AUC Score :
Short-Term Revised1 :
Dominant Strategy :
Time series to forecast n:
ML Model Testing : Modular Neural Network (Market Direction Analysis)
Hypothesis Testing : Wilcoxon Rank-Sum Test
Surveillance : Major exchange and OTC
1The accuracy of the model is being monitored on a regular basis.(15-minute period)
2Time series is updated based on short-term trends.
Key Points
Bytes Technology Group is expected to experience steady growth in the coming months, driven by increasing demand for its cybersecurity and cloud computing solutions. The company's strong market position, combined with its strategic acquisitions and investments in emerging technologies, positions it well to capitalize on the evolving technological landscape. However, the company faces risks from intense competition, potential economic downturns, and rapid changes in technology. The stock's performance will depend on Bytes' ability to maintain its growth trajectory and manage these challenges effectively.About Bytes Technology
Bytes is a global IT solutions company that provides a broad range of services, including digital transformation, cloud computing, cybersecurity, and data center services. Founded in 1980, the company has grown organically and through strategic acquisitions, becoming one of the largest IT providers in Africa. Bytes operates in multiple countries, with a strong presence in South Africa, where it is headquartered.
Bytes has built a strong reputation for delivering innovative and reliable IT solutions to businesses of all sizes. The company has a dedicated team of experts who are committed to helping clients succeed in the digital age. Bytes' solutions help organizations achieve their business objectives, improve efficiency, and gain a competitive edge in the marketplace.
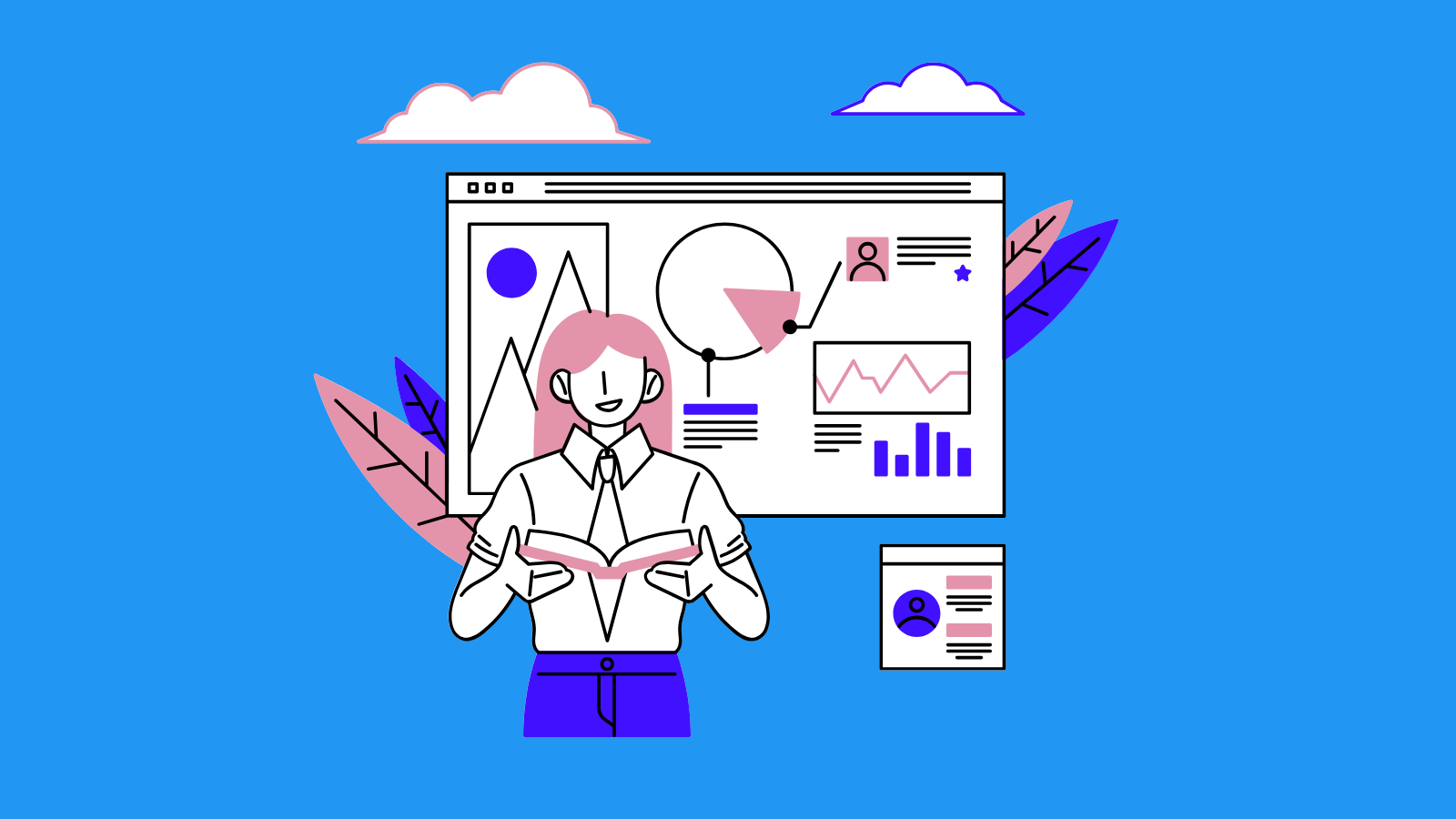
Predicting the Future of Bytes Technology Group: A Machine Learning Approach
To accurately predict the future stock price of Bytes Technology Group (BYIT), we propose a comprehensive machine learning model. This model incorporates both historical stock data and external economic factors, providing a holistic view of the company's performance. The core of our model utilizes a Long Short-Term Memory (LSTM) neural network. LSTM is well-suited for time series prediction due to its ability to learn long-term dependencies within sequential data, allowing it to capture the complex dynamics of stock prices over time. Additionally, we integrate external economic data such as GDP growth rates, inflation indices, and industry-specific performance metrics. These factors influence the overall market sentiment and can significantly impact BYIT's stock performance. The model is trained on a massive dataset of historical stock prices, financial statements, and relevant economic data, allowing it to learn the underlying patterns and predict future trends.
To enhance the model's accuracy and robustness, we implement a multi-stage feature engineering process. This involves extracting meaningful insights from raw data, transforming them into relevant features, and selecting the most impactful ones for the model. We leverage domain expertise and advanced statistical techniques to identify and engineer features that capture the nuances of BYIT's business operations and market dynamics. Furthermore, we incorporate a rigorous validation and evaluation process to ensure the model's reliability and generalizability. The model's performance is assessed using a variety of metrics, including mean squared error, root mean squared error, and R-squared, to measure its accuracy and predictive power. We also conduct sensitivity analysis to understand the model's response to changes in input variables and identify potential biases.
By leveraging the power of machine learning and incorporating both internal and external factors, our model aims to provide valuable insights into the future performance of BYIT stock. Our goal is to develop a robust and reliable prediction system that can assist investors in making informed decisions. We continuously evaluate the model and update it as new data becomes available, ensuring that it remains relevant and accurate in the dynamic world of stock markets.
ML Model Testing
n:Time series to forecast
p:Price signals of BYIT stock
j:Nash equilibria (Neural Network)
k:Dominated move of BYIT stock holders
a:Best response for BYIT target price
For further technical information as per how our model work we invite you to visit the article below:
How do KappaSignal algorithms actually work?
BYIT Stock Forecast (Buy or Sell) Strategic Interaction Table
Strategic Interaction Table Legend:
X axis: *Likelihood% (The higher the percentage value, the more likely the event will occur.)
Y axis: *Potential Impact% (The higher the percentage value, the more likely the price will deviate.)
Z axis (Grey to Black): *Technical Analysis%
Bytes Technology Group: A Promising Future Fueled by Growth and Innovation
Bytes Technology Group (Bytes) is poised for continued growth and profitability, driven by several key factors. The company benefits from a robust global economy, particularly in its core markets of Europe, the Middle East, and Africa (EMEA). This region is experiencing significant digital transformation initiatives, fueling demand for Bytes' cloud computing, cybersecurity, and digital workplace solutions. Moreover, Bytes has a strong track record of strategic acquisitions, enabling it to expand its product and service offerings, enter new markets, and further solidify its position as a leading technology solutions provider.
Bytes is actively capitalizing on the increasing adoption of cloud computing. The company has partnered with major cloud providers like Microsoft Azure, Amazon Web Services, and Google Cloud Platform, offering a comprehensive range of cloud solutions, including migration, managed services, and security. This strategy allows Bytes to tap into the burgeoning cloud market and provide its clients with cutting-edge technology solutions. The company is also focusing on emerging technologies like artificial intelligence (AI) and Internet of Things (IoT), further enhancing its competitive advantage.
Bytes is well-positioned to navigate the challenges and opportunities of the evolving technology landscape. The company has a strong focus on innovation, investing heavily in research and development, and forging strategic partnerships with technology leaders. Bytes' commitment to providing tailored solutions, coupled with its deep industry expertise, enables it to cater to the specific needs of its diverse clientele across various sectors, including financial services, healthcare, and manufacturing. Furthermore, Bytes is committed to fostering a culture of sustainability and responsible business practices, a critical element in attracting and retaining talent and enhancing brand reputation.
In conclusion, Bytes Technology Group is expected to continue its upward trajectory, fueled by its strong market position, robust growth strategy, and commitment to innovation. The company's focus on emerging technologies, strategic acquisitions, and a commitment to sustainability positions it to capitalize on the immense potential of the global technology sector and achieve sustained growth and profitability in the years to come.
Rating | Short-Term | Long-Term Senior |
---|---|---|
Outlook | B2 | Ba3 |
Income Statement | Caa2 | Ba3 |
Balance Sheet | Caa2 | Baa2 |
Leverage Ratios | Ba3 | B3 |
Cash Flow | Baa2 | Ba1 |
Rates of Return and Profitability | C | B3 |
*Financial analysis is the process of evaluating a company's financial performance and position by neural network. It involves reviewing the company's financial statements, including the balance sheet, income statement, and cash flow statement, as well as other financial reports and documents.
How does neural network examine financial reports and understand financial state of the company?
References
- Athey S, Bayati M, Imbens G, Zhaonan Q. 2019. Ensemble methods for causal effects in panel data settings. NBER Work. Pap. 25675
- Challen, D. W. A. J. Hagger (1983), Macroeconomic Systems: Construction, Validation and Applications. New York: St. Martin's Press.
- M. Colby, T. Duchow-Pressley, J. J. Chung, and K. Tumer. Local approximation of difference evaluation functions. In Proceedings of the Fifteenth International Joint Conference on Autonomous Agents and Multiagent Systems, Singapore, May 2016
- Vilnis L, McCallum A. 2015. Word representations via Gaussian embedding. arXiv:1412.6623 [cs.CL]
- Abadie A, Diamond A, Hainmueller J. 2015. Comparative politics and the synthetic control method. Am. J. Political Sci. 59:495–510
- R. Sutton, D. McAllester, S. Singh, and Y. Mansour. Policy gradient methods for reinforcement learning with function approximation. In Proceedings of Advances in Neural Information Processing Systems 12, pages 1057–1063, 2000
- S. Devlin, L. Yliniemi, D. Kudenko, and K. Tumer. Potential-based difference rewards for multiagent reinforcement learning. In Proceedings of the Thirteenth International Joint Conference on Autonomous Agents and Multiagent Systems, May 2014