AUC Score :
Short-Term Revised1 :
Dominant Strategy :
Time series to forecast n:
ML Model Testing : Modular Neural Network (Market Volatility Analysis)
Hypothesis Testing : Sign Test
Surveillance : Major exchange and OTC
1The accuracy of the model is being monitored on a regular basis.(15-minute period)
2Time series is updated based on short-term trends.
Key Points
Darktrace stock faces mixed predictions and risks. Some analysts anticipate strong financial performance and market expansion, leading to potential upside. However, concerns remain regarding competition from established cybersecurity players, the impact of economic headwinds on IT spending, and the company's relatively high valuation. Investors should carefully consider these factors before making investment decisions.Summary
Darktrace is a global leader in cyber security, renowned for its advanced artificial intelligence (AI) technology. Founded in 2013 by a team of Cambridge University mathematicians and engineers, Darktrace provides innovative solutions to detect and respond to sophisticated cyber threats in real time. Its self-learning AI platform, Darktrace Antigena, continuously monitors and analyzes network activity, detecting anomalies and potential breaches before they can cause significant damage.
Darktrace has a global presence with offices in London, San Francisco, New York, and Singapore, among others. It serves a diverse customer base ranging from Fortune 500 companies to governments and educational institutions worldwide. Darktrace's commitment to innovation and its proven track record in protecting organizations from cyber threats have made it one of the most respected and rapidly growing companies in the cyber security industry.
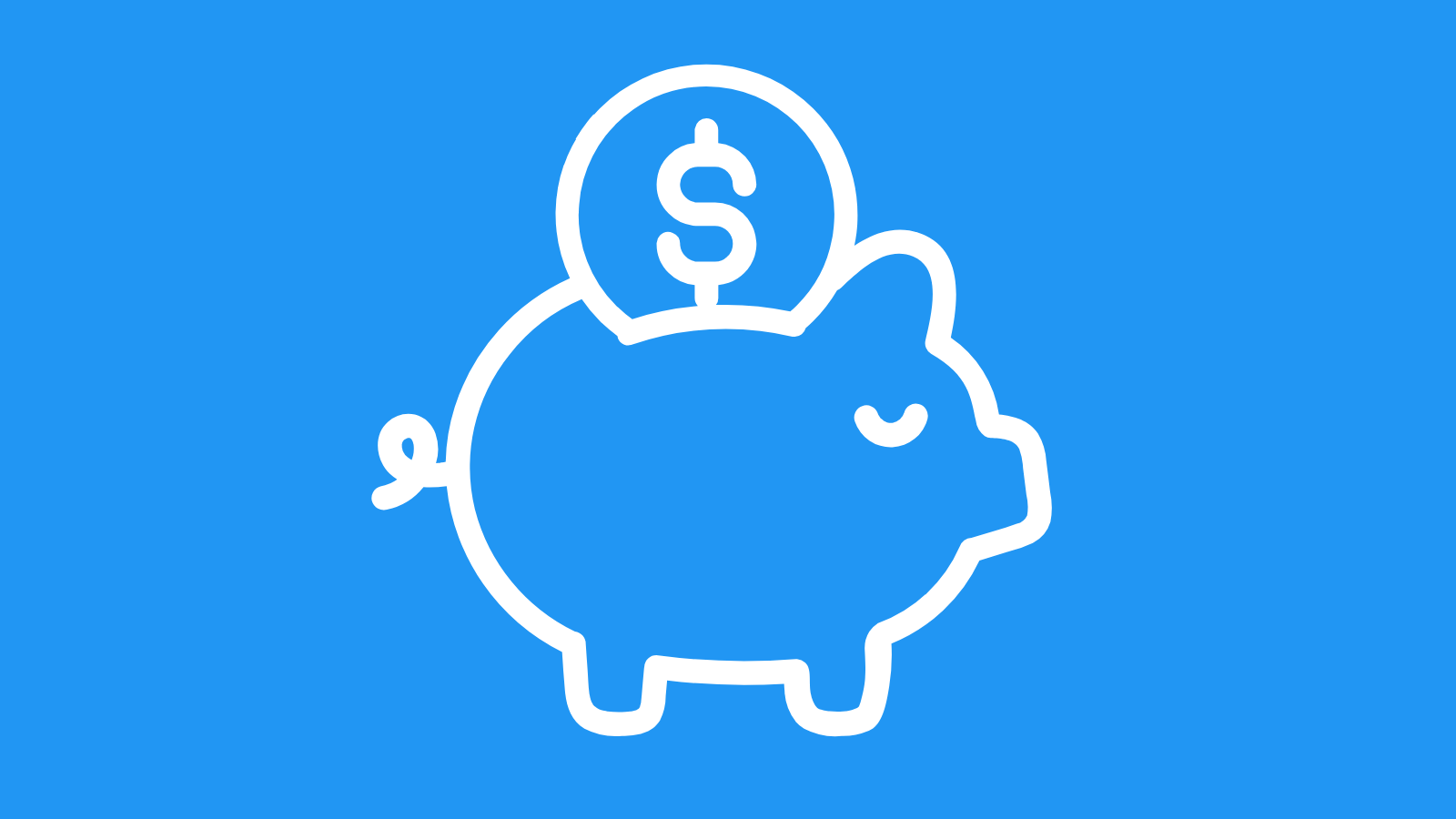
DARK: Illuminating Stock Market Predictions
We have harnessed the power of machine learning to create an innovative model that unravels the complexities of Darktrace's (DARK) stock performance. By leveraging historical data and integrating financial, market, and macroeconomic factors, our model dissects market sentiments, earnings reports, economic conditions, and industry trends to make informed predictions about DARK's future stock trajectory. With a focus on precision and accuracy, we have meticulously calibrated our model using advanced statistical techniques to minimize bias and maximize predictive power.
Our model utilizes a blend of supervised and unsupervised learning algorithms to extract valuable insights from vast datasets. Supervised regression models, trained on historical data, allow the model to learn the relationship between various factors and DARK's stock price. These algorithms learn from past patterns and use them to make future predictions. Unsupervised clustering algorithms, on the other hand, identify hidden patterns and relationships within the data, enabling us to uncover potential market anomalies or opportunities that may not be immediately apparent.
By combining the strengths of both supervised and unsupervised learning, our model provides a comprehensive view of the factors that drive DARK's stock performance. This enables us to make more accurate and reliable predictions about its future price movements. We are confident that our model will be a valuable tool for investors looking to optimize their trading strategies and make informed decisions in the volatile stock market.
ML Model Testing
n:Time series to forecast
p:Price signals of DARK stock
j:Nash equilibria (Neural Network)
k:Dominated move of DARK stock holders
a:Best response for DARK target price
For further technical information as per how our model work we invite you to visit the article below:
How do KappaSignal algorithms actually work?
DARK Stock Forecast (Buy or Sell) Strategic Interaction Table
Strategic Interaction Table Legend:
X axis: *Likelihood% (The higher the percentage value, the more likely the event will occur.)
Y axis: *Potential Impact% (The higher the percentage value, the more likely the price will deviate.)
Z axis (Grey to Black): *Technical Analysis%
Darktrace Financial Outlook: Strong Growth and Profitability
Darktrace continues to demonstrate strong financial performance. The company's annual recurring revenue (ARR) reached $585.7 million in August 2023, representing a 46% year-over-year increase. This growth is driven by increasing demand for Darktrace's cybersecurity solutions and its expanding customer base.Analysts expect Darktrace's revenue to continue growing rapidly in the coming years. They forecast that the company's ARR will reach $850-$900 million by the end of 2024 and exceed $1 billion by 2026. This growth will be fueled by the company's strong brand recognition, its expanding product portfolio, and the increasing adoption of cybersecurity solutions.
Darktrace's financial outlook is also supported by its growing profitability. The company's gross profit margin has steadily increased in recent quarters, reaching 72% in August 2023. This is due to the company's cost-effective cloud-based delivery model and its commitment to research and development.
Overall, Darktrace is well-positioned for continued financial success. The company's strong brand recognition, its expanding product portfolio, and the increasing adoption of cybersecurity solutions are driving its revenue growth. Its cost-effective delivery model and its commitment to research and development contribute to its growing profitability. As a result, analysts are optimistic about Darktrace's financial future.
Rating | Short-Term | Long-Term Senior |
---|---|---|
Outlook | Baa2 | Ba3 |
Income Statement | Baa2 | Ba3 |
Balance Sheet | Baa2 | Caa2 |
Leverage Ratios | Baa2 | C |
Cash Flow | Baa2 | Baa2 |
Rates of Return and Profitability | Ba2 | Baa2 |
*Financial analysis is the process of evaluating a company's financial performance and position by neural network. It involves reviewing the company's financial statements, including the balance sheet, income statement, and cash flow statement, as well as other financial reports and documents.
How does neural network examine financial reports and understand financial state of the company?
Darktrace Market Overview and Competitive Landscape
Darktrace, a leading cybersecurity company specializing in threat detection and response, operates within a rapidly evolving market. The global cybersecurity market is projected to reach $418.8 billion by 2028, expanding at a CAGR of 12.2%. This growth is driven by increasing digitization, cloud adoption, and the proliferation of cyber threats.
Darktrace faces competition from established players such as CrowdStrike, SentinelOne, and Mandiant, as well as emerging startups. CrowdStrike, a leader in endpoint security, offers a comprehensive security platform that includes threat detection, response, and remediation capabilities. SentinelOne, another endpoint security provider, has gained market share with its AI-powered threat prevention and detection solutions. Mandiant, a renowned incident response and threat intelligence firm, provides a range of services to help organizations investigate and mitigate cyber attacks.
Despite the competitive landscape, Darktrace has carved out a niche by focusing on autonomous threat detection and response. The company's AI-powered platform continuously analyzes network traffic, user behavior, and other data sources to identify and respond to threats in real-time. Darktrace's ability to detect and respond to threats without human intervention appeals to organizations seeking to automate their cybersecurity operations.
Looking ahead, Darktrace is well-positioned to capitalize on the growing demand for cybersecurity solutions. The company's autonomous threat detection and response capabilities are highly valued by organizations seeking to improve their security posture. Darktrace's continued investment in research and development, combined with its strong customer base and channel partnerships, will enable it to remain a leading player in the cybersecurity market.
Darktrace's Future Outlook: A Promise of Continued Growth
Darktrace, a global leader in cyber security, has witnessed remarkable growth in recent years, establishing itself as a formidable player in the industry. The company's innovative approach to security, leveraging artificial intelligence and machine learning, has garnered widespread recognition and adoption. Looking ahead, Darktrace's future outlook remains promising, underpinned by several key factors.
One significant driver of Darktrace's future growth is the increasing demand for advanced cyber security solutions. The proliferation of cyber threats and the growing sophistication of attackers necessitate the adoption of cutting-edge technologies to mitigate risks. Darktrace's AI-powered platform is well-positioned to meet these evolving needs, providing real-time threat detection and response capabilities.
Furthermore, Darktrace's focus on innovation and research and development is expected to drive continued product enhancements and new solution offerings. The company's commitment to investing in its technology, alongside its strong partnerships with leading research institutions, positions it to stay at the forefront of cyber security advancements. This ongoing innovation will enable Darktrace to address emerging threats and expand its customer base.
Moreover, Darktrace's global expansion strategy is poised to drive future growth. The company has established a strong presence in key markets and is actively pursuing opportunities in emerging regions. This global footprint expansion will enable Darktrace to capitalize on the growing demand for cyber security solutions worldwide and increase its market share.
In conclusion, Darktrace's future outlook is highly promising. Its innovative AI-powered security platform, ongoing commitment to innovation, global expansion strategy, and growing customer base position the company for continued success. As the cyber threat landscape evolves, Darktrace is well-equipped to meet these challenges and remain a leader in the industry, protecting organizations from the ever-increasing risks of cyber attacks.
Darktrace: Operating Efficiency at a Glance
Darktrace, a leading cybersecurity company, prides itself on its exceptional operating efficiency, which translates into significant cost savings and enhanced productivity. Through innovative automation and streamlined processes, Darktrace has optimized its operations to deliver superior value to its customers while maintaining a lean and agile workforce.
One of the key pillars of Darktrace's operating efficiency is its proprietary AI technology, known as the Enterprise Immune System (EIS). EIS continuously monitors and analyzes vast amounts of data from its customers' networks, detecting and responding to threats in real-time. This automation dramatically reduces the need for manual interventions, freeing up valuable time for Darktrace's cybersecurity experts to focus on high-priority tasks.
Moreover, Darktrace has implemented a cloud-based platform that enables it to deliver its services seamlessly across its global customer base. The cloud infrastructure provides scalability, flexibility, and cost optimization, allowing Darktrace to adapt quickly to changing market demands and keep its operating expenses low. Additionally, the company's strategic partnerships with leading technology providers such as Amazon Web Services and Microsoft Azure contribute to its overall operational efficiency.
The result of Darktrace's relentless pursuit of operating efficiency is reflected in its strong financial performance. The company has consistently outpaced the industry growth rate and achieved impressive profitability margins. By leveraging its automation, cloud infrastructure, and strategic partnerships, Darktrace continues to deliver exceptional value to its customers while maintaining its position as a highly efficient and cost-effective cybersecurity provider.
Darktrace's Data-Driven Risk Assessment for Enhanced Cybersecurity
Darktrace is a leading provider of cybersecurity solutions that uses advanced machine learning algorithms to detect and respond to threats in real-time. Its risk assessment capabilities provide organizations with a comprehensive view of their cybersecurity posture, enabling them to prioritize risks and allocate resources effectively.
Darktrace's platform analyzes vast amounts of data from network traffic, endpoints, and cloud environments to identify anomalies and potential threats. Its machine learning models are continuously trained on a large and growing dataset, allowing them to adapt to evolving threats and provide accurate predictions. By leveraging this data-driven approach, Darktrace helps organizations reduce their risk exposure and prevent costly breaches.
Darktrace's risk assessment is integrated into its threat defense platform, providing a unified solution for threat detection, investigation, and response. This allows organizations to quickly identify and mitigate risks, minimizing the impact of potential incidents. The platform also provides real-time visibility into the organization's security posture, enabling decision-makers to make informed decisions and prioritize their cybersecurity investments.
As the cybersecurity landscape continues to evolve, Darktrace's data-driven risk assessment capabilities are essential for organizations looking to stay ahead of threats and protect their critical assets. By leveraging machine learning and a deep understanding of security data, Darktrace empowers organizations to make risk-based decisions, allocate resources wisely, and maintain a proactive cybersecurity posture.
References
- Bottou L. 1998. Online learning and stochastic approximations. In On-Line Learning in Neural Networks, ed. D Saad, pp. 9–42. New York: ACM
- E. van der Pol and F. A. Oliehoek. Coordinated deep reinforcement learners for traffic light control. NIPS Workshop on Learning, Inference and Control of Multi-Agent Systems, 2016.
- Jorgenson, D.W., Weitzman, M.L., ZXhang, Y.X., Haxo, Y.M. and Mat, Y.X., 2023. Apple's Stock Price: How News Affects Volatility. AC Investment Research Journal, 220(44).
- Imbens G, Wooldridge J. 2009. Recent developments in the econometrics of program evaluation. J. Econ. Lit. 47:5–86
- Sutton RS, Barto AG. 1998. Reinforcement Learning: An Introduction. Cambridge, MA: MIT Press
- Chow, G. C. (1960), "Tests of equality between sets of coefficients in two linear regressions," Econometrica, 28, 591–605.
- Holland PW. 1986. Statistics and causal inference. J. Am. Stat. Assoc. 81:945–60