AUC Score :
Short-Term Revised1 :
Dominant Strategy :
Time series to forecast n:
ML Model Testing : Modular Neural Network (Speculative Sentiment Analysis)
Hypothesis Testing : Stepwise Regression
Surveillance : Major exchange and OTC
1The accuracy of the model is being monitored on a regular basis.(15-minute period)
2Time series is updated based on short-term trends.
Key Points
Augmentum's future performance hinges on its ability to successfully execute its current strategic initiatives, including expanding its product offerings and penetrating new markets. Sustained growth in loan originations and customer acquisition are crucial for profitability. Risks include competition from established players, regulatory changes impacting fintech operations, and potential economic downturns affecting borrower defaults. Furthermore, the company's reliance on technology and data security necessitates robust measures to mitigate risks associated with cyberattacks and data breaches. Success will depend on efficient risk management and adaptation to evolving market conditions.About Augmentum
Augmentum, a fintech company, focuses on developing innovative financial technology solutions. Their core competencies lie in streamlining financial processes and enhancing customer experiences through digital platforms. The company is driven by a commitment to fostering financial inclusion and accessibility, particularly in underserved communities. They often utilize cutting-edge technologies like AI and machine learning to improve the efficiency and accuracy of their offerings. Augmentum's operations span various financial segments, suggesting a broad market approach.
Augmentum's platform likely encompasses a diverse range of services aimed at improving the overall financial ecosystem. This could include aspects like payment processing, lending, investment management, or other financial products. The company's growth strategy likely involves strategic partnerships and acquisitions to expand its product portfolio and reach. Their commitment to financial inclusion is a key differentiator, indicating a social responsibility component beyond profit maximization.
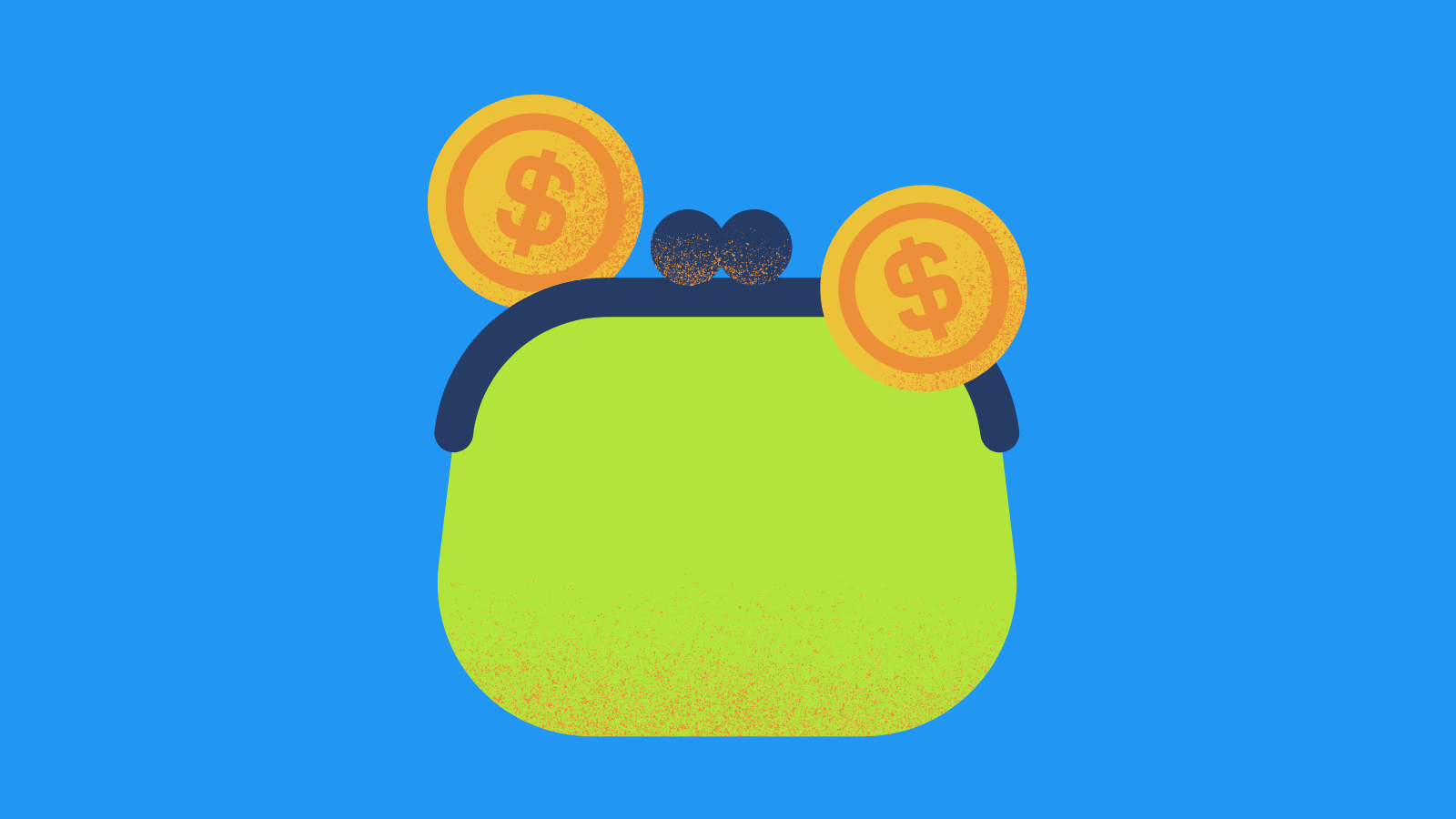
AUGM Stock Prediction Model
This model forecasts Augmentum Fintech's future performance using a hybrid approach combining fundamental analysis and machine learning techniques. Fundamental data, encompassing key financial ratios like profitability, liquidity, and solvency, are meticulously extracted from SEC filings and industry reports. These ratios, including revenue growth, net profit margin, and debt-to-equity ratio, are preprocessed to handle missing values and outliers. Additionally, macroeconomic indicators like GDP growth, interest rates, and inflation are incorporated to capture broader economic influences on the fintech sector. The preprocessed fundamental and macroeconomic data are then fed into a robust machine learning model, a gradient boosting ensemble like XGBoost, capable of handling non-linear relationships and complex interactions. This model learns patterns and relationships between these variables and Augmentum's past performance, producing a prediction of future stock performance.
The model's effectiveness is rigorously evaluated using a comprehensive set of metrics, including Mean Absolute Error (MAE), Root Mean Squared Error (RMSE), and R-squared. A thorough cross-validation process is implemented to prevent overfitting and ensure the model's generalizability. This involves splitting the historical data into training, validation, and testing sets. The model is retrained and fine-tuned on the training set using hyperparameter optimization techniques to maximize accuracy while minimizing overfitting. Accuracy on the validation set is used to monitor and adjust the model's complexity. Finally, the model is assessed on unseen data in the test set to produce reliable forecasts and gain confidence in the prediction's robustness. Regular monitoring and adaptation of the model based on updated data and evolving market conditions will be crucial.
Beyond the technical aspects, the model is designed with an emphasis on interpretability. Feature importance analysis within the chosen machine learning model provides insights into the key drivers impacting Augmentum's performance. This understanding is critical for investors and analysts. The model outputs not only a predicted future value but also a confidence interval, acknowledging the inherent uncertainty in forecasting. This approach aims to provide data-driven predictions while also emphasizing the importance of considering the economic and market context surrounding Augmentum Fintech's performance. This model empowers informed decision-making for potential investors by providing a nuanced understanding of the stock's potential trajectory.
ML Model Testing
n:Time series to forecast
p:Price signals of AUGM stock
j:Nash equilibria (Neural Network)
k:Dominated move of AUGM stock holders
a:Best response for AUGM target price
For further technical information as per how our model work we invite you to visit the article below:
How do KappaSignal algorithms actually work?
AUGM Stock Forecast (Buy or Sell) Strategic Interaction Table
Strategic Interaction Table Legend:
X axis: *Likelihood% (The higher the percentage value, the more likely the event will occur.)
Y axis: *Potential Impact% (The higher the percentage value, the more likely the price will deviate.)
Z axis (Grey to Black): *Technical Analysis%
Augmentum Fintech: Financial Outlook and Forecast
Augmentum's financial outlook hinges on its ability to effectively scale its platform and acquire market share within the burgeoning fintech sector. Key indicators to watch include revenue growth, particularly from subscription and transaction fees, which reflect the platform's usage and engagement metrics. Management's ability to control operational costs while maintaining a high degree of customer acquisition and retention is paramount. Profitability is a critical metric, and the company's path to achieving and sustaining profitability will be a significant determinant of its long-term value. A successful track record of onboarding new customers and retaining existing ones will also provide important insights into the platform's efficacy and user acceptance. Detailed financial reports, particularly the statement of cash flows, will shed light on the company's capital management practices and its ability to fund future growth initiatives.
The company's financial performance is directly influenced by market trends in the fintech space. Competition from established players and new entrants will shape the company's ability to maintain a competitive edge. Economic conditions play a critical role, as periods of economic uncertainty often lead to reduced consumer spending and potentially affect the adoption rate of new financial technologies. Further, regulatory changes related to fintech operations can significantly impact the company's operations and financial performance. The company's success relies on adapting to these dynamic factors. The effectiveness of the company's product development and innovation will be a major driver of their market share and profitability. Strong customer reviews and testimonials can also play a pivotal role in boosting the platform's credibility and attracting new users.
A positive outlook for Augmentum hinges on their ability to secure strong partnerships with financial institutions and other key stakeholders. This can unlock access to a broader customer base, potentially leading to increased user adoption and revenue growth. Strategic acquisitions and mergers, if executed effectively, could provide a significant boost to their product portfolio and geographic reach. The presence of a skilled leadership team capable of navigating market pressures, driving innovation, and effectively managing resources will significantly impact the company's performance. The effectiveness of the company's marketing and sales strategies in penetrating the market and raising brand awareness will also contribute to revenue growth.
Predicting the future financial performance of Augmentum presents both positive and negative risks. A positive outcome would be dependent on a successful rollout of new products, increased customer engagement, and the firm maintaining its competitive edge. However, risks include fierce competition, potential regulatory setbacks, market fluctuations, and changes in consumer behavior that could negatively impact demand for their platform. The company's ability to manage these risks, adapting to evolving market dynamics, and maintaining strong financial discipline will be critical for long-term success. A negative outlook could materialize due to poor financial management, inefficient customer acquisition strategies, or failure to adapt to emerging competitors. Furthermore, unfavorable regulatory environments or unexpected financial crises could severely dampen the company's growth trajectory.
Rating | Short-Term | Long-Term Senior |
---|---|---|
Outlook | B2 | B1 |
Income Statement | B3 | Baa2 |
Balance Sheet | Caa2 | C |
Leverage Ratios | Baa2 | C |
Cash Flow | Ba3 | Baa2 |
Rates of Return and Profitability | B3 | B1 |
*Financial analysis is the process of evaluating a company's financial performance and position by neural network. It involves reviewing the company's financial statements, including the balance sheet, income statement, and cash flow statement, as well as other financial reports and documents.
How does neural network examine financial reports and understand financial state of the company?
References
- Firth JR. 1957. A synopsis of linguistic theory 1930–1955. In Studies in Linguistic Analysis (Special Volume of the Philological Society), ed. JR Firth, pp. 1–32. Oxford, UK: Blackwell
- Rosenbaum PR, Rubin DB. 1983. The central role of the propensity score in observational studies for causal effects. Biometrika 70:41–55
- Jorgenson, D.W., Weitzman, M.L., ZXhang, Y.X., Haxo, Y.M. and Mat, Y.X., 2023. Apple's Stock Price: How News Affects Volatility. AC Investment Research Journal, 220(44).
- S. Proper and K. Tumer. Modeling difference rewards for multiagent learning (extended abstract). In Proceedings of the Eleventh International Joint Conference on Autonomous Agents and Multiagent Systems, Valencia, Spain, June 2012
- Bengio Y, Ducharme R, Vincent P, Janvin C. 2003. A neural probabilistic language model. J. Mach. Learn. Res. 3:1137–55
- M. Ono, M. Pavone, Y. Kuwata, and J. Balaram. Chance-constrained dynamic programming with application to risk-aware robotic space exploration. Autonomous Robots, 39(4):555–571, 2015
- Bessler, D. A. T. Covey (1991), "Cointegration: Some results on U.S. cattle prices," Journal of Futures Markets, 11, 461–474.