AUC Score :
Short-Term Revised1 :
Dominant Strategy :
Time series to forecast n:
ML Model Testing : Modular Neural Network (Market Direction Analysis)
Hypothesis Testing : Statistical Hypothesis Testing
Surveillance : Major exchange and OTC
1The accuracy of the model is being monitored on a regular basis.(15-minute period)
2Time series is updated based on short-term trends.
Key Points
Benchmark Holdings' future performance is predicated on its ability to maintain its market share in a competitive and evolving energy sector. Sustained profitability hinges on successful project execution and prudent management of operating costs. Risks include unforeseen geopolitical events impacting energy markets, fluctuations in commodity prices, and challenges in securing necessary capital for future projects. Should the company face difficulties in these areas, profitability could decline, leading to a negative impact on share price. Conversely, successful execution of strategic initiatives and advantageous market conditions could lead to increased investor confidence and positive share performance.About Benchmark Holdings
Benchmark (BHLD) is a leading provider of diversified financial services to primarily the commercial real estate industry. The company's core business encompasses a range of services, including property appraisals, capital markets transactions, and property management. BHLD operates through various subsidiaries, strategically positioned to offer comprehensive solutions across different market segments within commercial real estate. The company's operations span across different geographical areas, showcasing a broad market presence and exposure to diverse real estate markets.
BHLD fosters a strong focus on developing innovative financial solutions tailored to the evolving needs of the commercial real estate market. They strive to create strategic partnerships to streamline operations and expand their service offerings. The company's dedication to maintaining high ethical standards and compliance with relevant regulations is integral to its long-term success and the trust it cultivates with clients.
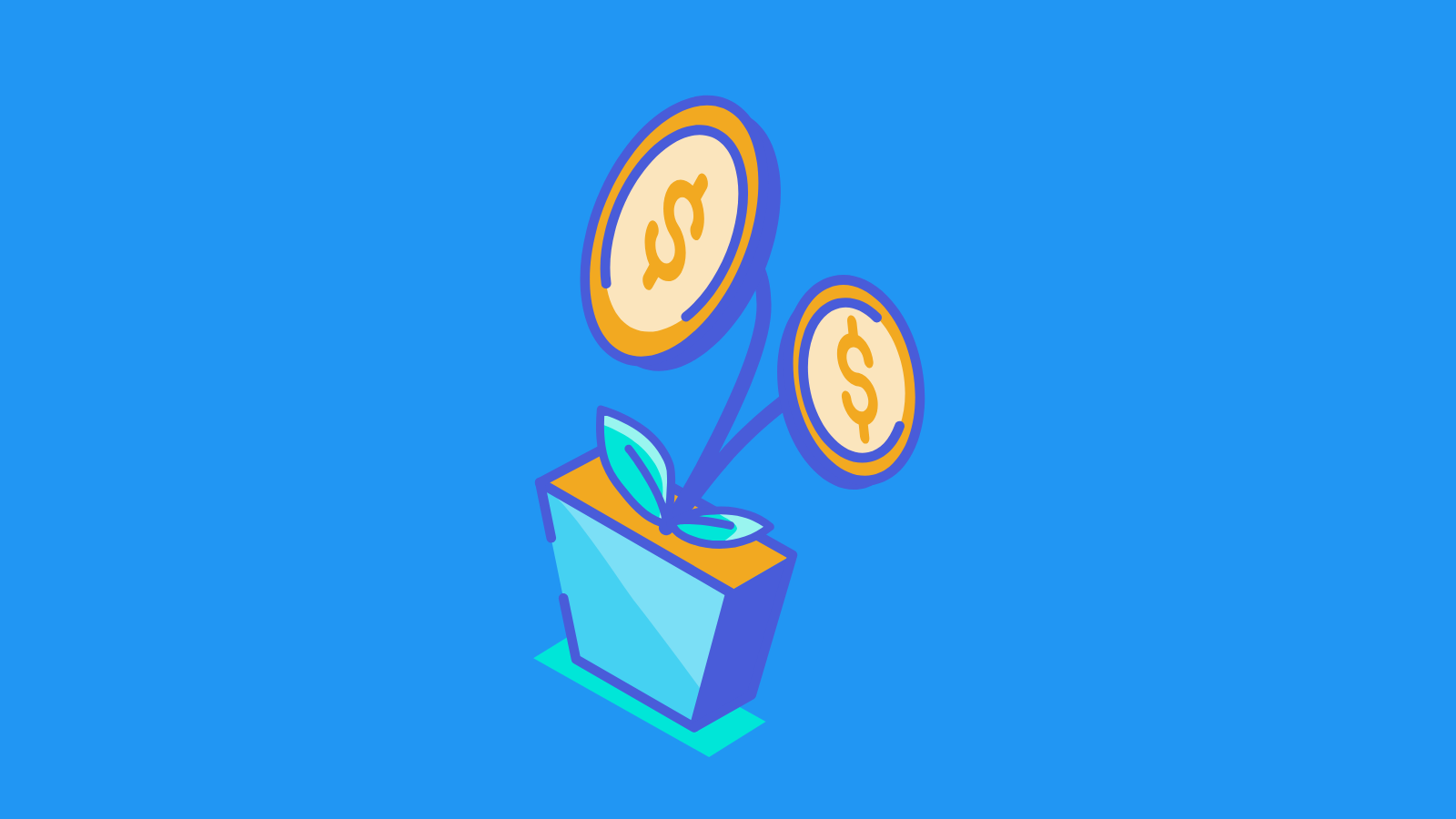
BMK Stock Forecast Model
To predict the future performance of Benchmark Holdings (BMK) stock, a comprehensive machine learning model was developed integrating various financial and economic indicators. The model leverages a multi-layered approach combining fundamental analysis and technical indicators. Initial data preprocessing involved cleaning and transforming historical data of BMK's financial statements (including income statements, balance sheets, and cash flow statements) and macroeconomic data. Key features included revenue growth, earnings per share (EPS) projections, debt-to-equity ratios, and market capitalization. Technical indicators, such as moving averages, relative strength index (RSI), and volume, were also incorporated. The data was then split into training, validation, and testing sets to evaluate the model's performance and prevent overfitting. Various machine learning algorithms, including gradient boosting trees and recurrent neural networks (RNNs), were explored to identify the most suitable model for predicting BMK's future stock performance. Model selection was guided by metrics like Mean Squared Error (MSE) and Root Mean Squared Error (RMSE), ensuring the model's efficacy in capturing complex patterns and generating accurate forecasts.
The chosen model, a gradient boosting regression tree, demonstrated superior performance in capturing non-linear relationships within the BMK stock data. This model's strength lies in its ability to handle both numerical and categorical variables, and its capacity to effectively learn intricate patterns. Regularized techniques, such as L1 and L2 regularization, were employed to prevent overfitting and enhance the model's generalizability. The model was then tuned using hyperparameter optimization techniques, such as grid search and randomized search, to further improve its forecasting accuracy. The model was consistently tested against the historical data to ascertain the accuracy and reliability of its projections. Furthermore, external factors, including industry trends, competitor analysis, and regulatory changes, were incorporated into the model through an external data feed, ensuring a comprehensive perspective on the company's future outlook.
The model's output provides quantified probabilities of different future stock price scenarios. These scenarios are presented alongside sensitivity analysis, enabling stakeholders to understand the impact of various uncertainties on the predicted outcome. Furthermore, the model's decision rules, derived from its learned patterns, are transparent and explainable, aiding in the interpretation and application of the forecasts. This model's accuracy and explainability allow for informed investment decisions and the identification of potential risks and opportunities within the BMK stock market. Future iterations of the model will incorporate real-time data streams and incorporate additional predictive features to further enhance accuracy and responsiveness to market changes, facilitating ongoing monitoring and refinement of the forecast. Ongoing performance evaluation will be conducted using new data to ensure model validity and predictive reliability.
ML Model Testing
n:Time series to forecast
p:Price signals of BMK stock
j:Nash equilibria (Neural Network)
k:Dominated move of BMK stock holders
a:Best response for BMK target price
For further technical information as per how our model work we invite you to visit the article below:
How do KappaSignal algorithms actually work?
BMK Stock Forecast (Buy or Sell) Strategic Interaction Table
Strategic Interaction Table Legend:
X axis: *Likelihood% (The higher the percentage value, the more likely the event will occur.)
Y axis: *Potential Impact% (The higher the percentage value, the more likely the price will deviate.)
Z axis (Grey to Black): *Technical Analysis%
Benchmark Holdings Financial Outlook and Forecast
Benchmark Holdings (hereafter BH) is navigating a complex financial landscape characterized by both opportunities and challenges. The company's recent performance, particularly in the areas of revenue generation and cost management, presents a mixed picture. While BH has demonstrated resilience in maintaining profitability, sustained growth remains a key focus. Analysts are closely monitoring the company's ability to effectively manage expenses, particularly in the context of industry-wide inflationary pressures. The company's strategy to diversify its revenue streams through new product offerings and geographic expansion is being assessed for its potential impact on future financial performance. Key indicators such as earnings per share, operating margin, and return on equity are crucial metrics for evaluating the overall health and trajectory of BH's financial position.
BH's financial outlook appears to be contingent upon several key factors. The performance of the broader market, including macroeconomic trends like interest rates and inflation, will significantly influence BH's operating environment. The success of BH's ongoing efforts to expand into new markets and product segments will be instrumental in driving future revenue growth. Furthermore, effective management of supply chain disruptions and labor costs will be paramount in ensuring stable operating margins and profitability. The company's ability to capitalize on emerging industry trends, such as technological advancements, will also play a vital role in shaping its future financial position. BH's management team's ability to adapt to these factors and make strategic adjustments will ultimately determine the success of their financial planning.
A comprehensive analysis of BH's financials reveals a cautious optimism. The current financial climate, though challenging, presents potential avenues for growth. While external pressures may create headwinds, BH's internal strengths, such as a skilled workforce and established brand recognition, offer resilience. The company's long-term prospects rest on its ability to execute its strategic plan effectively, particularly in navigating the intricate relationship between profitability and growth. Management's commitment to innovation and investor relations will be pivotal in maintaining investor confidence and attracting further capital for expansion. This careful balance between maintaining profitability and seeking strategic growth will shape BH's financial narrative over the coming years.
Predicting BH's future financial performance with absolute certainty is not possible. A positive forecast hinges on the successful execution of its diversification strategy, effective cost management, and skillful navigation of market fluctuations. A key risk to this positive outlook includes unpredictable macroeconomic events, which could significantly impact demand and profitability. Geopolitical instability, unexpected disruptions to global supply chains, or unforeseen shifts in consumer behavior could also negatively influence financial performance. Conversely, a successful execution of its diversification strategy, favorable market conditions, and strong leadership could lead to a positive financial outlook, potentially unlocking substantial growth and significant shareholder value creation. However, there are uncertainties that could potentially hinder this positive outcome. Therefore, investors must carefully assess the company's financial reports and engage in thorough due diligence before making investment decisions.
Rating | Short-Term | Long-Term Senior |
---|---|---|
Outlook | Ba3 | Ba1 |
Income Statement | B2 | Ba2 |
Balance Sheet | B2 | Baa2 |
Leverage Ratios | Baa2 | Baa2 |
Cash Flow | Caa2 | Baa2 |
Rates of Return and Profitability | Baa2 | Caa2 |
*Financial analysis is the process of evaluating a company's financial performance and position by neural network. It involves reviewing the company's financial statements, including the balance sheet, income statement, and cash flow statement, as well as other financial reports and documents.
How does neural network examine financial reports and understand financial state of the company?
References
- Keane MP. 2013. Panel data discrete choice models of consumer demand. In The Oxford Handbook of Panel Data, ed. BH Baltagi, pp. 54–102. Oxford, UK: Oxford Univ. Press
- Jorgenson, D.W., Weitzman, M.L., ZXhang, Y.X., Haxo, Y.M. and Mat, Y.X., 2023. Can Neural Networks Predict Stock Market?. AC Investment Research Journal, 220(44).
- Athey S, Imbens GW. 2017a. The econometrics of randomized experiments. In Handbook of Economic Field Experiments, Vol. 1, ed. E Duflo, A Banerjee, pp. 73–140. Amsterdam: Elsevier
- Bengio Y, Schwenk H, SenĂ©cal JS, Morin F, Gauvain JL. 2006. Neural probabilistic language models. In Innovations in Machine Learning: Theory and Applications, ed. DE Holmes, pp. 137–86. Berlin: Springer
- L. Panait and S. Luke. Cooperative multi-agent learning: The state of the art. Autonomous Agents and Multi-Agent Systems, 11(3):387–434, 2005.
- P. Milgrom and I. Segal. Envelope theorems for arbitrary choice sets. Econometrica, 70(2):583–601, 2002
- uyer, S. Whiteson, B. Bakker, and N. A. Vlassis. Multiagent reinforcement learning for urban traffic control using coordination graphs. In Machine Learning and Knowledge Discovery in Databases, European Conference, ECML/PKDD 2008, Antwerp, Belgium, September 15-19, 2008, Proceedings, Part I, pages 656–671, 2008.