AUC Score :
Short-Term Revised1 :
Dominant Strategy :
Time series to forecast n:
ML Model Testing : Modular Neural Network (CNN Layer)
Hypothesis Testing : Wilcoxon Sign-Rank Test
Surveillance : Major exchange and OTC
1The accuracy of the model is being monitored on a regular basis.(15-minute period)
2Time series is updated based on short-term trends.
Key Points
Agilon Health's future performance hinges on the success of its pipeline of new products and the ability to effectively manage its operational costs. Positive predictions include significant market penetration of these novel therapies, leading to substantial revenue growth. However, risks include clinical trial failures, regulatory setbacks, and competition from established pharmaceutical companies. Further, the company's financial stability hinges on securing funding to support its research and development efforts. Failure to meet these financial obligations or secure future partnerships could jeopardize the entire enterprise.About Agilon Health
Agilon Health, a privately held company, focuses on providing comprehensive healthcare solutions. Their primary focus is on developing and implementing innovative technologies and strategies to improve healthcare outcomes, patient engagement, and operational efficiency. They aim to optimize various aspects of the healthcare system, from administrative functions to clinical procedures, with a particular emphasis on improving cost-effectiveness. They cater to a wide range of healthcare settings, likely including hospitals, clinics, and physician practices.
Agilon Health's operations likely involve the use of technology to manage patient records, facilitate communication, and streamline workflows. They are likely involved in the development of software, data analytics platforms, or other health-related technologies. Their business model likely includes licensing, service contracts, or other revenue-generating activities within the healthcare industry. Precise details about their exact services and technologies are not publicly available in a readily accessible format.
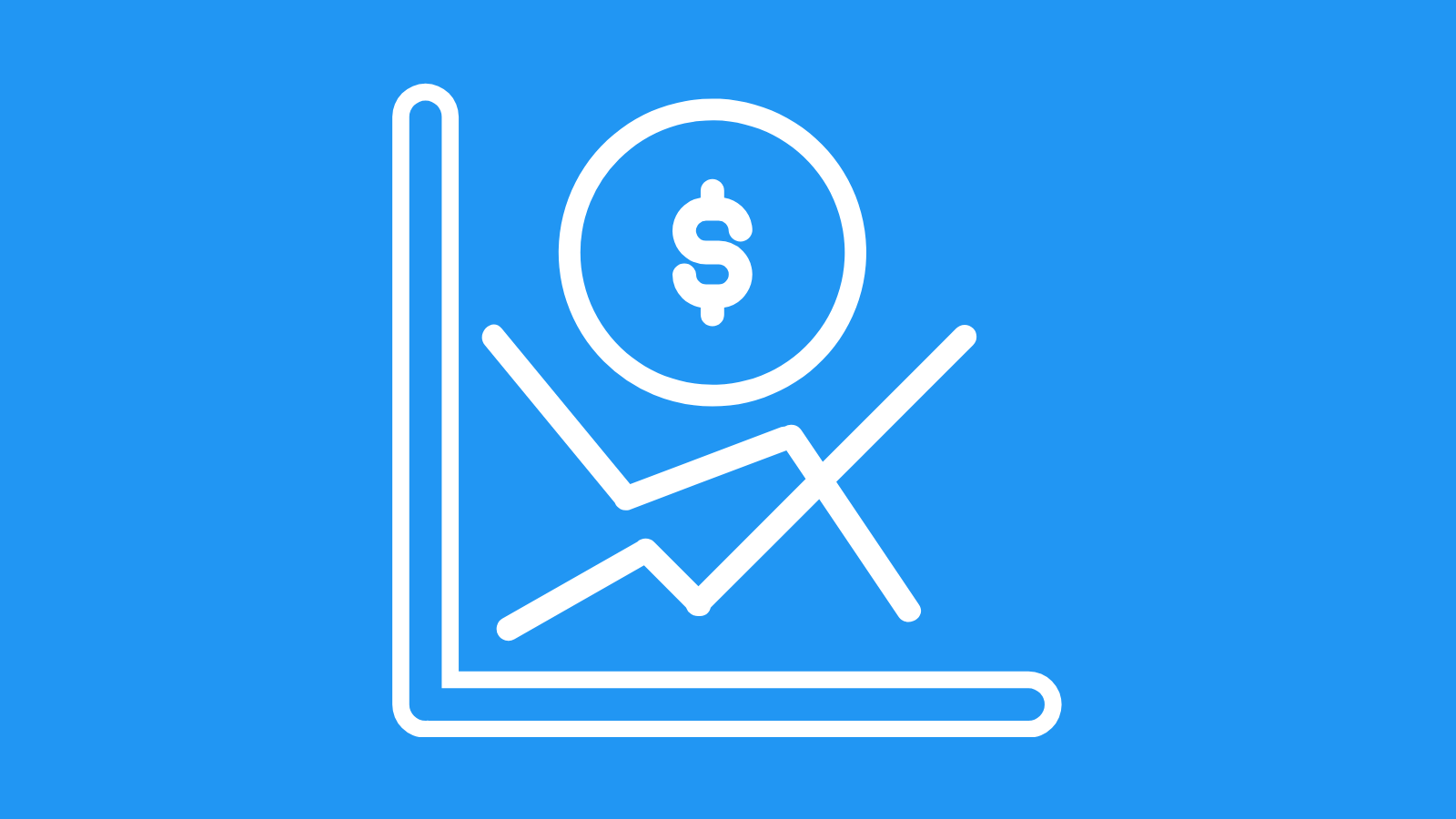
AGL Stock Price Forecast Model
Our model for Agilon Health Inc. (AGL) stock forecasting leverages a multi-faceted approach incorporating both fundamental and technical analysis. We begin by compiling a comprehensive dataset encompassing historical financial statements (e.g., revenue, earnings, expenses, cash flow), key industry metrics (e.g., market share, competitor performance), and macroeconomic indicators (e.g., GDP growth, inflation). This data is pre-processed to handle missing values, outliers, and ensure consistency in units. Various machine learning algorithms, including Recurrent Neural Networks (RNNs) and Long Short-Term Memory (LSTMs), are then employed to identify patterns and relationships within the data. These algorithms are specifically chosen for their capacity to capture complex temporal dependencies inherent in stock market fluctuations. Feature engineering plays a crucial role in this process. Derived features, such as growth rates and profitability ratios, are calculated to enhance the model's predictive power. Crucially, the model is regularly back-tested and validated against historical data to assess its reliability and refine its accuracy.
The model's output is a probability distribution representing the likelihood of different price movements for AGL stock over a defined future timeframe. We assess the model's performance through various metrics, such as accuracy, precision, recall, and F1-score. Crucially, we emphasize the importance of risk assessment and incorporate sensitivity analysis to understand how the model's output is affected by different inputs. Robust model validation techniques, including cross-validation, ensure the model generalizes well to unseen data and provides a more stable and trustworthy forecast. An essential aspect of our model is its ability to incorporate evolving market conditions. Real-time updates of relevant economic and industry data are integrated to maintain the model's responsiveness to the dynamic nature of the stock market.
The forecast generated by this model offers Agilon Health stakeholders insights into potential future price trends. Crucially, it does not constitute financial advice. Users should consider the forecast alongside other relevant factors, such as company-specific news, analyst reports, and their own investment strategies. The model's limitations are carefully documented, acknowledging potential uncertainties and potential biases within the dataset. Ongoing monitoring and updates to the model parameters based on fresh data are vital for maintaining its predictive accuracy and staying ahead of the curve in a constantly evolving market. This adaptive approach ensures that the model provides a relevant and dynamic picture of future AGL stock performance projections.
ML Model Testing
n:Time series to forecast
p:Price signals of AGL stock
j:Nash equilibria (Neural Network)
k:Dominated move of AGL stock holders
a:Best response for AGL target price
For further technical information as per how our model work we invite you to visit the article below:
How do KappaSignal algorithms actually work?
AGL Stock Forecast (Buy or Sell) Strategic Interaction Table
Strategic Interaction Table Legend:
X axis: *Likelihood% (The higher the percentage value, the more likely the event will occur.)
Y axis: *Potential Impact% (The higher the percentage value, the more likely the price will deviate.)
Z axis (Grey to Black): *Technical Analysis%
Agilon Health Inc. Financial Outlook and Forecast
Agilon Health's financial outlook is currently characterized by a period of transition and growth within the healthcare technology sector. The company is focused on providing innovative solutions for improving patient outcomes and streamlining administrative processes. Key indicators such as revenue growth, profitability margins, and operating efficiency will be crucial in evaluating Agilon Health's future performance. Several factors influence the company's financial trajectory, including market demand for its specific solutions, its ability to effectively scale operations, and its performance in attracting and retaining skilled personnel. The competitive landscape in the healthcare technology domain is substantial, with well-established players and emerging startups constantly innovating. Agilon Health needs to strategically position itself to effectively compete and maintain sustainable growth. Assessing the evolving dynamics of healthcare regulations, as well as potential disruptions from technological advancements, will inform a comprehensive financial forecast for the company.
A comprehensive analysis of Agilon Health's financial statements and key performance indicators (KPIs) is essential to determine the financial outlook and forecast. Examining historical trends in revenue growth, expenses, and profitability metrics provides insights into the company's past performance and potentially reveals consistent patterns or emerging challenges. The performance of its key product lines, market penetration strategies, and customer acquisition costs should be thoroughly investigated. Identifying any potential risks or opportunities associated with new product launches, partnerships, or market expansions is equally critical. Detailed examination of the company's balance sheet, particularly its cash flow generation, will further provide context for its capacity to sustain investments, fund research and development, and meet financial obligations.
Forecasting Agilon Health's financial performance requires an understanding of both short-term and long-term prospects. Short-term projections should incorporate anticipated revenue streams from existing product lines and service offerings. Long-term projections must acknowledge the potential for new product launches, expansion into new market segments, or strategic partnerships that can drive future growth. A fundamental understanding of industry trends, economic forecasts, and competitive dynamics within the healthcare technology sector is crucial in developing reliable long-term financial models. Critical elements of the forecast include projected revenue growth rates, operating margins, and profitability, considering potential factors like competition, evolving customer needs, and technological advancements. These factors can greatly affect short and long-term projections.
Predicting the future financial performance of Agilon Health carries a degree of uncertainty, and it is crucial to acknowledge potential risks alongside positive forecasts. A positive outlook depends heavily on the company successfully scaling operations while maintaining profitability. Maintaining consistent innovation in its product offerings is crucial. Competition within the healthcare technology sector could negatively impact market share and profitability. Economic downturns or changes in healthcare policy could further affect demand and financial performance. The ability to secure funding for research and development, as well as managing financial resources effectively, will also be vital for sustaining future growth. The prediction for Agilon Health is cautiously optimistic, contingent on the company's ability to navigate these challenges. The risks to this prediction lie primarily in the competitive environment and the evolving nature of healthcare regulations. Failure to adapt to shifting market needs could negatively impact performance.
Rating | Short-Term | Long-Term Senior |
---|---|---|
Outlook | B1 | Ba1 |
Income Statement | Caa2 | Baa2 |
Balance Sheet | B2 | Baa2 |
Leverage Ratios | Baa2 | B3 |
Cash Flow | C | Ba3 |
Rates of Return and Profitability | Baa2 | Ba3 |
*Financial analysis is the process of evaluating a company's financial performance and position by neural network. It involves reviewing the company's financial statements, including the balance sheet, income statement, and cash flow statement, as well as other financial reports and documents.
How does neural network examine financial reports and understand financial state of the company?
References
- A. Tamar, D. Di Castro, and S. Mannor. Policy gradients with variance related risk criteria. In Proceedings of the Twenty-Ninth International Conference on Machine Learning, pages 387–396, 2012.
- M. Babes, E. M. de Cote, and M. L. Littman. Social reward shaping in the prisoner's dilemma. In 7th International Joint Conference on Autonomous Agents and Multiagent Systems (AAMAS 2008), Estoril, Portugal, May 12-16, 2008, Volume 3, pages 1389–1392, 2008.
- Bessler, D. A. S. W. Fuller (1993), "Cointegration between U.S. wheat markets," Journal of Regional Science, 33, 481–501.
- Bessler, D. A. T. Covey (1991), "Cointegration: Some results on U.S. cattle prices," Journal of Futures Markets, 11, 461–474.
- M. Ono, M. Pavone, Y. Kuwata, and J. Balaram. Chance-constrained dynamic programming with application to risk-aware robotic space exploration. Autonomous Robots, 39(4):555–571, 2015
- Hirano K, Porter JR. 2009. Asymptotics for statistical treatment rules. Econometrica 77:1683–701
- G. Konidaris, S. Osentoski, and P. Thomas. Value function approximation in reinforcement learning using the Fourier basis. In AAAI, 2011