AUC Score :
Short-Term Revised1 :
Dominant Strategy :
Time series to forecast n:
ML Model Testing : Transductive Learning (ML)
Hypothesis Testing : ElasticNet Regression
Surveillance : Major exchange and OTC
1The accuracy of the model is being monitored on a regular basis.(15-minute period)
2Time series is updated based on short-term trends.
Key Points
This exclusive content is only available to premium users.About PennyMac Financial
PennyMac is a prominent mortgage real estate investment trust (REIT) operating primarily in the US. The company's core business involves the acquisition, origination, and servicing of mortgage loans. PennyMac is involved in various aspects of the mortgage lifecycle, including purchasing mortgages from other lenders, originating new loans directly to borrowers, and providing ongoing servicing for these loans. This integrated approach allows them to manage risk effectively and capitalize on market opportunities across the mortgage spectrum. They offer a range of products catering to both individual borrowers and institutional investors.
PennyMac's operations are heavily influenced by prevailing interest rates and broader economic conditions impacting the housing market. The company employs sophisticated technology and data analytics to manage its portfolio and optimize its operations. They are a significant player in the secondary mortgage market, contributing to the liquidity and efficiency of the overall mortgage finance system. PennyMac maintains a focus on compliance and regulatory adherence within the financial services sector. Their performance is tied to several factors including the volume of mortgage originations, the performance of their servicing portfolio, and the prevailing conditions in the broader financial markets.
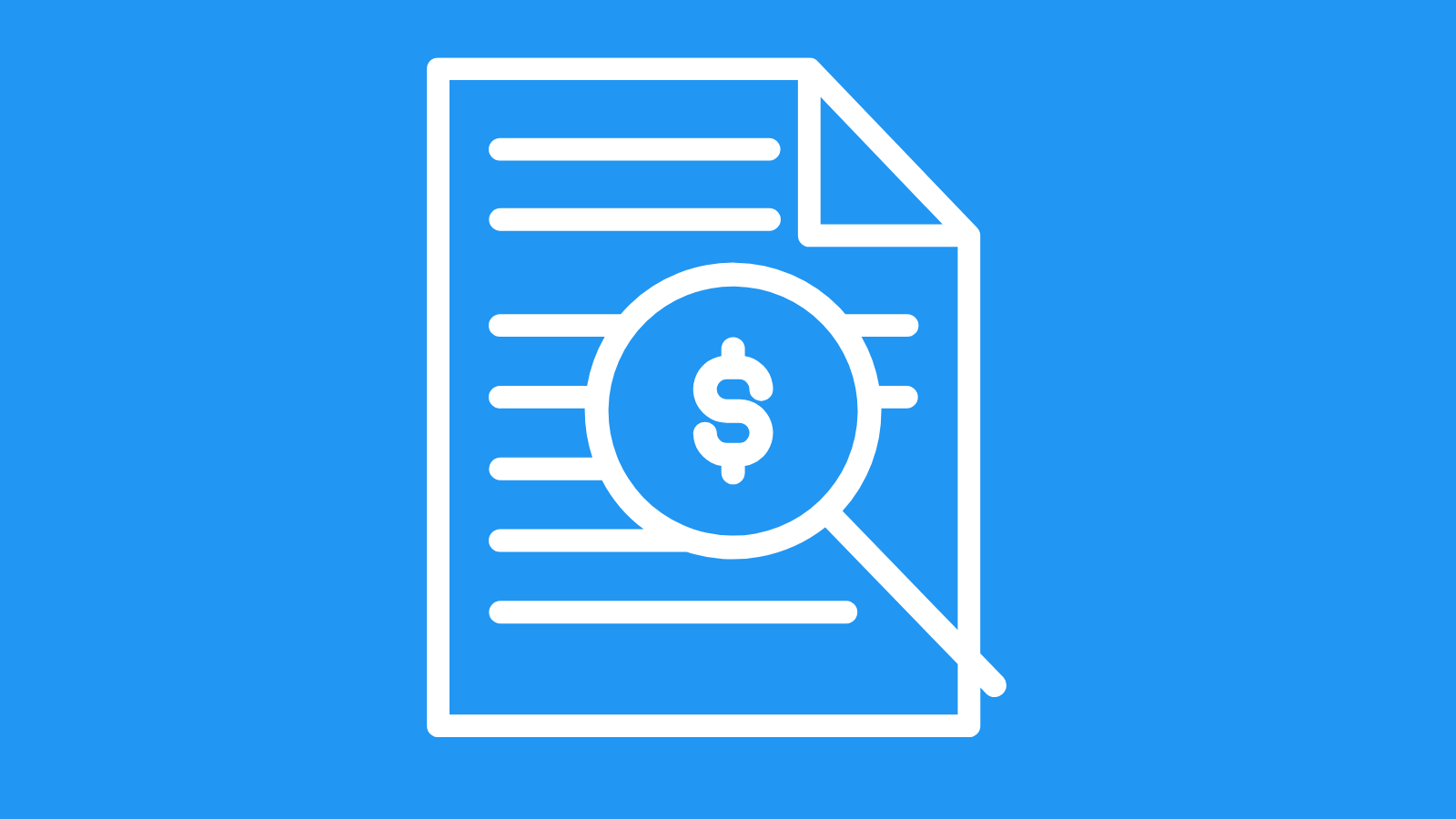
Predicting PennyMac's Financial Trajectory: A Machine Learning Approach
Our team of data scientists and economists has developed a sophisticated machine learning model to forecast the future performance of PennyMac Financial Services Inc. Common Stock (PFSI). The model leverages a diverse range of predictor variables, encompassing both macroeconomic indicators and firm-specific financial data. Macroeconomic factors include inflation rates, interest rate movements, GDP growth, and unemployment figures, all of which significantly impact the mortgage finance sector. Firm-specific data includes PennyMac's quarterly earnings reports, balance sheet information, key performance indicators such as loan origination volume and servicing portfolio size, as well as relevant regulatory changes impacting the mortgage industry. We employ a hybrid approach, combining time-series analysis techniques like ARIMA and LSTM neural networks with regression models to capture both the temporal dependencies inherent in financial data and the complex relationships between predictor variables and PFSI's stock performance. Feature engineering plays a crucial role, with particular attention paid to creating lagged variables and interaction terms to capture dynamic relationships and non-linear effects. Model selection and hyperparameter tuning are rigorously conducted using techniques like cross-validation and grid search, ensuring robust generalization performance.
The core of our predictive model relies on a Long Short-Term Memory (LSTM) recurrent neural network. LSTMs are particularly well-suited for handling sequential data such as financial time series, effectively capturing long-term dependencies that are often missed by simpler models. The LSTM network receives as input a multi-dimensional time series encompassing both macroeconomic and firm-specific variables, processed and scaled using appropriate normalization techniques. The output layer of the LSTM produces a forecast of future PFSI stock performance, expressed as a continuous variable representing expected return or a classification indicating potential directional movement (e.g., up, down, or sideways). To enhance robustness and reduce overfitting, we incorporate regularization techniques into the LSTM architecture, preventing the model from overly relying on specific features or noise in the training data. The model is further validated and refined using a comprehensive backtesting strategy on historical data, ensuring its predictive capability under diverse market conditions.
Beyond the core predictive model, our analysis incorporates a comprehensive risk assessment framework. We evaluate model uncertainty through techniques like bootstrapping and quantile regression, generating confidence intervals around the predictions. This ensures a transparent and nuanced understanding of the inherent uncertainty associated with any financial forecast. Furthermore, we actively monitor the model's performance over time and retrain it periodically using updated data and advancements in machine learning techniques, mitigating the risk of model degradation. Our continuous monitoring and model refinement are crucial in maintaining the accuracy and reliability of our PFSI stock prediction capabilities in the dynamic environment of the financial markets. The final output is a probability distribution of possible future outcomes, allowing for informed decision-making that incorporates the inherent risks involved in financial market predictions.
ML Model Testing
n:Time series to forecast
p:Price signals of PFSI stock
j:Nash equilibria (Neural Network)
k:Dominated move of PFSI stock holders
a:Best response for PFSI target price
For further technical information as per how our model work we invite you to visit the article below:
How do KappaSignal algorithms actually work?
PFSI Stock Forecast (Buy or Sell) Strategic Interaction Table
Strategic Interaction Table Legend:
X axis: *Likelihood% (The higher the percentage value, the more likely the event will occur.)
Y axis: *Potential Impact% (The higher the percentage value, the more likely the price will deviate.)
Z axis (Grey to Black): *Technical Analysis%
Rating | Short-Term | Long-Term Senior |
---|---|---|
Outlook | B1 | B1 |
Income Statement | C | Baa2 |
Balance Sheet | B1 | Caa2 |
Leverage Ratios | Baa2 | B3 |
Cash Flow | Baa2 | C |
Rates of Return and Profitability | Caa2 | Baa2 |
*Financial analysis is the process of evaluating a company's financial performance and position by neural network. It involves reviewing the company's financial statements, including the balance sheet, income statement, and cash flow statement, as well as other financial reports and documents.
How does neural network examine financial reports and understand financial state of the company?This exclusive content is only available to premium users.This exclusive content is only available to premium users.
PennyMac: Prospects for Enhanced Operating Efficiency
PennyMac's operating efficiency is a key driver of its profitability and overall financial health. The company's business model, centered around the servicing and origination of mortgages, necessitates a high degree of operational effectiveness to manage the large volume of transactions and data involved. Key aspects impacting PennyMac's operational efficiency include its technology infrastructure, automation capabilities, and the efficiency of its workforce. Investments in technology, such as advanced analytics platforms and automation tools for loan processing and servicing, are critical for streamlining workflows and reducing operational costs. Furthermore, PennyMac's ability to effectively manage its workforce, including the retention and training of skilled employees, directly contributes to its efficiency. A well-trained and efficient workforce translates to reduced error rates, faster turnaround times, and ultimately, improved operational performance.
Looking ahead, PennyMac's focus on technology modernization will likely play a significant role in shaping its future operating efficiency. The company is expected to continue investing in its technological infrastructure to enhance automation, improve data analytics capabilities, and reduce manual processes. This strategic investment should lead to significant gains in efficiency, particularly within its mortgage servicing operations where high volumes and repetitive tasks are prevalent. Moreover, PennyMac's ability to leverage data analytics to identify and mitigate operational bottlenecks, optimize resource allocation, and improve risk management will be crucial for sustaining improvements in operating efficiency. The extent of these improvements will depend on the success of these technology investments and the company's ability to effectively integrate them into its operational workflows.
Beyond technological advancements, PennyMac's operational efficiency is also contingent upon effective risk management and regulatory compliance. The mortgage industry is heavily regulated, and non-compliance can lead to significant operational disruptions and financial penalties. PennyMac's ability to efficiently navigate the regulatory landscape and maintain a strong compliance program is integral to its operational efficiency. Moreover, robust risk management practices, including effective fraud detection and prevention mechanisms, are crucial for minimizing operational losses and maintaining stability. Failure to adequately address regulatory requirements and manage risk could negatively impact the company's overall efficiency and profitability, potentially offsetting gains from technological investments.
In conclusion, PennyMac's future operating efficiency is projected to be significantly influenced by strategic technology investments, effective risk management, and regulatory compliance. While investments in automation and data analytics are expected to drive improvements, successful implementation and integration are crucial for realizing these potential benefits. Sustained focus on workforce training and retention, coupled with robust compliance and risk management programs, will further solidify PennyMac's operational efficiency and contribute to its long-term financial success. The company's success in these areas will be a key indicator of its competitiveness within the mortgage industry.
PennyMac: A Risk Assessment of its Common Stock
PennyMac's (PFSI) common stock carries inherent risks stemming primarily from its exposure to the cyclical nature of the mortgage industry. Interest rate fluctuations significantly impact refinancing activity, a crucial driver of PennyMac's revenue. Rising rates typically lead to reduced refinancing volume, impacting origination fees and servicing income, while falling rates can increase competition and potentially compress margins. Furthermore, the company's performance is sensitive to changes in the overall macroeconomic environment. Economic downturns, increased unemployment, and a weakening housing market can all lead to higher delinquencies and defaults on mortgages in PennyMac's portfolio, thereby negatively impacting its profitability and potentially leading to credit losses. Regulatory changes within the financial services sector also pose a considerable risk, as new rules and compliance costs could affect operating efficiency and profitability.
Credit risk represents a significant concern for PennyMac. As a major player in mortgage origination and servicing, the company holds a substantial portfolio of mortgages, which are subject to default risk. The quality of these mortgages, influenced by factors such as borrower creditworthiness and the prevailing economic climate, directly affects PennyMac's profitability. A rise in delinquencies or defaults could lead to significant losses, requiring increased loan loss provisions and potentially impacting the company's financial stability. Careful underwriting standards and robust risk management practices are crucial for mitigating this risk, but external factors outside PennyMac's control can still impact its credit portfolio performance. Effective risk mitigation strategies include diversification of loan types and geographic locations, as well as employing sophisticated credit scoring models.
Operational risks associated with technology failures, cybersecurity breaches, and human error also need to be considered. PennyMac relies heavily on technology for its operations, encompassing mortgage origination, servicing, and data management. Any disruption to its technological infrastructure can lead to significant operational disruptions, resulting in financial losses and reputational damage. Cybersecurity threats are increasingly prevalent in the financial services industry, and a successful attack could expose sensitive customer data and potentially lead to regulatory penalties. Internal controls and robust business continuity plans are essential for mitigating these risks. Continuous investments in technology upgrades and cybersecurity measures are also crucial for maintaining operational resilience and safeguarding against potential losses.
In summary, investing in PennyMac's common stock presents a multifaceted risk profile. While the company benefits from its strong position in the mortgage market and its diverse business model, it is highly sensitive to macroeconomic conditions, interest rate changes, and credit risk. Potential investors should carefully assess their own risk tolerance and thoroughly evaluate PennyMac's financial statements, risk management strategies, and industry outlook before making any investment decisions. A comprehensive understanding of these risks, coupled with diligent due diligence, is crucial for informed investment decisions in this sector.
References
- Wan M, Wang D, Goldman M, Taddy M, Rao J, et al. 2017. Modeling consumer preferences and price sensitiv- ities from large-scale grocery shopping transaction logs. In Proceedings of the 26th International Conference on the World Wide Web, pp. 1103–12. New York: ACM
- Hastie T, Tibshirani R, Wainwright M. 2015. Statistical Learning with Sparsity: The Lasso and Generalizations. New York: CRC Press
- Angrist JD, Pischke JS. 2008. Mostly Harmless Econometrics: An Empiricist's Companion. Princeton, NJ: Princeton Univ. Press
- Athey S, Imbens GW. 2017b. The state of applied econometrics: causality and policy evaluation. J. Econ. Perspect. 31:3–32
- Vilnis L, McCallum A. 2015. Word representations via Gaussian embedding. arXiv:1412.6623 [cs.CL]
- Jorgenson, D.W., Weitzman, M.L., ZXhang, Y.X., Haxo, Y.M. and Mat, Y.X., 2023. Tesla Stock: Hold for Now, But Watch for Opportunities. AC Investment Research Journal, 220(44).
- Farrell MH, Liang T, Misra S. 2018. Deep neural networks for estimation and inference: application to causal effects and other semiparametric estimands. arXiv:1809.09953 [econ.EM]