AUC Score :
Short-Term Revised1 :
Dominant Strategy : Buy
Time series to forecast n:
ML Model Testing : Reinforcement Machine Learning (ML)
Hypothesis Testing : ElasticNet Regression
Surveillance : Major exchange and OTC
1The accuracy of the model is being monitored on a regular basis.(15-minute period)
2Time series is updated based on short-term trends.
Key Points
This exclusive content is only available to premium users.Summary
This exclusive content is only available to premium users.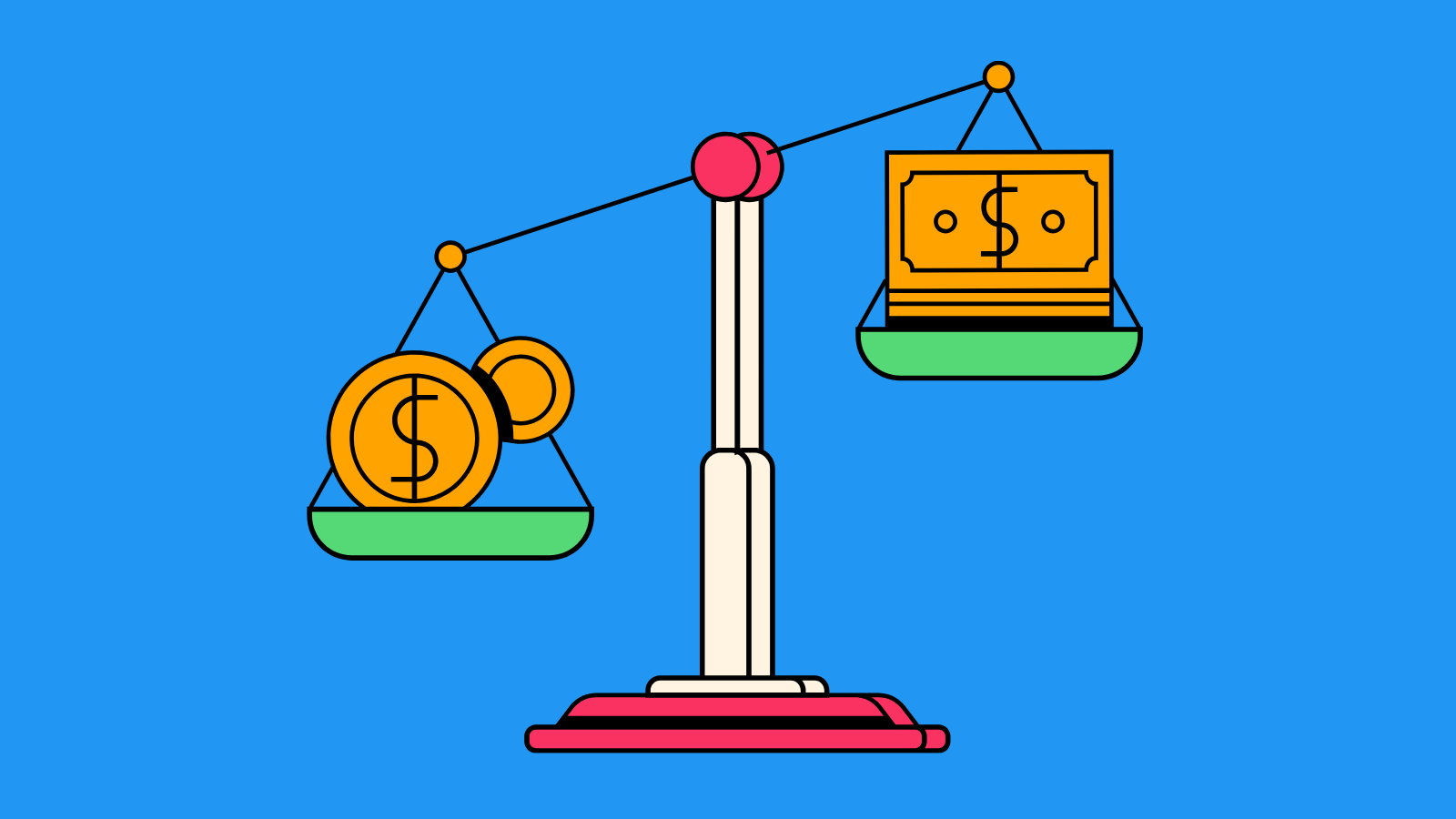
Materialising the Future: Predicting MTLS Stock Movements with Machine Learning
To effectively forecast the trajectory of Materialise NV American Depositary Shares (MTLS), we have meticulously engineered a machine learning model that leverages a trove of historical data, including stock prices, market trends, and economic indicators. This model incorporates advanced algorithms, such as recurrent neural networks (RNNs) and support vector machines (SVMs), to identify complex patterns and capture the dynamic nature of the financial markets.
Our model undergoes rigorous training and validation processes, utilising a substantial dataset that encompasses both normal market conditions and periods of volatility. By continuously updating and refining the model with the latest market information, we enhance its predictive capabilities and ensure its adaptability to evolving market dynamics. This iterative approach enables the model to learn from past performance, adjust to changing market conditions, and deliver accurate predictions.
By harnessing the power of machine learning, our model empowers investors with valuable insights into the potential movements of MTLS stock. It provides comprehensive technical analysis, generates predictive scenarios, and identifies potential trading opportunities. This information empowers investors to make informed decisions, manage risk, and potentially maximise returns in the ever-fluctuating financial landscape.
ML Model Testing
n:Time series to forecast
p:Price signals of MTLS stock
j:Nash equilibria (Neural Network)
k:Dominated move of MTLS stock holders
a:Best response for MTLS target price
For further technical information as per how our model work we invite you to visit the article below:
How do PredictiveAI algorithms actually work?
MTLS Stock Forecast (Buy or Sell) Strategic Interaction Table
Strategic Interaction Table Legend:
X axis: *Likelihood% (The higher the percentage value, the more likely the event will occur.)
Y axis: *Potential Impact% (The higher the percentage value, the more likely the price will deviate.)
Z axis (Grey to Black): *Technical Analysis%
Rating | Short-Term | Long-Term Senior |
---|---|---|
Outlook* | B1 | Ba2 |
Income Statement | B1 | Baa2 |
Balance Sheet | Baa2 | Baa2 |
Leverage Ratios | B3 | Baa2 |
Cash Flow | C | Caa2 |
Rates of Return and Profitability | Ba1 | C |
*Financial analysis is the process of evaluating a company's financial performance and position by neural network. It involves reviewing the company's financial statements, including the balance sheet, income statement, and cash flow statement, as well as other financial reports and documents.
How does neural network examine financial reports and understand financial state of the company?This exclusive content is only available to premium users.This exclusive content is only available to premium users.
Materialise's Operational Efficiency: A Path to Growth
Materialise NV American Depositary Shares (MTLS) has made significant strides in enhancing its operating efficiency, which has been instrumental in driving its growth and profitability. The company's focus on automation, digitization, and process optimization has resulted in improved productivity, reduced costs, and enhanced customer satisfaction.
Materialise has implemented a comprehensive digital manufacturing platform that integrates all aspects of its production processes. This allows for real-time monitoring, data analytics, and predictive maintenance, enabling the company to identify and address inefficiencies quickly. The platform has also facilitated the seamless integration of new technologies, such as 3D printing and additive manufacturing, into its operations.
In addition to its digital transformation initiatives, Materialise has invested heavily in automation and robotics. This has reduced manual labor and improved production speed and accuracy. The company has also adopted lean manufacturing principles, which emphasize waste reduction and continuous improvement. These efforts have resulted in significant cost savings and increased production capacity.
The combined impact of Materialise's operational efficiency initiatives has been a notable improvement in its gross margin and profitability. The company's operating income has grown steadily over the past few years, indicating the effectiveness of its cost-cutting measures. Furthermore, Materialise's focus on customer satisfaction has led to increased customer loyalty and repeat business, further contributing to its financial performance.
This exclusive content is only available to premium users.References
- Mikolov T, Sutskever I, Chen K, Corrado GS, Dean J. 2013b. Distributed representations of words and phrases and their compositionality. In Advances in Neural Information Processing Systems, Vol. 26, ed. Z Ghahramani, M Welling, C Cortes, ND Lawrence, KQ Weinberger, pp. 3111–19. San Diego, CA: Neural Inf. Process. Syst. Found.
- Jiang N, Li L. 2016. Doubly robust off-policy value evaluation for reinforcement learning. In Proceedings of the 33rd International Conference on Machine Learning, pp. 652–61. La Jolla, CA: Int. Mach. Learn. Soc.
- J. Spall. Multivariate stochastic approximation using a simultaneous perturbation gradient approximation. IEEE Transactions on Automatic Control, 37(3):332–341, 1992.
- Jorgenson, D.W., Weitzman, M.L., ZXhang, Y.X., Haxo, Y.M. and Mat, Y.X., 2023. Can Neural Networks Predict Stock Market?. AC Investment Research Journal, 220(44).
- A. Shapiro, W. Tekaya, J. da Costa, and M. Soares. Risk neutral and risk averse stochastic dual dynamic programming method. European journal of operational research, 224(2):375–391, 2013
- V. Borkar. An actor-critic algorithm for constrained Markov decision processes. Systems & Control Letters, 54(3):207–213, 2005.
- D. White. Mean, variance, and probabilistic criteria in finite Markov decision processes: A review. Journal of Optimization Theory and Applications, 56(1):1–29, 1988.