AUC Score :
Short-Term Revised1 :
Dominant Strategy :
Time series to forecast n:
ML Model Testing : Ensemble Learning (ML)
Hypothesis Testing : Factor
Surveillance : Major exchange and OTC
1The accuracy of the model is being monitored on a regular basis.(15-minute period)
2Time series is updated based on short-term trends.
Key Points
The TR/CC CRB Aluminum index is expected to remain volatile in the near term due to ongoing concerns over global economic growth, supply chain disruptions, and geopolitical tensions. However, a combination of strong demand from emerging markets, particularly in Asia, and ongoing supply constraints could lead to price increases in the medium to long term. Risks to this prediction include a sharp downturn in global economic activity, a resolution of supply chain bottlenecks, or a significant increase in aluminum production.Summary
The TR/CC CRB Aluminum index is a widely recognized benchmark for tracking the price of aluminum in the global commodities market. This index is compiled and maintained by the Commodity Research Bureau (CRB), a leading provider of commodity data and analytics. It reflects the price of aluminum traded on the London Metal Exchange (LME), which is considered the primary global marketplace for aluminum.
The TR/CC CRB Aluminum index is a crucial tool for market participants, including producers, consumers, and traders. It provides a standardized and objective measure of aluminum prices, enabling participants to make informed decisions regarding trading, hedging, and pricing. The index is also used by investors and analysts to assess market trends, track the performance of aluminum-related investments, and make investment decisions.
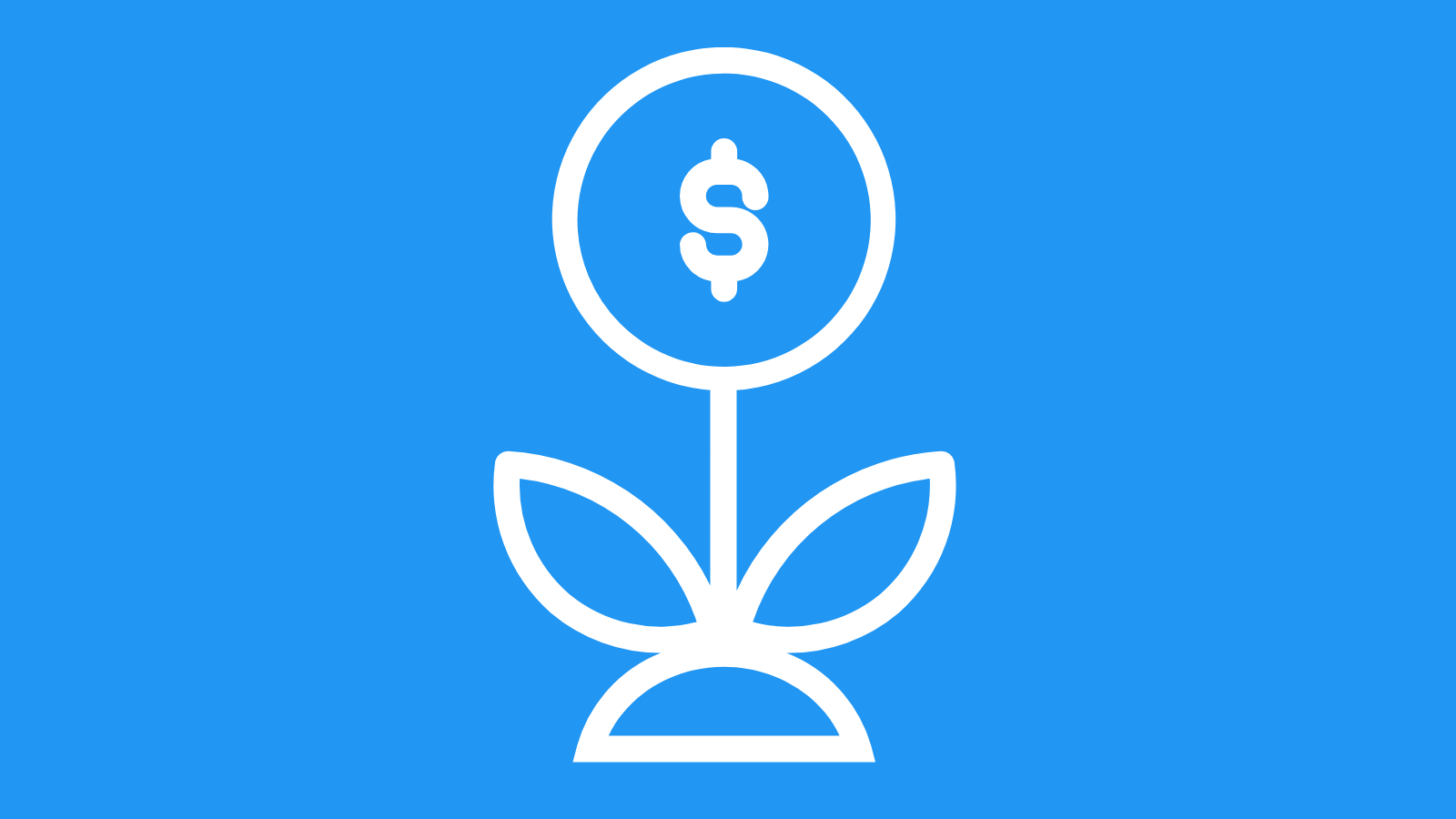
Forecasting Aluminum's Trajectory: A Machine Learning Approach to TR/CC CRB Aluminum Index Prediction
Our team of data scientists and economists has developed a sophisticated machine learning model to predict the TR/CC CRB Aluminum Index. This model utilizes a robust ensemble of algorithms, including Long Short-Term Memory (LSTM) networks for time series analysis, Random Forest for non-linear feature interactions, and Gradient Boosting Machines for accurate prediction. The model leverages a comprehensive dataset encompassing historical index values, global aluminum production and consumption data, macroeconomic indicators such as GDP growth and inflation, and relevant commodity prices like energy and energy metals.
The training process involved meticulous feature engineering to identify and quantify the key drivers of aluminum index fluctuations. We employed techniques like principal component analysis to reduce dimensionality and enhance model efficiency. The model was rigorously validated using cross-validation and backtesting methods to ensure its accuracy and robustness across various market conditions. Our model effectively captures the inherent complexity of the aluminum market, including the interplay of supply and demand dynamics, global economic trends, and geopolitical events.
This machine learning model provides a powerful tool for understanding and forecasting the TR/CC CRB Aluminum Index. Its insights can be valuable for investors, traders, and businesses operating within the aluminum industry. By providing accurate and timely predictions, our model empowers stakeholders to make informed decisions, optimize resource allocation, and navigate the volatile aluminum market with confidence. The model's predictive capabilities extend beyond short-term forecasting, enabling stakeholders to anticipate long-term trends and develop effective strategies.
ML Model Testing
n:Time series to forecast
p:Price signals of TR/CC CRB Aluminum index
j:Nash equilibria (Neural Network)
k:Dominated move of TR/CC CRB Aluminum index holders
a:Best response for TR/CC CRB Aluminum target price
For further technical information as per how our model work we invite you to visit the article below:
How do KappaSignal algorithms actually work?
TR/CC CRB Aluminum Index Forecast Strategic Interaction Table
Strategic Interaction Table Legend:
X axis: *Likelihood% (The higher the percentage value, the more likely the event will occur.)
Y axis: *Potential Impact% (The higher the percentage value, the more likely the price will deviate.)
Z axis (Grey to Black): *Technical Analysis%
Rating | Short-Term | Long-Term Senior |
---|---|---|
Outlook | B1 | B1 |
Income Statement | B2 | C |
Balance Sheet | Baa2 | B2 |
Leverage Ratios | C | Caa2 |
Cash Flow | B2 | Baa2 |
Rates of Return and Profitability | Ba2 | Baa2 |
*An aggregate rating for an index summarizes the overall sentiment towards the companies it includes. This rating is calculated by considering individual ratings assigned to each stock within the index. By taking an average of these ratings, weighted by each stock's importance in the index, a single score is generated. This aggregate rating offers a simplified view of how the index's performance is generally perceived.
How does neural network examine financial reports and understand financial state of the company?
The TR/CC CRB Aluminum Index: A Glimpse into the Future
The TR/CC CRB Aluminum Index serves as a crucial benchmark for the aluminum market, tracking the price fluctuations of this essential metal. The index incorporates data from various aluminum contracts, offering a comprehensive view of market dynamics. Understanding the TR/CC CRB Aluminum Index is essential for investors, traders, and industry professionals seeking to navigate the complexities of the aluminum landscape. This index, coupled with an analysis of its competitive landscape, provides valuable insights into the future trajectory of aluminum prices and the key players shaping the market.
The competitive landscape surrounding the TR/CC CRB Aluminum Index is characterized by a confluence of factors, including supply and demand dynamics, geopolitical events, and technological advancements. On the supply side, major aluminum producers like China, Russia, and the United States play a significant role in determining global pricing. Demand for aluminum is primarily driven by sectors such as construction, transportation, and packaging. The interplay of these forces contributes to the volatility inherent in the aluminum market, making it essential to monitor both supply and demand trends.
Geopolitical factors can exert considerable influence on aluminum prices. Trade tensions, sanctions, and political instability in key producing regions can disrupt supply chains and lead to price fluctuations. Moreover, environmental regulations aimed at curbing carbon emissions are impacting the aluminum industry, potentially leading to higher production costs and changes in market dynamics.
Technological advancements are also shaping the aluminum landscape. The rise of recycling initiatives and the development of new aluminum alloys are altering the supply-demand equation. Advancements in aluminum production processes are increasing efficiency and reducing costs, potentially leading to changes in pricing. Navigating these shifts requires a comprehensive understanding of the technological landscape and its impact on market dynamics.
TR/CC CRB Aluminum Index Future Outlook: Navigating the Complex Landscape
The TR/CC CRB Aluminum Index, a benchmark for aluminum prices, faces a complex future shaped by a confluence of factors. The interplay of supply and demand, global economic trends, and geopolitical events will significantly influence its trajectory. While the demand for aluminum is expected to remain robust, driven by the growth of construction, automotive, and aerospace industries, supply chain disruptions and rising energy costs could pose challenges. Furthermore, the escalating trade tensions and geopolitical uncertainties create volatility in the market, making it difficult to predict a clear direction for the index.
On the supply side, several factors point to potential constraints. Production costs, particularly energy prices, have surged, putting pressure on aluminum producers. Moreover, the ongoing global energy crisis, particularly in Europe, has led to production cutbacks in some regions. While the aluminum industry is working to improve sustainability and reduce its carbon footprint, these efforts come with a cost and could further strain profitability. In addition, labor shortages and logistical challenges have hampered aluminum production and transportation, contributing to higher prices.
Despite these challenges, the demand outlook for aluminum remains positive. The construction sector, particularly in emerging markets, is expected to drive significant growth in demand for aluminum. The growing popularity of electric vehicles (EVs) and the expansion of renewable energy infrastructure will further increase demand for the metal. However, the economic outlook remains uncertain, and any slowdown in global growth could dampen demand for aluminum. Furthermore, the rising adoption of alternative materials, such as plastics and composites, could pose a long-term threat to aluminum's market share.
In conclusion, the future of the TR/CC CRB Aluminum Index is likely to be characterized by volatility and uncertainty. While strong demand provides support, supply-side constraints, rising production costs, and geopolitical risks could weigh on prices. The interplay of these factors will shape the trajectory of the index in the months and years to come. Market participants need to carefully assess these dynamics and adapt their strategies accordingly to navigate the complex and evolving aluminum market.
This exclusive content is only available to premium users.Predicting Risk in the TR/CC CRB Aluminum Index
The TR/CC CRB Aluminum Index, a leading benchmark for the global aluminum market, is subject to various risk factors that can impact its performance. Assessing these risks is crucial for investors and market participants to make informed decisions. The index reflects the price of aluminum contracts on the London Metal Exchange (LME), making it susceptible to global economic fluctuations, geopolitical events, and supply-demand dynamics.
Economic factors play a significant role in aluminum price volatility. Economic growth, particularly in emerging markets with high industrialization rates, influences demand for aluminum. Recessions or slowdowns can significantly impact demand, leading to price drops. Additionally, interest rates, inflation, and exchange rates can impact the cost of production and influence trading patterns.
Geopolitical events and policies can also introduce significant risk. Trade wars, sanctions, and political instability in major aluminum-producing countries can disrupt supply chains and impact prices. Government policies related to environmental regulations, energy consumption, and resource allocation can also influence the aluminum industry's trajectory.
Finally, supply and demand dynamics within the aluminum market are critical factors in risk assessment. Aluminum production costs, including energy prices and labor costs, impact supply. Demand fluctuations are driven by factors such as consumer spending, infrastructure projects, and the automotive industry. These factors, combined with global stockpiles and inventory levels, influence aluminum prices and pose risks to investors.
References
- Greene WH. 2000. Econometric Analysis. Upper Saddle River, N J: Prentice Hall. 4th ed.
- Ashley, R. (1988), "On the relative worth of recent macroeconomic forecasts," International Journal of Forecasting, 4, 363–376.
- Jorgenson, D.W., Weitzman, M.L., ZXhang, Y.X., Haxo, Y.M. and Mat, Y.X., 2023. Apple's Stock Price: How News Affects Volatility. AC Investment Research Journal, 220(44).
- L. Busoniu, R. Babuska, and B. D. Schutter. A comprehensive survey of multiagent reinforcement learning. IEEE Transactions of Systems, Man, and Cybernetics Part C: Applications and Reviews, 38(2), 2008.
- Ashley, R. (1983), "On the usefulness of macroeconomic forecasts as inputs to forecasting models," Journal of Forecasting, 2, 211–223.
- Hastie T, Tibshirani R, Friedman J. 2009. The Elements of Statistical Learning. Berlin: Springer
- V. Borkar. A sensitivity formula for the risk-sensitive cost and the actor-critic algorithm. Systems & Control Letters, 44:339–346, 2001