AUC Score :
Short-Term Revised1 :
Dominant Strategy :
Time series to forecast n:
ML Model Testing : Ensemble Learning (ML)
Hypothesis Testing : Stepwise Regression
Surveillance : Major exchange and OTC
1The accuracy of the model is being monitored on a regular basis.(15-minute period)
2Time series is updated based on short-term trends.
Key Points
The CAC 40 index is anticipated to experience moderate fluctuations in the coming period. Economic headwinds, such as persistent inflation and rising interest rates, are likely to exert downward pressure on the index. However, potential positive catalysts, including advancements in specific sectors like technology or a rebound in consumer confidence, could create opportunities for upward movement. Geopolitical uncertainties and unforeseen global events remain a significant risk factor. Investors should prepare for volatility and consider diversifying their portfolios. The precise trajectory will depend on the interplay of these factors and their respective strength.About CAC 40 Index
The CAC 40 is a stock market index that tracks the performance of the 40 largest publicly listed companies in France. It represents a significant portion of the French stock market and is widely considered a key indicator of the overall health and performance of the French economy. The index provides a benchmark for investors to measure their returns against when investing in French equities. Its composition is reviewed periodically to ensure the companies represented remain highly relevant to the current market landscape.
The CAC 40's importance extends beyond France, as it is a well-known and actively traded index globally. It influences investment decisions from both domestic and international investors, reflecting the confidence or concerns about the French economy. It serves as a crucial tool for portfolio diversification and a gauge of the market trends within the European stock market. Fluctuations in the CAC 40 index can significantly impact the broader French market and other related global markets.
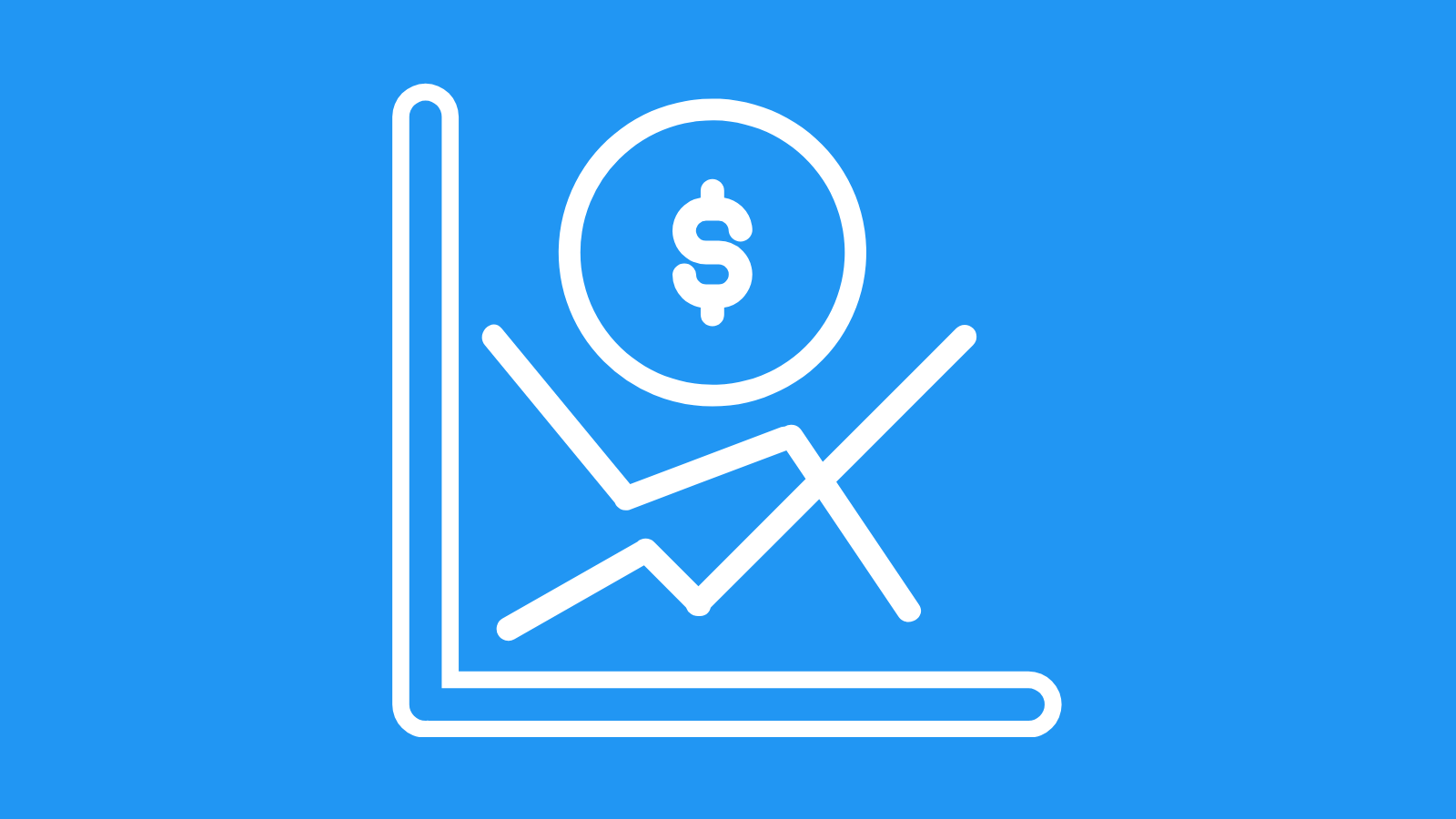
CAC 40 Index Forecasting Model
This model leverages a multi-layered approach to forecasting the CAC 40 index. We begin by assembling a comprehensive dataset encompassing various economic indicators, such as inflation rates, GDP growth, interest rates, and unemployment figures, alongside crucial financial data like company earnings reports, trading volume, and market sentiment indices. Data preprocessing is paramount; this involves cleaning, transforming, and feature engineering to ensure data quality and compatibility with the machine learning algorithms. This includes handling missing values, scaling features to similar ranges, and creating engineered features that capture non-linear relationships within the data. Time series decomposition will be used to separate trend, seasonality, and noise components from the historical data, allowing for a more accurate representation of underlying patterns.
A sophisticated ensemble learning model, combining Gradient Boosting Machines (GBM) and Recurrent Neural Networks (RNNs), will form the core of our predictive framework. GBM excels at capturing complex relationships within the data, while RNNs are particularly well-suited for handling sequential data and identifying temporal patterns within the economic indicators. Hyperparameter tuning will be crucial for optimal model performance, ensuring that both the GBM and RNN components are adequately configured to capture subtle nuances and long-term trends. Crucially, we will incorporate a rolling window approach for model training. This allows us to update the model incrementally with new data, maintaining its adaptability to dynamic market conditions. Regular model evaluation will occur using metrics such as Mean Absolute Error (MAE) and Root Mean Squared Error (RMSE), rigorously assessing the model's predictive accuracy and stability. This ongoing evaluation is critical to ensuring the model's ability to adjust to changing market dynamics.
Finally, a crucial component of the model involves rigorous backtesting and validation. We will utilize a substantial portion of historical data to evaluate the model's performance, and split it into training, validation, and testing sets. This comprehensive approach aims to ensure the model's reliability and generalizability to future data. Robust risk management strategies will be developed to mitigate potential forecasting errors. The model will output both point forecasts and probabilistic forecasts, providing a range of possible outcomes rather than a single prediction. This empowers users with greater insight into the potential uncertainty inherent in financial markets, enabling them to make more informed investment decisions. We anticipate that this approach will generate a high-accuracy model for CAC 40 Index prediction, while also providing an understanding of the underlying forces driving market fluctuations.
ML Model Testing
n:Time series to forecast
p:Price signals of CAC 40 index
j:Nash equilibria (Neural Network)
k:Dominated move of CAC 40 index holders
a:Best response for CAC 40 target price
For further technical information as per how our model work we invite you to visit the article below:
How do KappaSignal algorithms actually work?
CAC 40 Index Forecast Strategic Interaction Table
Strategic Interaction Table Legend:
X axis: *Likelihood% (The higher the percentage value, the more likely the event will occur.)
Y axis: *Potential Impact% (The higher the percentage value, the more likely the price will deviate.)
Z axis (Grey to Black): *Technical Analysis%
CAC 40 Index Financial Outlook and Forecast
The CAC 40, representing the largest publicly traded companies in France, is anticipated to experience moderate growth in the near-term, driven by factors such as robust economic performance within the Eurozone. The current global economic climate, however, presents a complex interplay of influences. Inflationary pressures remain a persistent challenge, impacting consumer spending and corporate profitability. The potential for a sustained economic slowdown in key international markets, coupled with geopolitical uncertainties, adds a layer of volatility to the overall outlook. The performance of sectors reliant on international trade and investment will likely be heavily influenced by these global dynamics. Factors such as interest rate policies adopted by central banks globally, currency fluctuations, and evolving geopolitical tensions will significantly impact the CAC 40's trajectory.
Furthermore, the resilience of the French economy itself will play a critical role in the index's performance. Government policies aimed at stimulating domestic demand and fostering innovation could contribute to a more favorable environment for the listed companies. However, the French economy also faces specific challenges, including the need to address persistent labor market issues and the structural reforms required to enhance competitiveness. These factors could affect the ability of CAC 40 companies to achieve profitability targets. Regulatory changes, particularly those affecting environmental sustainability and corporate social responsibility will likely influence the valuations and performance of different sectors within the index. The success of French companies in adapting to these changes will be crucial.
The recent performance of various sectors within the CAC 40 has been mixed. Energy-related companies have benefited from surging energy prices but face headwinds as these prices moderate. Technology sectors generally demonstrate resilience to economic downturns, although valuations could be impacted by shifting investor sentiment and the interest rate environment. The performance of consumer-facing companies will likely reflect consumer confidence and spending habits. The degree of resilience displayed by the French companies within the index will be an important indicator of their ability to navigate the ongoing economic complexities. Companies exhibiting strong fundamentals and strategic adaptability are more likely to achieve positive results.
Predicting the precise trajectory of the CAC 40 is inherently challenging. A positive outlook anticipates continued, albeit moderate, growth, driven by strong domestic fundamentals and the resilience of key sectors. However, risks associated with global economic slowdown, persistent inflation, and geopolitical instability could negatively impact investor sentiment and market performance. Further, unexpected shifts in regulatory environments or technological disruptions could significantly affect specific sectors. This uncertainty warrants a cautious, nuanced approach to investment decisions. The prediction of positive growth carries the risk of market fluctuations and unexpected events impacting the index negatively. A negative outlook could stem from significant global economic disruptions, resulting in reduced corporate profits and lower investor confidence, leading to a substantial market decline for the CAC 40.
Rating | Short-Term | Long-Term Senior |
---|---|---|
Outlook | B2 | B1 |
Income Statement | C | C |
Balance Sheet | B3 | Baa2 |
Leverage Ratios | Ba3 | B2 |
Cash Flow | B3 | B2 |
Rates of Return and Profitability | B1 | B3 |
*An aggregate rating for an index summarizes the overall sentiment towards the companies it includes. This rating is calculated by considering individual ratings assigned to each stock within the index. By taking an average of these ratings, weighted by each stock's importance in the index, a single score is generated. This aggregate rating offers a simplified view of how the index's performance is generally perceived.
How does neural network examine financial reports and understand financial state of the company?
References
- C. Szepesvári. Algorithms for Reinforcement Learning. Synthesis Lectures on Artificial Intelligence and Machine Learning. Morgan & Claypool Publishers, 2010
- Hartford J, Lewis G, Taddy M. 2016. Counterfactual prediction with deep instrumental variables networks. arXiv:1612.09596 [stat.AP]
- Jacobs B, Donkers B, Fok D. 2014. Product Recommendations Based on Latent Purchase Motivations. Rotterdam, Neth.: ERIM
- Athey S, Imbens GW. 2017b. The state of applied econometrics: causality and policy evaluation. J. Econ. Perspect. 31:3–32
- Holland PW. 1986. Statistics and causal inference. J. Am. Stat. Assoc. 81:945–60
- S. Bhatnagar, H. Prasad, and L. Prashanth. Stochastic recursive algorithms for optimization, volume 434. Springer, 2013
- Rumelhart DE, Hinton GE, Williams RJ. 1986. Learning representations by back-propagating errors. Nature 323:533–36