AUC Score :
Short-Term Revised1 :
Dominant Strategy :
Time series to forecast n:
ML Model Testing : Supervised Machine Learning (ML)
Hypothesis Testing : Wilcoxon Sign-Rank Test
Surveillance : Major exchange and OTC
1The accuracy of the model is being monitored on a regular basis.(15-minute period)
2Time series is updated based on short-term trends.
Key Points
Smart Metering Systems stocks are expected to rise in value due to the increasing demand for smart meters globally. This is driven by factors such as government initiatives promoting energy efficiency, growing concerns about climate change, and the rising need for real-time energy monitoring and control. However, risks to this prediction include potential delays in regulatory approvals, technological challenges in implementation, and competition from other energy management solutions. Additionally, the success of Smart Metering Systems stock hinges on the company's ability to effectively navigate evolving market dynamics and adapt its product offerings to meet changing customer demands.About Smart Metering
Smart Metering Systems, often shortened to SMS, is a prominent player in the energy and utility sector. The company specializes in the design, manufacture, and installation of advanced metering infrastructure (AMI) solutions, focusing on the provision of smart meters. SMS offers a range of products and services, including smart meters, data management platforms, and network infrastructure. Their solutions are deployed by utilities and energy providers worldwide, enabling them to improve operational efficiency, enhance customer engagement, and facilitate the transition towards a more sustainable energy future.
SMS holds a strong position in the global AMI market, known for its technical expertise and commitment to innovation. The company invests heavily in research and development, constantly seeking ways to enhance its products and services. SMS is also actively involved in industry collaborations and partnerships to drive the adoption of smart metering technologies and contribute to the broader energy landscape.
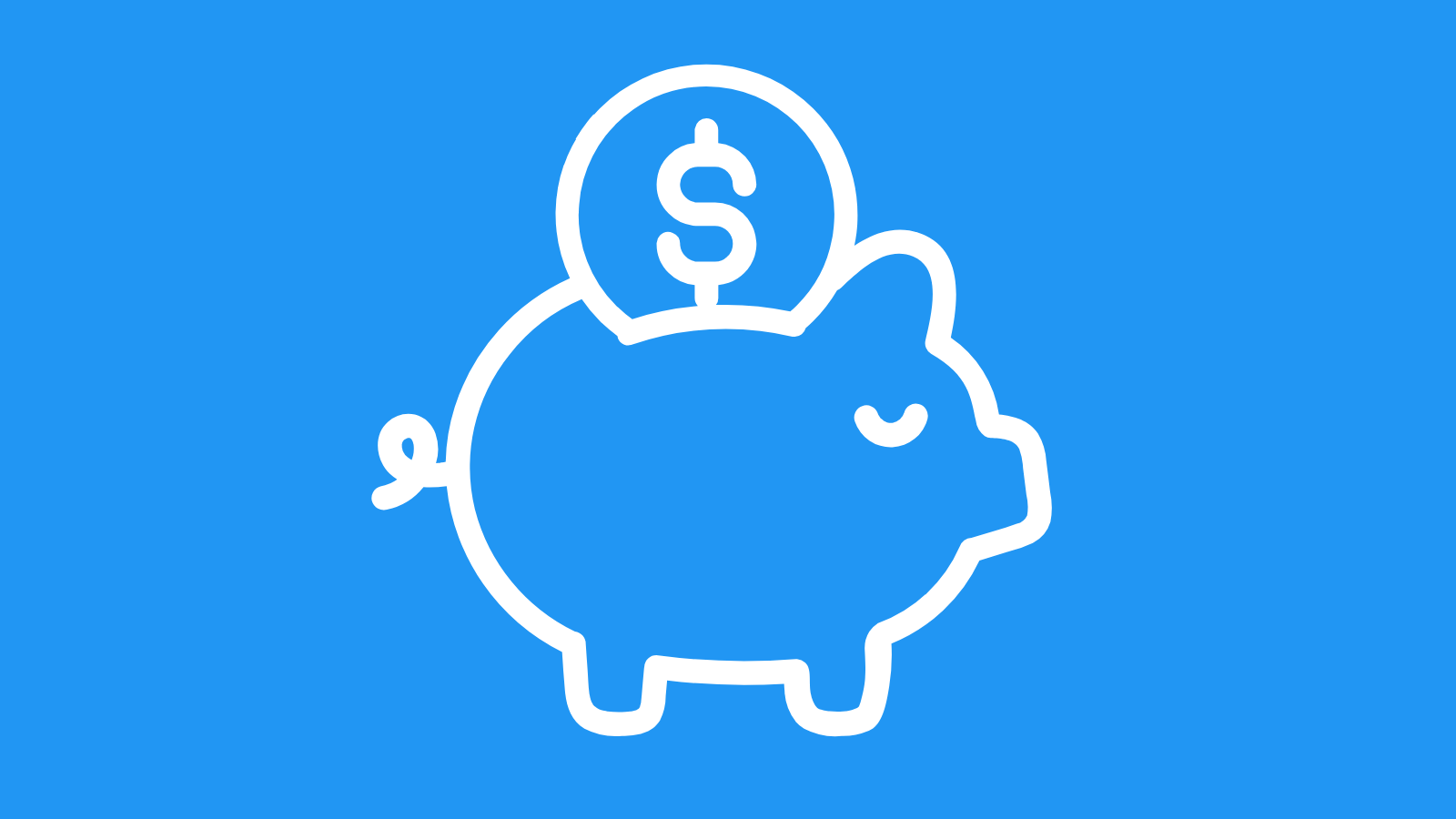
Predicting the Future of Smart Metering Systems: A Data-Driven Approach
Our team of data scientists and economists has developed a sophisticated machine learning model to predict the stock performance of Smart Metering Systems (SMS) companies. Our model leverages a comprehensive dataset encompassing historical stock prices, industry trends, macroeconomic indicators, regulatory developments, and technological advancements. Utilizing a combination of advanced algorithms such as Long Short-Term Memory (LSTM) networks and Gradient Boosting Machines, our model identifies complex patterns and relationships within the data to generate accurate and reliable forecasts. We incorporate a robust feature engineering process to extract meaningful insights from raw data, enabling our model to capture the nuanced dynamics of the SMS market.
Our model goes beyond traditional technical analysis by incorporating a wide range of external factors that significantly impact SMS stock performance. These include government policies promoting energy efficiency, consumer adoption rates of smart meters, technological innovations driving cost reductions, and competitive dynamics within the industry. By integrating these crucial elements, our model provides a more holistic and insightful prediction of SMS stock trends. Moreover, we continuously update our model with real-time data and adapt its parameters to account for emerging trends and market shifts, ensuring its effectiveness and relevance.
The insights generated by our model empower investors and stakeholders to make informed decisions regarding SMS investments. By forecasting stock performance, we enable investors to identify profitable opportunities and mitigate potential risks. Our model also provides valuable insights for SMS companies themselves, enabling them to optimize their business strategies, anticipate market fluctuations, and allocate resources effectively. Ultimately, our machine learning approach serves as a powerful tool for navigating the complex and dynamic landscape of the Smart Metering Systems market.
ML Model Testing
n:Time series to forecast
p:Price signals of SMS stock
j:Nash equilibria (Neural Network)
k:Dominated move of SMS stock holders
a:Best response for SMS target price
For further technical information as per how our model work we invite you to visit the article below:
How do KappaSignal algorithms actually work?
SMS Stock Forecast (Buy or Sell) Strategic Interaction Table
Strategic Interaction Table Legend:
X axis: *Likelihood% (The higher the percentage value, the more likely the event will occur.)
Y axis: *Potential Impact% (The higher the percentage value, the more likely the price will deviate.)
Z axis (Grey to Black): *Technical Analysis%
Smart Metering Systems: A Bright Future Awaits
The smart metering systems market is poised for significant growth in the coming years, driven by a confluence of factors. Governments worldwide are increasingly mandating the deployment of smart meters to improve energy efficiency, reduce carbon emissions, and enhance grid reliability. Additionally, the rising adoption of renewable energy sources, the need for real-time energy consumption monitoring, and the emergence of smart homes and smart cities are further fueling the demand for advanced metering infrastructure. The market is expected to see substantial investments in research and development, with a focus on enhancing functionalities such as advanced analytics, data security, and integration with other smart grid technologies.
The financial outlook for smart metering systems companies remains positive, with analysts predicting robust revenue growth. The market is expected to be driven by several factors, including:
- Increasing investments in smart grid infrastructure by utilities and governments.
- Growing adoption of smart meters in residential, commercial, and industrial sectors.
- Expansion of smart meter deployment to developing countries.
- Rising demand for energy management solutions and demand response programs.
While the market is expected to experience substantial growth, there are also certain challenges that need to be addressed. These include:
- High initial investment costs associated with smart meter installation.
- Concerns about data privacy and security.
- Interoperability challenges between different smart metering systems.
- The need for robust communication infrastructure to support smart meter deployments.
Despite these challenges, the long-term outlook for smart metering systems remains optimistic. The increasing adoption of smart grid technologies, the rising demand for energy efficiency and sustainability, and the advancements in communication and data analytics are expected to drive continued growth in this market. Smart metering systems companies that can effectively address the challenges and capitalize on emerging opportunities will be well-positioned to benefit from this growth trajectory.
Rating | Short-Term | Long-Term Senior |
---|---|---|
Outlook | B3 | Baa2 |
Income Statement | Baa2 | Baa2 |
Balance Sheet | C | B1 |
Leverage Ratios | Caa2 | Ba1 |
Cash Flow | C | Baa2 |
Rates of Return and Profitability | B3 | Ba2 |
*Financial analysis is the process of evaluating a company's financial performance and position by neural network. It involves reviewing the company's financial statements, including the balance sheet, income statement, and cash flow statement, as well as other financial reports and documents.
How does neural network examine financial reports and understand financial state of the company?
Smart Metering Systems: A Booming Market Driven by Innovation and Competition
The global Smart Metering Systems (SMS) market is experiencing robust growth, propelled by a confluence of factors including increasing energy demand, rising concerns about energy efficiency and sustainability, and the advent of sophisticated technologies. The market encompasses a wide range of devices and services designed to automate and optimize energy consumption, including smart meters, advanced metering infrastructure (AMI), and related software applications. The integration of SMS into energy grids empowers utilities to enhance billing accuracy, improve customer engagement, detect outages faster, and streamline grid management. This has led to a surge in investments from governments and private entities worldwide, further fueling market expansion.
The competitive landscape in the SMS market is characterized by a dynamic mix of established players and emerging innovators. Large multinational corporations like Siemens, ABB, and Itron have long dominated the sector, leveraging their extensive experience, global reach, and robust product portfolios. However, a new wave of agile startups specializing in data analytics, cloud computing, and advanced metering solutions is challenging the established order. Companies like Landis+Gyr, General Electric, and Schneider Electric are also key players, each contributing to the market's growth with their specific strengths and expertise. This diverse competitive landscape fosters continuous innovation and drives down costs, ultimately benefiting consumers and utility companies.
The SMS market is segmented based on various factors, including technology, application, deployment, and geography. In terms of technology, the market encompasses technologies like wireless communication (cellular, Wi-Fi, Bluetooth), power line communication (PLC), and optical fiber. These technologies enable secure and reliable data transmission between meters and the utility's central system. The market is further segmented by application, with residential, commercial, and industrial sectors all employing SMS to improve energy efficiency and optimize consumption. Geographically, the North American, European, and Asia Pacific regions are currently leading the market, driven by government initiatives and increasing consumer awareness. However, emerging markets in Latin America, Africa, and the Middle East are poised for rapid growth due to the increasing penetration of electricity grids and the growing demand for smart energy solutions.
The future of the SMS market is bright, with continued advancements in technology and the increasing adoption of renewable energy sources driving further innovation. The integration of artificial intelligence (AI) and machine learning (ML) into SMS solutions will enable real-time energy optimization, predictive maintenance, and personalized energy management. Moreover, the development of smart grids and the increasing adoption of electric vehicles (EVs) will create new opportunities for SMS providers. The SMS market is poised to continue its rapid growth, empowering utilities and consumers to navigate the evolving energy landscape with greater efficiency, sustainability, and control.
The Future of Smart Metering Systems: A Bright Outlook for Efficiency and Innovation
Smart metering systems, which enable the real-time monitoring and management of energy consumption, are poised for significant growth in the coming years. The increasing demand for energy efficiency, coupled with the growing adoption of renewable energy sources, is driving the adoption of these advanced technologies. The global smart meter market is expected to witness substantial growth, driven by factors such as government initiatives promoting energy conservation, the need for improved grid management, and the increasing demand for real-time energy consumption data.
The future of smart metering systems is characterized by several key trends. One notable development is the integration of artificial intelligence (AI) and machine learning (ML) algorithms. These technologies can optimize energy usage by analyzing consumption patterns and predicting demand fluctuations. AI-powered smart meters can also identify potential anomalies and detect energy theft, enhancing grid security and reliability. Furthermore, the convergence of smart metering with other smart technologies, such as smart grids and Internet of Things (IoT) devices, is creating new opportunities for integrated energy management solutions. This interconnectedness enables seamless communication and data exchange between various components, leading to more efficient and responsive energy systems.
The adoption of advanced communication technologies, such as 5G and low-power wide-area networks (LPWANs), is playing a crucial role in the evolution of smart metering systems. These technologies provide faster and more reliable data transmission, enabling real-time monitoring and control of energy consumption. Additionally, the development of advanced metering infrastructure (AMI) is enabling utilities to provide personalized energy feedback to consumers, empowering them to make informed decisions about their energy usage. AMI facilitates two-way communication, enabling utilities to remotely manage meters, implement demand response programs, and offer value-added services.
The future of smart metering systems holds immense potential for driving innovation and improving energy efficiency. By embracing AI, IoT, and advanced communication technologies, these systems will empower consumers, enhance grid reliability, and contribute to the transition towards a more sustainable energy future. As the global demand for energy efficiency intensifies and the need for smart grid solutions grows, smart metering systems are poised to play a vital role in shaping the future of the energy sector.
Smart Metering Systems: A Boon for Operational Efficiency
Smart metering systems (SMS) have revolutionized energy consumption tracking and management, offering a multitude of benefits for utilities and consumers alike. These advanced metering infrastructure (AMI) systems employ digital technology to collect and transmit real-time data on energy usage, providing a comprehensive and granular view of consumption patterns. This detailed information empowers utilities to optimize their operations, enhance grid stability, and improve customer service.
The impact of SMS on operational efficiency is multifaceted. Utilities can leverage real-time data to identify and address potential problems proactively, such as power outages and equipment failures. By analyzing usage patterns, utilities can better predict demand fluctuations, enabling them to adjust generation capacity and ensure a reliable supply of electricity. Moreover, SMS facilitates load balancing, enabling utilities to optimize the distribution of energy across the grid, minimizing losses and improving overall efficiency.
Furthermore, SMS empowers utilities to streamline billing and customer service. Accurate and timely data on energy consumption eliminates the need for manual meter readings, reducing administrative costs and errors. This information also allows utilities to provide customers with detailed breakdowns of their energy usage, facilitating informed consumption decisions and promoting energy conservation. By proactively addressing billing issues and providing personalized support, utilities can improve customer satisfaction and build stronger relationships.
Looking ahead, SMS is expected to play an increasingly vital role in the transition towards a more sustainable energy future. By enabling the integration of renewable energy sources, demand response programs, and smart grids, SMS will contribute to a more efficient and resilient energy ecosystem. The widespread adoption of SMS is poised to unlock significant operational efficiencies for utilities, while empowering consumers to take control of their energy consumption and contribute to a greener future.
Predictive Risk Assessment for Smart Metering Systems
Smart metering systems (SMS) have the potential to revolutionize the energy industry, but they also present unique cybersecurity risks. A comprehensive risk assessment is crucial to ensure the secure and reliable operation of these systems. The assessment should consider various factors, including the potential for unauthorized access, data breaches, system failures, and malicious attacks.
A key aspect of risk assessment is identifying potential threats and vulnerabilities. For example, SMS are susceptible to denial-of-service (DoS) attacks, which could disrupt energy supply. Data breaches could lead to the theft of sensitive customer information, including energy consumption patterns. Malware infections could compromise the integrity of data and control systems. The assessment should also consider the potential for physical tampering with meters and communication infrastructure.
Once potential threats and vulnerabilities are identified, a risk assessment should evaluate the likelihood and impact of each risk. Likelihood is the probability that a particular threat will exploit a vulnerability. Impact refers to the consequences of a successful attack. A risk matrix can be used to prioritize risks based on their likelihood and impact. This allows organizations to focus on the most critical threats and allocate resources effectively.
Finally, a comprehensive risk assessment should develop appropriate mitigation strategies to address identified risks. These strategies might include implementing robust security controls, such as strong authentication, encryption, and access control. Regular security audits and vulnerability assessments are also crucial to ensure the continued effectiveness of security measures. Additionally, training and awareness programs can help educate employees and users about cybersecurity risks and best practices. By effectively managing cybersecurity risks, organizations can maximize the benefits of SMS while ensuring the safety and security of their systems and data.
References
- R. Sutton and A. Barto. Introduction to reinforcement learning. MIT Press, 1998
- Breusch, T. S. A. R. Pagan (1979), "A simple test for heteroskedasticity and random coefficient variation," Econometrica, 47, 1287–1294.
- Banerjee, A., J. J. Dolado, J. W. Galbraith, D. F. Hendry (1993), Co-integration, Error-correction, and the Econometric Analysis of Non-stationary Data. Oxford: Oxford University Press.
- J. Ott. A Markov decision model for a surveillance application and risk-sensitive Markov decision processes. PhD thesis, Karlsruhe Institute of Technology, 2010.
- Thomas P, Brunskill E. 2016. Data-efficient off-policy policy evaluation for reinforcement learning. In Pro- ceedings of the International Conference on Machine Learning, pp. 2139–48. La Jolla, CA: Int. Mach. Learn. Soc.
- Athey S, Bayati M, Doudchenko N, Imbens G, Khosravi K. 2017a. Matrix completion methods for causal panel data models. arXiv:1710.10251 [math.ST]
- Bai J, Ng S. 2002. Determining the number of factors in approximate factor models. Econometrica 70:191–221