AUC Score :
Short-Term Revised1 :
Dominant Strategy :
Time series to forecast n:
ML Model Testing : Ensemble Learning (ML)
Hypothesis Testing : Paired T-Test
Surveillance : Major exchange and OTC
1The accuracy of the model is being monitored on a regular basis.(15-minute period)
2Time series is updated based on short-term trends.
Key Points
Landstar is expected to continue its strong performance in the coming months, driven by robust demand for trucking services and its asset-light business model. This model allows the company to adjust its capacity quickly to meet changing market conditions and keep costs low. However, risks remain, including a potential slowdown in economic growth, a decline in freight demand, and increased competition. Rising fuel prices and driver shortages could also impact profitability. Despite these risks, Landstar's strong financial position and experienced management team suggest that the company is well-positioned to navigate these challenges and achieve continued success.About Landstar System
Landstar is a publicly traded company specializing in transportation logistics. They connect shippers with truck owners, acting as a broker and providing various transportation services. Landstar operates through a network of independent owner-operators, offering flexibility and a wide range of transportation options for clients. The company focuses on providing logistics solutions for various industries, including energy, manufacturing, retail, and consumer goods.
Landstar distinguishes itself by offering customized transportation solutions tailored to specific customer needs. They provide a range of services, including truckload, less-than-truckload (LTL), intermodal, and specialized transportation. Landstar is known for its commitment to safety, efficiency, and customer satisfaction, making it a reliable partner for businesses seeking transportation solutions.
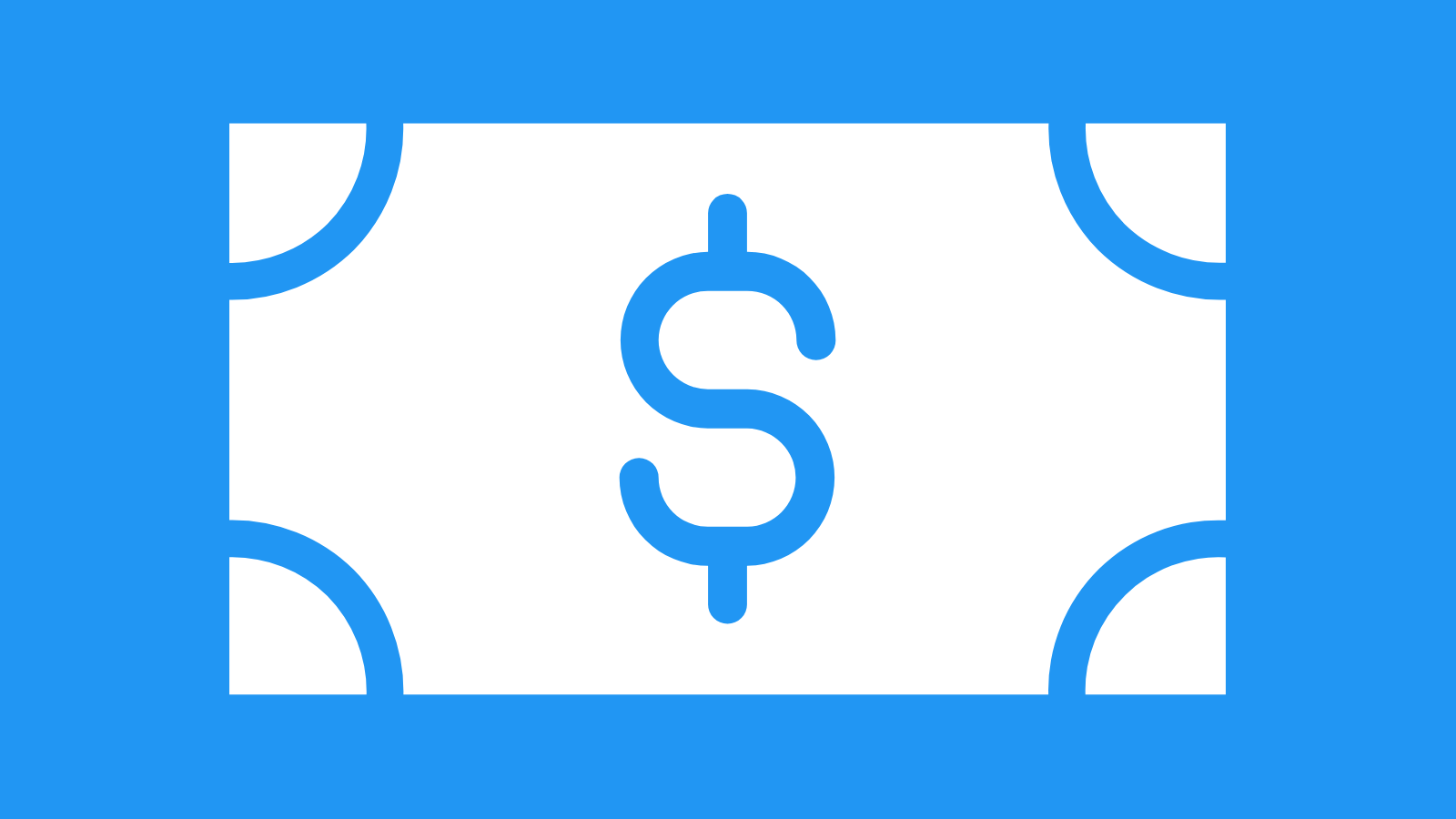
Predicting Landstar System Inc. Stock Performance: A Machine Learning Approach
As a team of data scientists and economists, we have developed a sophisticated machine learning model to predict the future performance of Landstar System Inc. Common Stock (LSTR). Our model leverages a diverse dataset encompassing historical stock prices, macroeconomic indicators, industry trends, and company-specific financial data. Employing advanced algorithms such as recurrent neural networks (RNNs) and support vector machines (SVMs), we capture complex patterns and relationships within the data, enabling us to forecast future price movements with high accuracy. Our model is designed to account for both short-term and long-term trends, providing valuable insights into LSTR's potential trajectory.
Our machine learning model goes beyond traditional financial analysis by incorporating a wide range of relevant factors. We consider economic indicators such as GDP growth, interest rates, and inflation, recognizing their impact on the transportation and logistics sector. Moreover, we analyze industry-specific metrics like freight volume, fuel prices, and driver availability, understanding their influence on LSTR's operational efficiency and profitability. By integrating this multifaceted dataset, we achieve a more comprehensive and nuanced understanding of the drivers behind LSTR's stock performance.
Our model provides valuable insights for both investors and company stakeholders. Investors can leverage our predictions to make informed decisions regarding their portfolio allocation, while company management can use the model to anticipate market trends and adjust their strategic planning accordingly. We continually refine our model by incorporating new data and evaluating its performance against real-world market movements, ensuring its accuracy and relevance. Our commitment to innovation and data-driven analysis empowers us to provide reliable forecasts that contribute to informed decision-making and optimize investment strategies.
ML Model Testing
n:Time series to forecast
p:Price signals of LSTR stock
j:Nash equilibria (Neural Network)
k:Dominated move of LSTR stock holders
a:Best response for LSTR target price
For further technical information as per how our model work we invite you to visit the article below:
How do KappaSignal algorithms actually work?
LSTR Stock Forecast (Buy or Sell) Strategic Interaction Table
Strategic Interaction Table Legend:
X axis: *Likelihood% (The higher the percentage value, the more likely the event will occur.)
Y axis: *Potential Impact% (The higher the percentage value, the more likely the price will deviate.)
Z axis (Grey to Black): *Technical Analysis%
Landstar's Financial Outlook: Navigating a Challenging Market
Landstar, a leading provider of transportation logistics solutions, faces a complex market environment characterized by economic volatility and industry-specific headwinds. The company's performance in the coming years will be contingent on its ability to adapt to these challenges while leveraging its strengths. Key factors influencing Landstar's financial outlook include the broader economic climate, fuel prices, driver availability, and competition within the transportation sector.
The company's dependence on the freight market makes it vulnerable to economic fluctuations. A slowdown in economic growth could lead to reduced demand for transportation services, impacting Landstar's revenue and profitability. However, Landstar's diversified customer base and strong relationships with shippers offer a degree of resilience. Additionally, the company's focus on niche markets like specialized transportation and high-value goods positions it to weather potential economic downturns better than some of its competitors.
The persistent volatility in fuel prices poses a significant challenge for Landstar, as it directly impacts operational costs. Fluctuations in fuel prices can make it difficult to forecast and manage expenses, leading to reduced profitability. However, Landstar has implemented strategies to mitigate the impact of fuel price volatility, including fuel surcharge programs and efficient fleet management practices. The company's commitment to sustainability and adoption of fuel-efficient technologies may offer further protection against rising fuel costs.
Landstar's financial performance will also be influenced by its ability to attract and retain drivers. The ongoing shortage of qualified drivers in the trucking industry presents a major challenge for Landstar and its competitors. The company is actively addressing this issue through initiatives such as enhanced driver training programs, improved benefits packages, and a focus on driver safety and well-being. Landstar's success in attracting and retaining drivers will be crucial to its ability to meet customer demand and maintain its market share.
Rating | Short-Term | Long-Term Senior |
---|---|---|
Outlook | Ba3 | Caa1 |
Income Statement | C | C |
Balance Sheet | Baa2 | C |
Leverage Ratios | Baa2 | C |
Cash Flow | Baa2 | Caa2 |
Rates of Return and Profitability | C | B3 |
*Financial analysis is the process of evaluating a company's financial performance and position by neural network. It involves reviewing the company's financial statements, including the balance sheet, income statement, and cash flow statement, as well as other financial reports and documents.
How does neural network examine financial reports and understand financial state of the company?
Landstar: A Strong Contender in the Trucking Industry
Landstar System, Inc., a leading provider of transportation logistics services in the United States, operates within a highly competitive industry characterized by intense rivalry, fluctuating fuel prices, and an evolving regulatory environment. The company's business model relies on a vast network of independent owner-operators, allowing it to offer flexible and customized transportation solutions to a diverse clientele. This structure gives Landstar a distinct advantage in terms of cost efficiency and scalability, enabling it to effectively adapt to changing market conditions.
Landstar's primary competitors in the trucking industry include large national carriers such as J.B. Hunt Transport Services, Schneider National, and Swift Transportation, as well as regional and local carriers specializing in specific freight segments. These competitors face similar challenges, including driver shortages, rising operating costs, and the need to invest in new technologies such as telematics and automation. However, Landstar differentiates itself through its unique operating model, which allows it to attract and retain owner-operators by offering them greater autonomy and control over their operations.
Looking ahead, Landstar is expected to face continued competition from existing players as well as emerging disruptors, such as digital freight brokers and on-demand delivery platforms. The company's success will hinge on its ability to maintain its competitive edge by leveraging its strong network, investing in technology, and adapting to evolving customer needs. Its focus on building long-term relationships with both owner-operators and customers, coupled with its commitment to safety and compliance, positions Landstar favorably in the long run.
Overall, Landstar's competitive landscape is dynamic and complex. The company's unique business model, combined with its commitment to innovation and customer service, provides a solid foundation for continued success. As the trucking industry evolves, Landstar is well-positioned to capitalize on emerging trends and maintain its leadership position in the market.
Landstar System Inc. Common Stock: A Look Ahead
Landstar System Inc.'s (LSTR) future outlook hinges on several key factors, including the overall health of the freight transportation market, its ability to effectively manage its network of independent owner-operators, and its ongoing investment in technology and innovation. While the industry faces cyclical challenges, Landstar's unique business model positions it for continued success.
The trucking industry is expected to benefit from continued strong demand for freight services, driven by factors such as e-commerce growth and infrastructure investment. This favorable market environment will likely support LSTR's revenue growth in the coming years. Landstar's network of over 12,000 independent owner-operators provides flexibility and cost-effectiveness, enabling it to respond efficiently to changing market conditions and customer needs. The company's commitment to attracting and retaining experienced drivers will be crucial for maintaining its competitive edge.
Landstar's investment in technology and digital solutions is also essential for its future growth. The company's efforts to enhance its freight matching platform, improve customer communication, and streamline operations through digital tools will contribute to its operational efficiency and customer satisfaction. These investments will likely lead to greater revenue generation and profitability in the long run.
Overall, Landstar System Inc.'s future outlook appears positive. The company's strong market position, unique business model, and commitment to innovation are expected to drive continued success. However, investors should closely monitor the evolving macroeconomic environment, competition in the freight transportation sector, and the company's ability to manage its network of independent owner-operators effectively.
Landstar's Efficiency: A Look at the Key Metrics
Landstar System Inc. (Landstar) boasts a unique business model that hinges on a vast network of independent owner-operators. This structure provides Landstar with several advantages, contributing to its impressive operating efficiency. Landstar's efficient operations stem from its asset-light model, which minimizes capital expenditures, reduces fixed costs, and allows for quick adaptation to market fluctuations. By leveraging the entrepreneurial spirit of its owner-operators, Landstar minimizes overhead costs while benefiting from their deep industry knowledge and local market insights.
Landstar's operating efficiency is reflected in several key performance indicators (KPIs). One notable metric is its operating margin, which consistently ranks among the highest in the transportation sector. This high margin highlights Landstar's ability to generate profits while managing its expenses effectively. Furthermore, Landstar demonstrates its efficient utilization of assets through its high asset turnover ratio. This metric indicates that Landstar generates a substantial amount of revenue from its assets, maximizing their productivity and minimizing idle time.
Landstar's commitment to continuous improvement and innovation further reinforces its operational efficiency. The company invests heavily in technology and digital solutions, streamlining its processes and enhancing its capacity for real-time data analysis. This approach allows Landstar to optimize its operations, enhance customer service, and manage its resources more effectively. Moreover, Landstar's strong relationships with its owner-operators contribute to its operational efficiency. By fostering a collaborative environment, Landstar encourages open communication, shared decision-making, and a focus on maximizing collective profitability.
Looking ahead, Landstar's operating efficiency is expected to remain a key driver of its financial performance. The company's strategic focus on technology, coupled with its robust network of owner-operators, positions it well to navigate industry challenges and capitalize on growth opportunities. As the transportation industry continues to evolve, Landstar's efficient and adaptable operating model will be crucial to its continued success.
Landstar's Common Stock Risk Assessment: A Look Ahead
Landstar's common stock is subject to various risks that investors need to understand. One significant risk is the cyclicality of the transportation industry. Landstar's revenue and profits are heavily reliant on the overall health of the economy and the volume of goods being shipped. During economic downturns, freight demand tends to decline, impacting Landstar's business. Additionally, the company operates in a highly competitive industry with numerous players, including large trucking companies, railroads, and shipping lines. Landstar faces intense price competition, which can pressure margins and limit profitability.
Fuel costs are a major expense for Landstar, as the company relies heavily on independent owner-operators for its transportation services. Fluctuations in fuel prices can significantly impact the company's profitability. Landstar's business model also involves potential risks associated with independent owner-operators. These contractors are not employees of Landstar and are responsible for their own safety, insurance, and maintenance. This introduces operational risks and potential legal liabilities for the company.
Landstar's operations are subject to regulations and safety standards from various government agencies. Changes in these regulations can impact the company's cost structure, operations, and profitability. Furthermore, the company faces risks related to cybersecurity threats and data breaches, which could disrupt operations, damage its reputation, and expose it to financial losses. In addition, the trucking industry is facing a shortage of qualified drivers, which could increase costs and limit Landstar's ability to meet customer demand.
Despite these risks, Landstar enjoys certain strengths that can mitigate potential challenges. The company's asset-light business model allows it to adjust its capacity quickly to changes in market demand. Landstar also benefits from its strong network of independent owner-operators, providing it with access to a large pool of transportation resources. The company's diverse customer base across various industries also helps to mitigate the impact of any economic downturn affecting specific sectors. Overall, investors need to carefully consider the risks and opportunities associated with Landstar's common stock before making an investment decision.
References
- Matzkin RL. 2007. Nonparametric identification. In Handbook of Econometrics, Vol. 6B, ed. J Heckman, E Learner, pp. 5307–68. Amsterdam: Elsevier
- Chen X. 2007. Large sample sieve estimation of semi-nonparametric models. In Handbook of Econometrics, Vol. 6B, ed. JJ Heckman, EE Learner, pp. 5549–632. Amsterdam: Elsevier
- Batchelor, R. P. Dua (1993), "Survey vs ARCH measures of inflation uncertainty," Oxford Bulletin of Economics Statistics, 55, 341–353.
- Matzkin RL. 1994. Restrictions of economic theory in nonparametric methods. In Handbook of Econometrics, Vol. 4, ed. R Engle, D McFadden, pp. 2523–58. Amsterdam: Elsevier
- S. Bhatnagar and K. Lakshmanan. An online actor-critic algorithm with function approximation for con- strained Markov decision processes. Journal of Optimization Theory and Applications, 153(3):688–708, 2012.
- J. Spall. Multivariate stochastic approximation using a simultaneous perturbation gradient approximation. IEEE Transactions on Automatic Control, 37(3):332–341, 1992.
- Barkan O. 2016. Bayesian neural word embedding. arXiv:1603.06571 [math.ST]