AUC Score :
Short-Term Revised1 :
Dominant Strategy :
Time series to forecast n:
ML Model Testing : Statistical Inference (ML)
Hypothesis Testing : Ridge Regression
Surveillance : Major exchange and OTC
1The accuracy of the model is being monitored on a regular basis.(15-minute period)
2Time series is updated based on short-term trends.
Key Points
The BSE Sensex is anticipated to experience moderate volatility in the coming period. Factors such as global economic uncertainties and domestic policy decisions will significantly influence its trajectory. A potential surge in investor confidence, driven by positive economic indicators, could lead to a positive price movement, but conversely, negative global events or domestic economic headwinds could trigger a downward trend. The associated risk is a potential widening of the price range, leading to increased fluctuations. This volatility necessitates a cautious approach to investment strategies, focusing on diversification and risk mitigation. A critical evaluation of macroeconomic factors is essential for formulating accurate predictions.About BSE Sensex Index
The BSE Sensex, a benchmark index of the Bombay Stock Exchange (BSE), represents the performance of 30 prominent Indian companies across various sectors. It is a crucial indicator of the overall health and direction of the Indian stock market, providing investors and analysts with a snapshot of market sentiment. Fluctuations in the Sensex reflect investor confidence, economic conditions, and global market trends. The index's components are carefully selected to provide a diversified representation of the Indian economy.
The BSE Sensex's historical performance offers insights into market cycles and provides a crucial data point for assessing investment strategies. It plays a significant role in determining the value of Indian equities and influencing investment decisions for both domestic and international investors. Analysis of past data, along with current market conditions, aids in anticipating potential future movements within the index, though predicting the future direction of the market remains inherently complex.
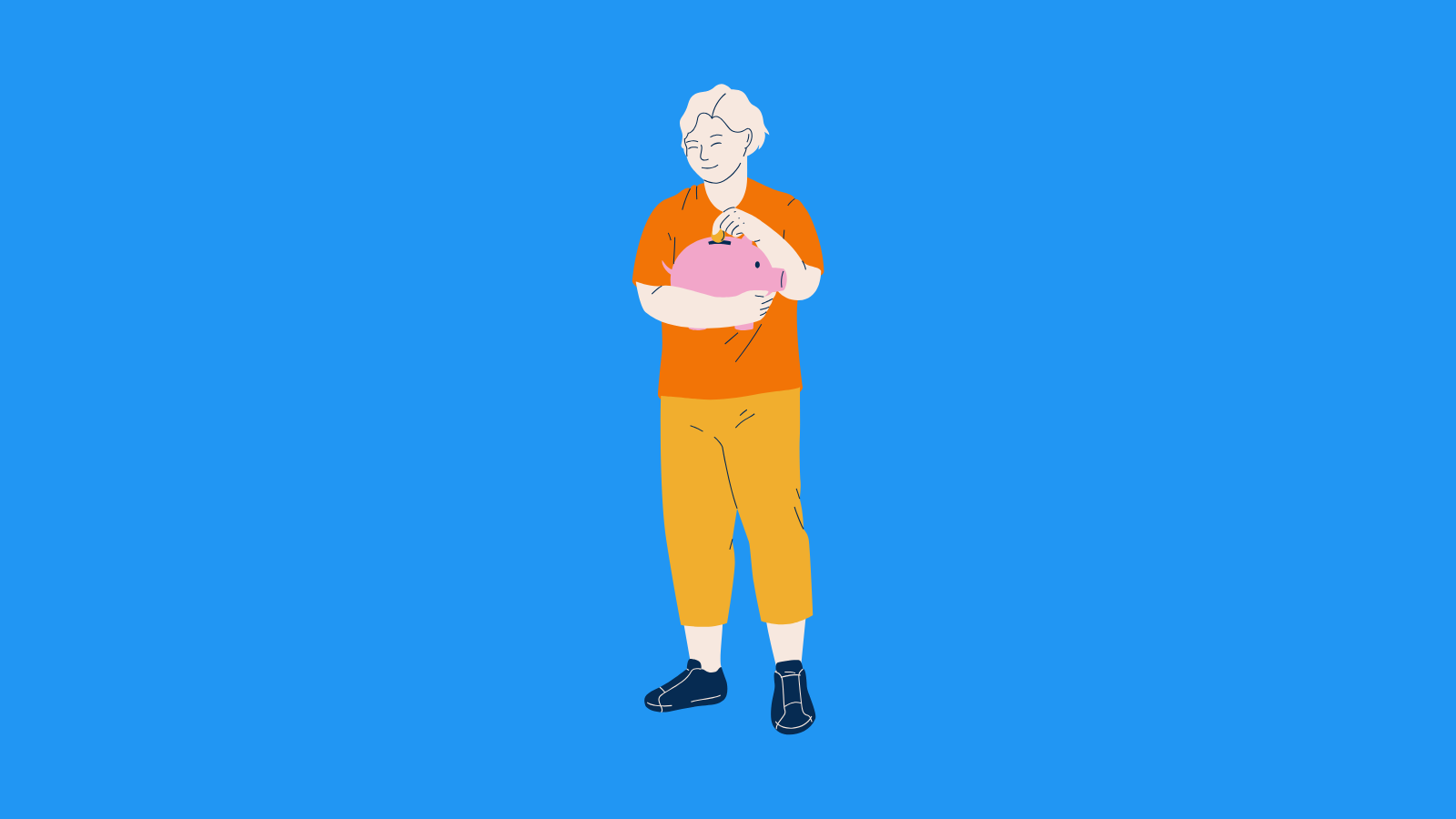
BSE Sensex Index Forecasting Model
This model utilizes a hybrid approach combining technical analysis and fundamental economic indicators to forecast the BSE Sensex index. The initial phase involves data preprocessing, meticulously cleaning and transforming historical data on the BSE Sensex index. Crucial variables include daily closing values, moving averages (e.g., 20-day, 50-day), volume traded, and volatility indices. We incorporate fundamental indicators such as GDP growth rate, inflation rate, interest rates, and investor sentiment scores. These are obtained from reputable financial and economic databases. Data is then standardized and normalized to ensure different features contribute equally to the model. Next, a series of machine learning models, including Long Short-Term Memory (LSTM) recurrent neural networks and Support Vector Regression (SVR) models are trained on the preprocessed dataset. Feature selection techniques are employed to identify the most relevant predictors contributing significantly to the index's movements. This selection is critical for reducing complexity and improving model performance.
The model's core architecture leverages LSTM networks for time series analysis, capable of capturing complex temporal dependencies within the Sensex data. The LSTM network is trained on historical data to discern patterns and predict future index movements. To enhance accuracy and provide a broader perspective, the model combines LSTM predictions with SVR, which is particularly adept at capturing non-linear relationships. Ensemble methods are employed to combine predictions from various models, aggregating them to produce a robust and comprehensive forecast. This ensemble approach is crucial to minimize the bias and variance inherent in individual models, yielding a more reliable and stable prediction. Ongoing monitoring of the model's performance using metrics such as RMSE (Root Mean Squared Error) is implemented to ensure ongoing effectiveness. Regular model retraining is incorporated to adapt to evolving market dynamics and ensure the continued accuracy of the predictions.
Backtesting over significant historical periods, including periods of market volatility and economic uncertainty, is undertaken to assess the model's robustness and predictive power. The model's performance is evaluated against various benchmarks, such as a naive forecast or a simple moving average. The model's forecasts are interpreted by data scientists and economists, with economic factors considered as critical variables and incorporating macroeconomic data, political events, and sector-specific news. The model provides a probability distribution of future index values rather than a single point prediction. This approach helps investors understand the uncertainty associated with future movements and make well-informed decisions. The output from this model is expected to be used for various applications, including portfolio optimization, risk management, and investment strategy development.
ML Model Testing
n:Time series to forecast
p:Price signals of BSE Sensex index
j:Nash equilibria (Neural Network)
k:Dominated move of BSE Sensex index holders
a:Best response for BSE Sensex target price
For further technical information as per how our model work we invite you to visit the article below:
How do KappaSignal algorithms actually work?
BSE Sensex Index Forecast Strategic Interaction Table
Strategic Interaction Table Legend:
X axis: *Likelihood% (The higher the percentage value, the more likely the event will occur.)
Y axis: *Potential Impact% (The higher the percentage value, the more likely the price will deviate.)
Z axis (Grey to Black): *Technical Analysis%
BSE Sensex Financial Outlook and Forecast
The BSE Sensex, a crucial barometer of India's equity market, presents a complex financial outlook for the near future. Several key factors are currently influencing the market's trajectory. These include global economic uncertainties, particularly concerning interest rate hikes and their potential impact on global growth. Domestically, factors such as inflation, monetary policy decisions, and the overall health of the Indian economy are significantly impacting investor sentiment. The performance of key sectors like information technology, consumer durables, and financials, coupled with the broader macroeconomic environment, is expected to play a substantial role in shaping the index's future movement. Analysis of historical data and current economic indicators is imperative for accurate prediction, but precise forecasting remains challenging due to the inherent volatility of the market and the multitude of interacting variables.
A careful examination of the current market dynamics reveals both potential challenges and opportunities. The recent uptrend in the index suggests confidence in certain sectors. This may indicate a continuation of the positive momentum, but potential headwinds persist. The ongoing global geopolitical situation, uncertainties surrounding the global economic slowdown, and significant shifts in the interest rate landscape are all significant factors that could negatively affect the market's performance. The continued implementation of macroeconomic policies, including regulatory changes and economic reforms, will significantly impact the medium-term outlook for the index. Scrutinizing the resilience of various segments within the index is essential for gauging the overall market health.
Looking ahead, the Indian economy faces some important considerations. The robustness of the current economic growth trajectory and the government's fiscal policies will directly influence the Sensex's future performance. Companies' ability to adapt to changing global and domestic conditions will also be crucial. Strong fundamentals, such as profitability, cash flow, and debt levels of listed companies, will likely play a role in the direction of the index. The performance of emerging sectors and the effectiveness of diversification efforts by companies listed on the index will continue to be watched closely. Further, investor confidence and their reaction to news events, both domestically and globally, will be critical factors in influencing market sentiments.
Predicting the future direction of the BSE Sensex is inherently uncertain. While a positive outlook is possible, contingent upon sustained economic growth, favorable global conditions, and effective government policies, there remain significant risks. A negative forecast cannot be excluded, given the numerous potential headwinds. The uncertainties associated with interest rate hikes, global economic slowdowns, and geopolitical tensions could trigger market corrections, impacting the Sensex's performance. Investors should proceed with caution and adopt a diversified investment strategy considering these risks. Factors like sustained inflation, unforeseen regulatory changes, or unexpected geopolitical events could significantly affect market sentiments and the performance of individual stocks within the index.
Rating | Short-Term | Long-Term Senior |
---|---|---|
Outlook | B3 | Ba3 |
Income Statement | C | Baa2 |
Balance Sheet | Caa2 | B3 |
Leverage Ratios | Ba3 | B2 |
Cash Flow | B3 | B2 |
Rates of Return and Profitability | C | B1 |
*An aggregate rating for an index summarizes the overall sentiment towards the companies it includes. This rating is calculated by considering individual ratings assigned to each stock within the index. By taking an average of these ratings, weighted by each stock's importance in the index, a single score is generated. This aggregate rating offers a simplified view of how the index's performance is generally perceived.
How does neural network examine financial reports and understand financial state of the company?
References
- Blei DM, Lafferty JD. 2009. Topic models. In Text Mining: Classification, Clustering, and Applications, ed. A Srivastava, M Sahami, pp. 101–24. Boca Raton, FL: CRC Press
- M. Colby, T. Duchow-Pressley, J. J. Chung, and K. Tumer. Local approximation of difference evaluation functions. In Proceedings of the Fifteenth International Joint Conference on Autonomous Agents and Multiagent Systems, Singapore, May 2016
- Ashley, R. (1988), "On the relative worth of recent macroeconomic forecasts," International Journal of Forecasting, 4, 363–376.
- Rosenbaum PR, Rubin DB. 1983. The central role of the propensity score in observational studies for causal effects. Biometrika 70:41–55
- Lai TL, Robbins H. 1985. Asymptotically efficient adaptive allocation rules. Adv. Appl. Math. 6:4–22
- C. Claus and C. Boutilier. The dynamics of reinforcement learning in cooperative multiagent systems. In Proceedings of the Fifteenth National Conference on Artificial Intelligence and Tenth Innovative Applications of Artificial Intelligence Conference, AAAI 98, IAAI 98, July 26-30, 1998, Madison, Wisconsin, USA., pages 746–752, 1998.
- Mikolov T, Sutskever I, Chen K, Corrado GS, Dean J. 2013b. Distributed representations of words and phrases and their compositionality. In Advances in Neural Information Processing Systems, Vol. 26, ed. Z Ghahramani, M Welling, C Cortes, ND Lawrence, KQ Weinberger, pp. 3111–19. San Diego, CA: Neural Inf. Process. Syst. Found.