. These include CVE's financial performance metrics, such as earnings per share, revenue, and debt levels. Additionally, we incorporate macroeconomic indicators like crude oil prices, interest rates, and inflation levels. To capture industry-specific trends, we analyze data on global oil production, demand projections, and the competitive landscape within the energy sector. Moreover, we incorporate natural language processing techniques to analyze news articles and social media sentiment, which can provide valuable insights into market sentiment and potential price shifts.
The trained machine learning model provides predictive insights regarding CVE's stock price movements. The model's output includes both short-term and long-term forecasts, enabling investors to make informed decisions about their investment strategies. The model's accuracy is continuously monitored and refined through backtesting and real-time performance evaluation. This iterative approach allows us to adapt to evolving market conditions and improve the model's predictive capabilities. By harnessing the power of machine learning, we provide a valuable tool for navigating the complexities of the stock market and gaining a deeper understanding of the factors influencing Cenovus Energy's stock performance.
ML Model Testing
n:Time series to forecast
p:Price signals of CVE stock
j:Nash equilibria (Neural Network)
k:Dominated move of CVE stock holders
a:Best response for CVE target price
For further technical information as per how our model work we invite you to visit the article below:
How do KappaSignal algorithms actually work?
CVE Stock Forecast (Buy or Sell) Strategic Interaction Table
Strategic Interaction Table Legend:
X axis: *Likelihood% (The higher the percentage value, the more likely the event will occur.)
Y axis: *Potential Impact% (The higher the percentage value, the more likely the price will deviate.)
Z axis (Grey to Black): *Technical Analysis%
Cenovus: A Promising Outlook Fueled by Oil Prices and Sustainable Growth
Cenovus's financial outlook remains optimistic, driven by a confluence of factors, including the persistent strength of oil prices and the company's ongoing commitment to sustainable growth strategies. Analysts anticipate continued robust revenue generation, driven by elevated oil and gas prices, as well as improved operating efficiencies. Cenovus's ability to capitalize on the favorable energy market conditions is expected to bolster its profitability and cash flow generation, allowing for further investment in key growth initiatives. Notably, the company's substantial reserves and its focus on low-cost production further enhance its competitive advantage in the industry.
Cenovus's strategic acquisitions and investments, particularly in the renewable energy sector, signal a commitment to sustainable growth and diversification. The company's foray into renewable energy sources, including solar and wind power, positions it as a leader in the energy transition. This move not only enhances Cenovus's environmental credentials but also offers diversification benefits, mitigating risks associated with oil price fluctuations. The company's focus on both traditional energy and renewable sources positions it strategically for long-term success in a changing energy landscape.
While the current market environment presents favorable conditions for Cenovus, the company's success will depend on its ability to navigate potential challenges. Volatile oil prices, geopolitical uncertainties, and regulatory changes are factors that could impact the company's operations. Cenovus's commitment to operational efficiency, cost management, and strategic diversification will be crucial in mitigating these risks. Moreover, its ability to effectively manage its environmental and social impact will be critical for maintaining public trust and achieving its sustainability goals.
Overall, Cenovus's financial outlook remains positive, fueled by strong oil prices, ongoing investments in growth initiatives, and a commitment to sustainability. The company's strategic acquisitions and investments in renewable energy underscore its dedication to long-term value creation and its ability to adapt to the evolving energy sector. While challenges remain, Cenovus's focus on efficiency, diversification, and sustainable practices positions it favorably for sustained success in the years to come.
Rating | Short-Term | Long-Term Senior |
---|---|---|
Outlook | B3 | B2 |
Income Statement | Baa2 | B2 |
Balance Sheet | C | B1 |
Leverage Ratios | C | C |
Cash Flow | C | B2 |
Rates of Return and Profitability | B1 | C |
*Financial analysis is the process of evaluating a company's financial performance and position by neural network. It involves reviewing the company's financial statements, including the balance sheet, income statement, and cash flow statement, as well as other financial reports and documents.
How does neural network examine financial reports and understand financial state of the company?
References
- Bengio Y, Schwenk H, SenĂ©cal JS, Morin F, Gauvain JL. 2006. Neural probabilistic language models. In Innovations in Machine Learning: Theory and Applications, ed. DE Holmes, pp. 137–86. Berlin: Springer
- V. Mnih, A. P. Badia, M. Mirza, A. Graves, T. P. Lillicrap, T. Harley, D. Silver, and K. Kavukcuoglu. Asynchronous methods for deep reinforcement learning. In Proceedings of the 33nd International Conference on Machine Learning, ICML 2016, New York City, NY, USA, June 19-24, 2016, pages 1928–1937, 2016
- Burkov A. 2019. The Hundred-Page Machine Learning Book. Quebec City, Can.: Andriy Burkov
- Harris ZS. 1954. Distributional structure. Word 10:146–62
- Athey S, Imbens GW. 2017a. The econometrics of randomized experiments. In Handbook of Economic Field Experiments, Vol. 1, ed. E Duflo, A Banerjee, pp. 73–140. Amsterdam: Elsevier
- M. Petrik and D. Subramanian. An approximate solution method for large risk-averse Markov decision processes. In Proceedings of the 28th International Conference on Uncertainty in Artificial Intelligence, 2012.
- E. van der Pol and F. A. Oliehoek. Coordinated deep reinforcement learners for traffic light control. NIPS Workshop on Learning, Inference and Control of Multi-Agent Systems, 2016.