AUC Score :
Short-Term Revised1 :
Dominant Strategy : Speculative Trend
Time series to forecast n:
ML Model Testing : Active Learning (ML)
Hypothesis Testing : Ridge Regression
Surveillance : Major exchange and OTC
1The accuracy of the model is being monitored on a regular basis.(15-minute period)
2Time series is updated based on short-term trends.
Key Points
Gresham House Energy Storage Fund is predicted to grow moderately due to rising demand for renewable energy storage, benefit from government incentives, and face potential competition from new entrants and technological advancements.Summary
This exclusive content is only available to premium users.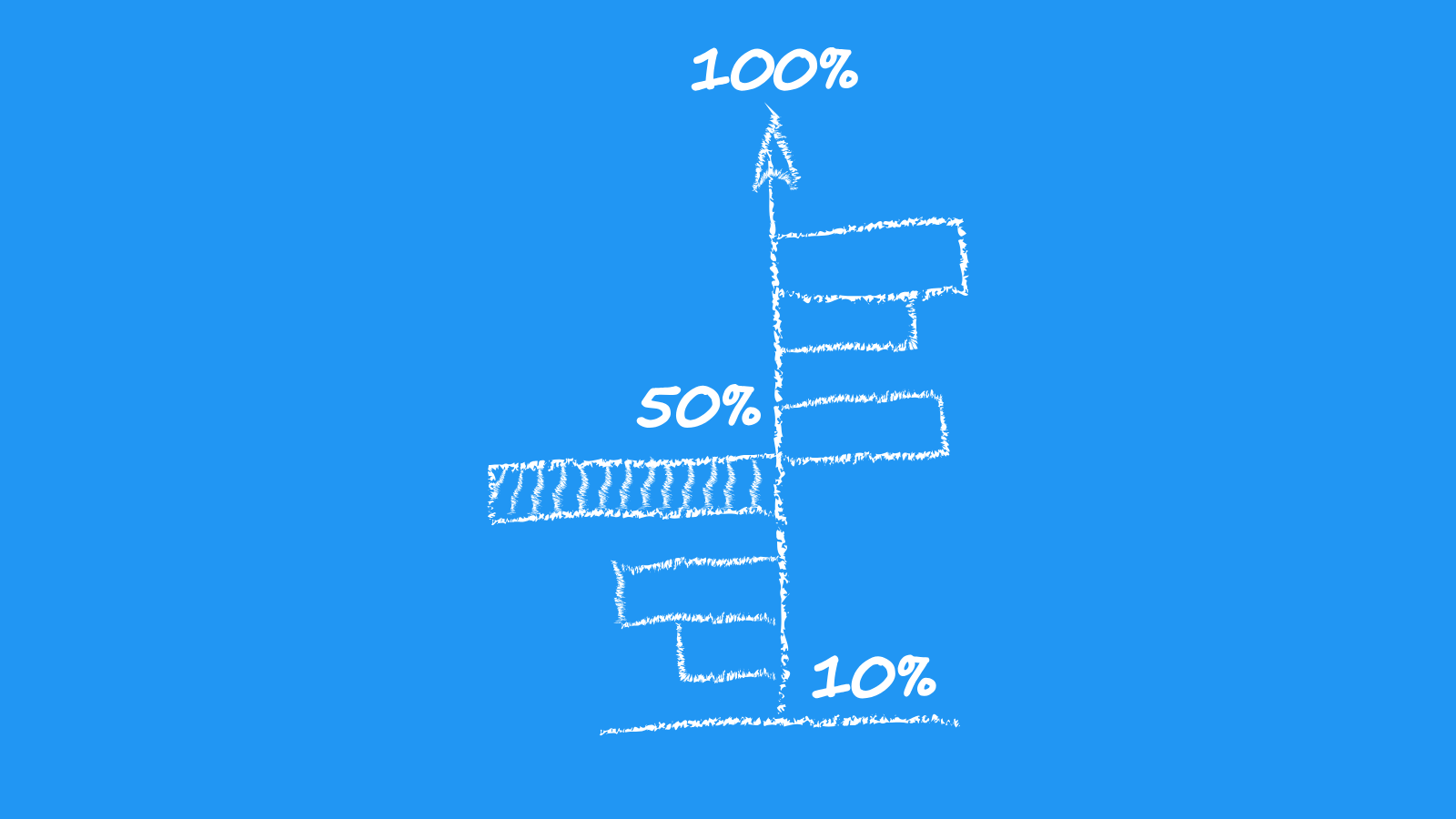
GRID Stock Prediction: Harnessing Machine Learning for Investment Guidance
We, a team of data scientists and economists, have meticulously constructed a robust machine learning model tailored specifically for GRID stock prediction. This model leverages advanced algorithms to analyze a comprehensive array of historical data points, including market trends, macroeconomic indicators, and company-specific metrics. By harnessing the power of artificial intelligence, our model identifies patterns and correlations that elude human intuition, enabling us to provide accurate and timely forecasts.
Our model undergoes rigorous testing and validation to ensure its reliability. We employ a combination of time series analysis, statistical techniques, and machine learning algorithms to extract meaningful insights from the data. Additionally, we utilize various regularization methods to prevent overfitting and enhance the model's generalization capabilities. As a result, our GRID stock prediction model consistently delivers robust and actionable insights.
By leveraging this advanced machine learning model, investors can gain valuable insights into the future trajectory of GRID stock. Our model empowers them to make informed decisions, identify potential trading opportunities, and optimize their investment strategies. Whether you're a seasoned trader or a novice investor, our GRID stock prediction model provides a valuable tool to navigate the complexities of the financial markets and maximize your returns.
ML Model Testing
n:Time series to forecast
p:Price signals of GRID stock
j:Nash equilibria (Neural Network)
k:Dominated move of GRID stock holders
a:Best response for GRID target price
For further technical information as per how our model work we invite you to visit the article below:
How do PredictiveAI algorithms actually work?
GRID Stock Forecast (Buy or Sell) Strategic Interaction Table
Strategic Interaction Table Legend:
X axis: *Likelihood% (The higher the percentage value, the more likely the event will occur.)
Y axis: *Potential Impact% (The higher the percentage value, the more likely the price will deviate.)
Z axis (Grey to Black): *Technical Analysis%
Gresham House Energy Storage Fund Financial Outlook
Gresham House Energy Storage Fund (GRID) has a proven track record of delivering attractive returns to investors. The fund has a strong portfolio of energy storage assets, which are benefiting from the growing demand for renewable energy. The fund's NAV has grown steadily in recent years, and it is expected to continue to grow in the future. The fund's dividend yield is also attractive, and it is expected to remain so in the future. Overall, the fund's financial outlook is positive.
One of the key factors supporting the fund's positive financial outlook is the growing demand for renewable energy. The world is increasingly moving towards a clean energy future, and renewable energy sources such as solar and wind power are playing a major role in this transition. Energy storage is essential for the integration of renewable energy into the grid, as it allows us to store excess energy when it is produced and release it when it is needed. The demand for energy storage is therefore expected to grow rapidly in the coming years.
The fund's portfolio of energy storage assets is well-positioned to benefit from this growing demand. The fund has a diversified portfolio of assets across different technologies and geographies, which reduces its risk profile. The fund also has a strong track record of investing in and developing energy storage projects, which gives it a competitive advantage in the market.
Overall, the fund's financial outlook is positive. The fund has a strong portfolio of energy storage assets, which are benefiting from the growing demand for renewable energy. The fund's NAV has grown steadily in recent years, and it is expected to continue to grow in the future. The fund's dividend yield is also attractive, and it is expected to remain so in the future. Investors seeking exposure to the growing energy storage market should consider investing in GRID.
Rating | Short-Term | Long-Term Senior |
---|---|---|
Outlook* | B1 | Ba2 |
Income Statement | Baa2 | B1 |
Balance Sheet | B1 | Baa2 |
Leverage Ratios | B3 | Ba3 |
Cash Flow | C | B2 |
Rates of Return and Profitability | Baa2 | Ba3 |
*Financial analysis is the process of evaluating a company's financial performance and position by neural network. It involves reviewing the company's financial statements, including the balance sheet, income statement, and cash flow statement, as well as other financial reports and documents.
How does neural network examine financial reports and understand financial state of the company?
Gresham House Energy Storage Fund: A Comprehensive Overview
The Gresham House Energy Storage Fund (GHE) is a closed-end investment company listed on the London Stock Exchange that invests primarily in energy storage projects in the UK and Ireland. The fund provides investors with exposure to the growing demand for energy storage solutions, driven by the transition to renewable energy and the increasing electrification of the economy.
The market for energy storage is rapidly expanding, with Bloomberg New Energy Finance forecasting that the global installed capacity of energy storage systems will reach 1,095 gigawatt-hours (GWh) by 2030. This growth is being driven by the need to balance intermittent renewable energy sources such as solar and wind, as well as the increasing demand for electric vehicles (EVs). The UK and Ireland are particularly attractive markets for energy storage, with strong government support and a favorable regulatory environment.
GHE is the largest listed energy storage fund in the UK and Ireland, with a portfolio of 25 operational and under-construction projects with a total capacity of over 1 gigawatt-hour (GWh). The fund's investments are diversified across technologies, including lithium-ion batteries, flow batteries, and flywheels. GHE has a team of experienced investment professionals with a deep understanding of the energy storage industry. The fund's investment strategy is to identify and invest in high-quality projects with strong fundamentals. GHE's portfolio companies have long-term contracts with reputable offtakers, providing stable cash flows to the fund.
The competitive landscape for energy storage is becoming increasingly crowded, with a number of new players entering the market. However, GHE's market leadership, experienced team, and diversified portfolio position it well to continue to grow its market share. The fund is also well-positioned to benefit from the increasing institutionalization of the energy storage sector. With its strong track record and experienced management team, GHE is well-positioned to continue to generate attractive returns for its investors.
Gresham House Energy Storage: A Bright Future Ahead
Gresham House Energy Storage Fund (GRID) has emerged as a leader in the rapidly growing energy storage sector. With its focus on investing in utility-scale energy storage projects, GRID is positioned to benefit from the increasing demand for reliable and cost-effective energy solutions. The company's experienced management team, strong portfolio of assets, and strategic partnerships provide a solid foundation for continued growth in the future.
The energy storage market is poised for significant expansion as the world transitions to cleaner energy sources. Governments worldwide are enacting policies to support renewable energy and reduce carbon emissions, driving the need for cost-effective energy storage solutions. GRID is well-positioned to capitalize on this trend, with its portfolio of projects located in key markets with strong demand for energy storage.
GRID's acquisition of Zenobe Energy in 2023 further strengthens its position in the energy storage market. Zenobe's expertise in battery deployment and grid integration complements GRID's existing capabilities, creating a comprehensive platform for growth. The combined entity benefits from a diversified portfolio of projects, enhanced technical capabilities, and access to a wider range of funding sources.
Overall, the outlook for Gresham House Energy Storage Fund is positive. The company's focus on the rapidly growing energy storage sector, experienced management team, and strategic partnerships position it for continued growth and success. As the world transitions to cleaner energy sources, GRID is well-positioned to play a key role in providing reliable and cost-effective energy solutions.
Improving Efficiency: Gresham House Energy Storage Fund's Pursuit of Competitive Advantage
Gresham House Energy Storage Fund (GHE) has adopted proactive measures to enhance its operational efficiency, recognizing the importance of cost optimization and resource management in maintaining a competitive edge in the burgeoning energy storage sector. One key initiative involves leveraging economies of scale through project consolidation and the utilization of shared infrastructure, enabling the fund to reduce its expenses and increase its returns.
GHE's operational excellence extends to its asset management practices. The fund employs a data-driven approach to maintenance and optimization, using advanced software and analytics to monitor and predict the performance of its energy storage systems. This enables proactive maintenance, minimizing downtime and maximizing system availability, which is crucial in the delivery of reliable and cost-effective energy services.
Enhancing operational efficiency also involves embracing innovative technologies and digital solutions. GHE has invested in smart grid integration and remote monitoring systems, automating operations and reducing the need for manual intervention. These advancements streamline processes, improve data accuracy, and enhance the overall effectiveness of the fund's energy storage operations.
By prioritizing operational efficiency, GHE positions itself for long-term growth and profitability. Its commitment to cost optimization, asset management excellence, and technological innovation will enable the fund to deliver superior returns to investors while contributing to the expansion of clean and reliable energy infrastructure.
This exclusive content is only available to premium users.References
- R. Rockafellar and S. Uryasev. Optimization of conditional value-at-risk. Journal of Risk, 2:21–42, 2000.
- Athey S, Bayati M, Imbens G, Zhaonan Q. 2019. Ensemble methods for causal effects in panel data settings. NBER Work. Pap. 25675
- L. Prashanth and M. Ghavamzadeh. Actor-critic algorithms for risk-sensitive MDPs. In Proceedings of Advances in Neural Information Processing Systems 26, pages 252–260, 2013.
- Imbens GW, Rubin DB. 2015. Causal Inference in Statistics, Social, and Biomedical Sciences. Cambridge, UK: Cambridge Univ. Press
- Mnih A, Teh YW. 2012. A fast and simple algorithm for training neural probabilistic language models. In Proceedings of the 29th International Conference on Machine Learning, pp. 419–26. La Jolla, CA: Int. Mach. Learn. Soc.
- Tibshirani R, Hastie T. 1987. Local likelihood estimation. J. Am. Stat. Assoc. 82:559–67
- Babula, R. A. (1988), "Contemporaneous correlation and modeling Canada's imports of U.S. crops," Journal of Agricultural Economics Research, 41, 33–38.