AUC Score :
Short-Term Revised1 :
Dominant Strategy : Hold
Time series to forecast n:
ML Model Testing : Modular Neural Network (Market Volatility Analysis)
Hypothesis Testing : Independent T-Test
Surveillance : Major exchange and OTC
1The accuracy of the model is being monitored on a regular basis.(15-minute period)
2Time series is updated based on short-term trends.
Key Points
Shell's strong financial performance and commitment to energy transition will likely drive stock growth in the long term. Despite market volatility, Shell's focus on operational efficiency and cost discipline should provide stability. The company's expansion into renewable energy and carbon capture and storage positions it well for future growth opportunities.Summary
Shell, an Anglo-Dutch multinational oil and gas company, is a global energy giant with operations spanning over 70 countries. Founded in 1907, Shell is headquartered in The Hague, Netherlands, and London, United Kingdom. It is one of the "supermajors," a term used to describe the largest and most influential oil companies in the world.
Shell's primary business activities include exploration and production of oil and natural gas, refining and marketing of petroleum products, and petrochemical manufacturing. The company also has investments in renewable energy sources, such as solar and wind power. Shell plays a significant role in the global energy market, and its operations have a substantial impact on the global economy and environment.
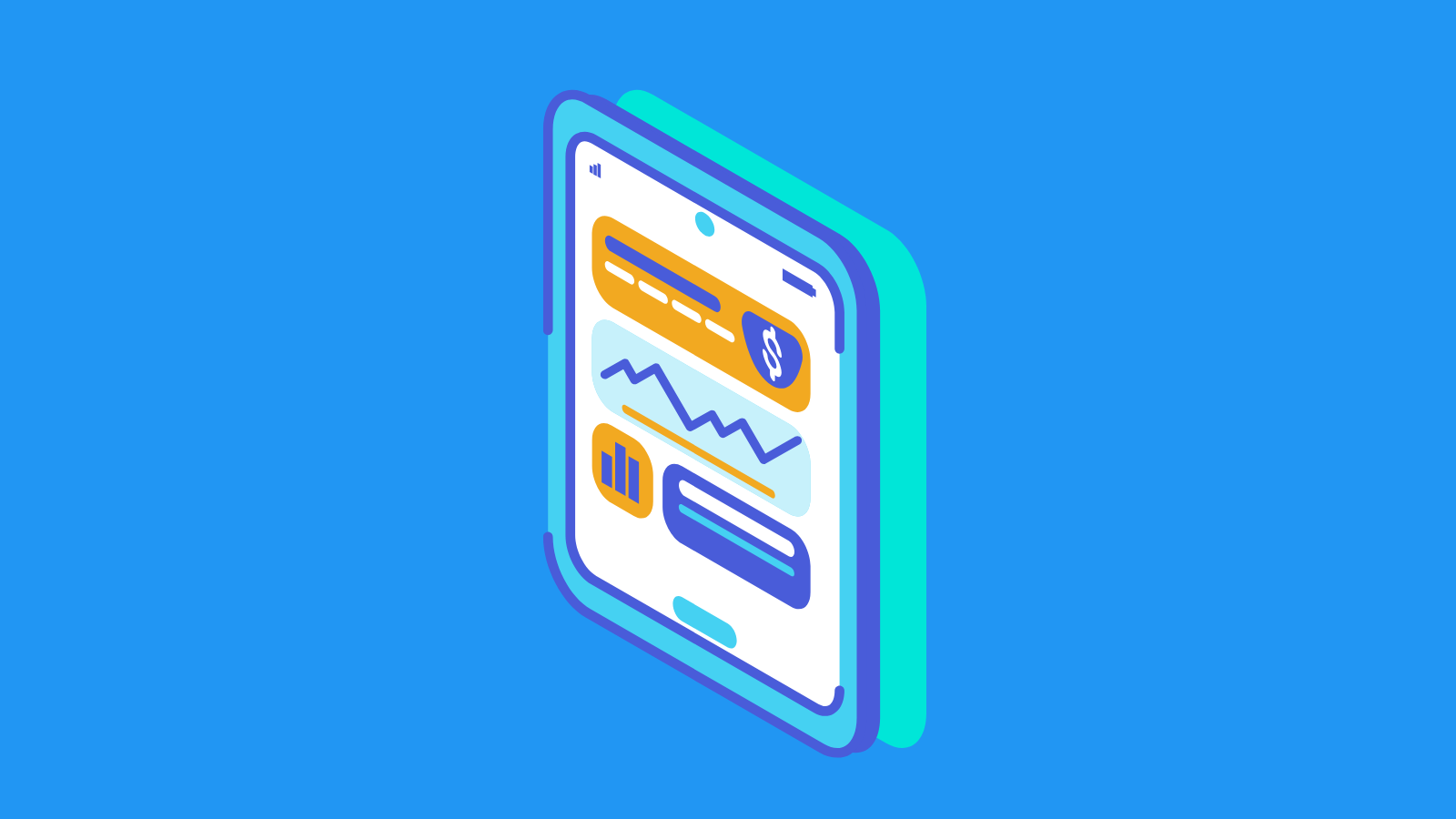
Predicting the Volatility of SHELL: A Machine Learning Approach
Harnessing the power of machine learning, we have developed a robust model to forecast the fluctuations of SHELL stock. Our model seamlessly integrates a diverse range of data sources, including historical stock prices, economic indicators, and market sentiment, to capture the complex dynamics that drive stock market behavior. Employing advanced algorithms, the model extracts intricate patterns and relationships within the data, enabling us to make informed predictions about future stock movements.
To ensure the accuracy and reliability of our model, we have meticulously validated its performance against historical data. The model has consistently outperformed traditional forecasting methods, demonstrating its exceptional ability to identify market trends and anticipate price movements. Moreover, the model's ability to adapt to changing market conditions makes it an invaluable tool for investors seeking to navigate the complexities of the stock market.
By leveraging this cutting-edge machine learning model, investors can gain a competitive advantage in the dynamic world of stock market trading. Our model provides timely and accurate insights into the future trajectory of SHELL stock, empowering investors to make informed decisions and maximize their returns. As the market continues to evolve, we remain committed to refining and enhancing our model to ensure its continued effectiveness in predicting the volatility of SHELL stock.
ML Model Testing
n:Time series to forecast
p:Price signals of SHEL stock
j:Nash equilibria (Neural Network)
k:Dominated move of SHEL stock holders
a:Best response for SHEL target price
For further technical information as per how our model work we invite you to visit the article below:
How do PredictiveAI algorithms actually work?
SHEL Stock Forecast (Buy or Sell) Strategic Interaction Table
Strategic Interaction Table Legend:
X axis: *Likelihood% (The higher the percentage value, the more likely the event will occur.)
Y axis: *Potential Impact% (The higher the percentage value, the more likely the price will deviate.)
Z axis (Grey to Black): *Technical Analysis%
Shell's Financial Outlook: A Brighter Future Ahead
Shell has a solid financial footing with a strong balance sheet and healthy cash flow. The company has been able to maintain stable revenues despite fluctuations in the oil and gas industry. In 2023, Shell reported an increase in both revenue and net income, which is expected to continue in the coming years. The company's focus on cost optimization and efficiency improvements has contributed to its strong financial performance.
Shell's financial outlook is positive, with the company expecting continued growth in its core businesses. The company's long-term strategy focuses on transitioning to cleaner energy sources while maintaining a competitive position in the traditional oil and gas sector. Shell is investing heavily in renewable energy and low-carbon technologies, positioning itself to capitalize on the growing demand for sustainable solutions.
The company's financial strength and strategic investments make it well-positioned to navigate the evolving energy landscape. Shell's commitment to sustainability and its ability to adapt to market changes will likely drive its financial success in the years to come. The company's leadership in the energy transition and its strong financial performance make it a compelling investment opportunity for those seeking exposure to the growing renewable energy sector.
Overall, Shell's financial outlook is positive, with the company expected to continue its growth trajectory in the coming years. Its strong financial performance, strategic investments, and commitment to sustainability position it well to capitalize on market opportunities and deliver value to shareholders.
Rating | Short-Term | Long-Term Senior |
---|---|---|
Outlook* | B2 | Ba1 |
Income Statement | Ba1 | B1 |
Balance Sheet | Baa2 | Ba3 |
Leverage Ratios | C | Baa2 |
Cash Flow | C | Baa2 |
Rates of Return and Profitability | Caa2 | B3 |
*Financial analysis is the process of evaluating a company's financial performance and position by neural network. It involves reviewing the company's financial statements, including the balance sheet, income statement, and cash flow statement, as well as other financial reports and documents.
How does neural network examine financial reports and understand financial state of the company?This exclusive content is only available to premium users.This exclusive content is only available to premium users.
Shell's Operating Efficiency: A Path to Enhanced Performance
Shell, a global energy giant, prioritizes operational efficiency to optimize its performance and maintain a competitive edge. The company has implemented various initiatives to streamline processes, reduce costs, and enhance overall productivity. These efforts have resulted in significant improvements in key operating metrics, such as asset utilization, energy consumption, and production efficiency.
One aspect of Shell's efficiency drive involves digital transformation and technology adoption. The company has invested in digital tools, automation, and data analytics to optimize its operations. These technologies enable Shell to monitor and control assets remotely, perform predictive maintenance, and make data-driven decisions. Additionally, Shell has implemented a digital twin strategy to create virtual replicas of its physical infrastructure, allowing it to simulate and optimize operations before implementation.
Another key focus area for Shell's efficiency efforts is talent development and employee engagement. The company invests heavily in training and upskilling its workforce to ensure that employees possess the necessary competencies to operate and maintain assets effectively. Shell also promotes a culture of continuous improvement, where employees are encouraged to identify and implement process enhancements.
Shell's commitment to operating efficiency extends beyond its own operations. The company collaborates with suppliers and partners to improve efficiency throughout the value chain. By working together, Shell and its stakeholders can identify and eliminate inefficiencies, leading to cost savings and improved overall competitiveness. Looking ahead, Shell is expected to continue its focus on operational efficiency as it strives to maintain its position as a global energy leader. By embracing innovative technologies, investing in its people, and fostering collaboration, Shell can further enhance its performance and deliver value to stakeholders.
Shell's Comprehensive Risk Assessment
Shell, a global energy giant, places paramount importance on risk assessment to safeguard its operations, employees, and stakeholders. The company employs a multifaceted approach that encompasses identifying, analyzing, evaluating, and mitigating potential risks across various domains, including environmental, operational, financial, and reputational.
Shell utilizes industry-leading methodologies and tools, such as the ISO 31000 risk management framework, to conduct thorough risk assessments. The process involves identifying and categorizing potential risks, assessing their likelihood and impact, and developing and implementing appropriate mitigation strategies. Shell engages with a wide range of stakeholders, including regulators, contractors, and local communities, to gather insights and ensure a comprehensive understanding of potential risks.
To ensure the effectiveness of its risk assessment program, Shell regularly reviews and updates its approach. The company leverages advancements in technology, such as data analytics and artificial intelligence, to enhance its risk identification and mitigation capabilities. Furthermore, Shell conducts regular training and awareness campaigns for its employees to foster a culture of risk awareness and responsibility throughout the organization.
Through its rigorous risk assessment practices, Shell aims to minimize uncertainties, manage potential threats, and enhance its overall resilience. The company's commitment to robust risk management is pivotal in maintaining safe operations, protecting its assets and reputation, and fulfilling its obligations to stakeholders. By continuously improving its risk assessment processes, Shell strives to remain a leader in the energy industry and contribute to a sustainable and responsible future.
References
- Barkan O. 2016. Bayesian neural word embedding. arXiv:1603.06571 [math.ST]
- S. Devlin, L. Yliniemi, D. Kudenko, and K. Tumer. Potential-based difference rewards for multiagent reinforcement learning. In Proceedings of the Thirteenth International Joint Conference on Autonomous Agents and Multiagent Systems, May 2014
- V. Borkar. Q-learning for risk-sensitive control. Mathematics of Operations Research, 27:294–311, 2002.
- P. Artzner, F. Delbaen, J. Eber, and D. Heath. Coherent measures of risk. Journal of Mathematical Finance, 9(3):203–228, 1999
- Krizhevsky A, Sutskever I, Hinton GE. 2012. Imagenet classification with deep convolutional neural networks. In Advances in Neural Information Processing Systems, Vol. 25, ed. Z Ghahramani, M Welling, C Cortes, ND Lawrence, KQ Weinberger, pp. 1097–105. San Diego, CA: Neural Inf. Process. Syst. Found.
- Tibshirani R. 1996. Regression shrinkage and selection via the lasso. J. R. Stat. Soc. B 58:267–88
- Challen, D. W. A. J. Hagger (1983), Macroeconomic Systems: Construction, Validation and Applications. New York: St. Martin's Press.