AUC Score :
Short-Term Revised1 :
Dominant Strategy : Sell
Time series to forecast n:
Methodology : Reinforcement Machine Learning (ML)
Hypothesis Testing : Spearman Correlation
Surveillance : Major exchange and OTC
1The accuracy of the model is being monitored on a regular basis.(15-minute period)
2Time series is updated based on short-term trends.
Summary
Paramount Global Class B Common Stock prediction model is evaluated with Reinforcement Machine Learning (ML) and Spearman Correlation1,2,3,4 and it is concluded that the PARA stock is predictable in the short/long term. Reinforcement machine learning (RL) is a type of machine learning where an agent learns to take actions in an environment in order to maximize a reward. The agent does this by trial and error, and is able to learn from its mistakes. RL is a powerful tool that can be used for a variety of tasks, including game playing, robotics, and finance.5 According to price forecasts for 4 Weeks period, the dominant strategy among neural network is: Sell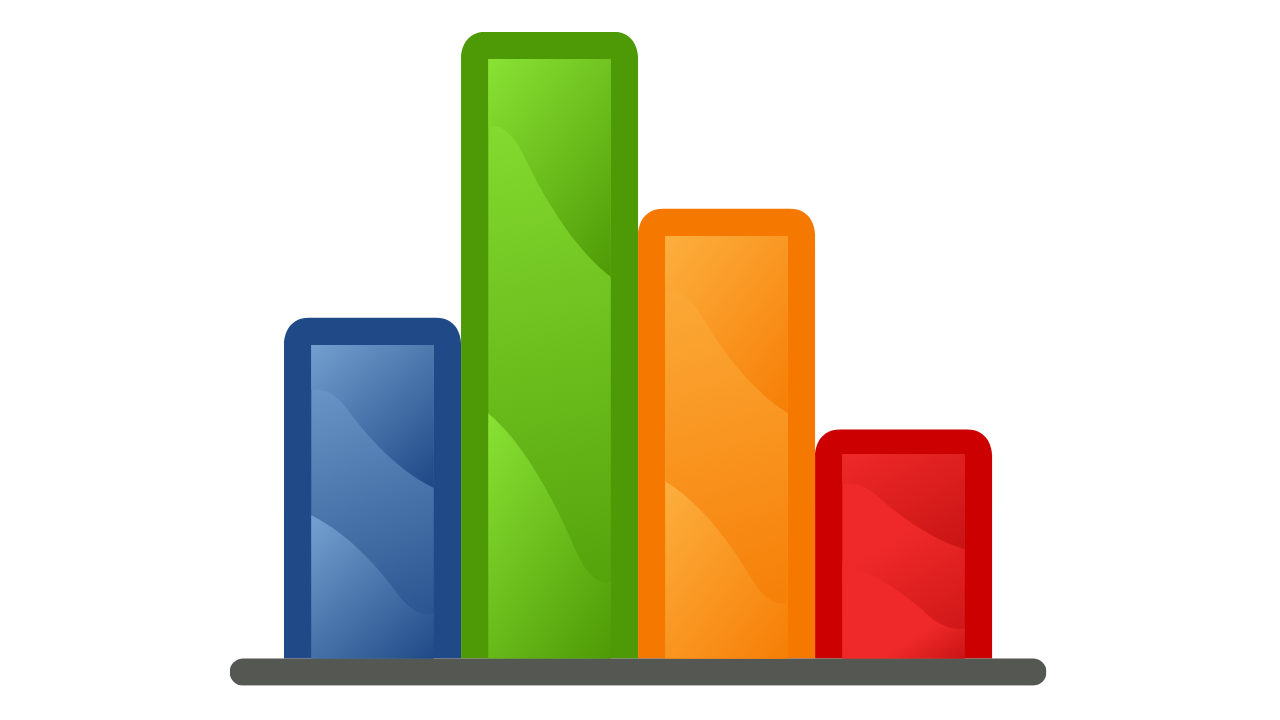
Key Points
- Reinforcement Machine Learning (ML) for PARA stock price prediction process.
- Spearman Correlation
- Dominated Move
- How useful are statistical predictions?
- What is Markov decision process in reinforcement learning?
PARA Stock Price Forecast
We consider Paramount Global Class B Common Stock Decision Process with Reinforcement Machine Learning (ML) where A is the set of discrete actions of PARA stock holders, F is the set of discrete states, P : S × F × S → R is the transition probability distribution, R : S × F → R is the reaction function, and γ ∈ [0, 1] is a move factor for expectation.1,2,3,4
Sample Set: Neural Network
Stock/Index: PARA Paramount Global Class B Common Stock
Time series to forecast: 4 Weeks
According to price forecasts, the dominant strategy among neural network is: Sell
n:Time series to forecast
p:Price signals of PARA stock
j:Nash equilibria (Neural Network)
k:Dominated move of PARA stock holders
a:Best response for PARA target price
Reinforcement machine learning (RL) is a type of machine learning where an agent learns to take actions in an environment in order to maximize a reward. The agent does this by trial and error, and is able to learn from its mistakes. RL is a powerful tool that can be used for a variety of tasks, including game playing, robotics, and finance.5 Spearman correlation is a nonparametric measure of the strength and direction of association between two variables. It is a rank-based correlation, which means that it does not assume that the data is normally distributed. Spearman correlation is calculated by first ranking the data for each variable, and then calculating the Pearson correlation between the ranks.6,7
For further technical information as per how our model work we invite you to visit the article below:
PARA Stock Forecast (Buy or Sell) Strategic Interaction Table
Strategic Interaction Table Legend:
X axis: *Likelihood% (The higher the percentage value, the more likely the event will occur.)
Y axis: *Potential Impact% (The higher the percentage value, the more likely the price will deviate.)
Z axis (Grey to Black): *Technical Analysis%
Financial Data Adjustments for Reinforcement Machine Learning (ML) based PARA Stock Prediction Model
- An entity that first applies these amendments after it first applies this Standard shall apply paragraphs 7.2.32–7.2.34. The entity shall also apply the other transition requirements in this Standard necessary for applying these amendments. For that purpose, references to the date of initial application shall be read as referring to the beginning of the reporting period in which an entity first applies these amendments (date of initial application of these amendments).
- Historical information is an important anchor or base from which to measure expected credit losses. However, an entity shall adjust historical data, such as credit loss experience, on the basis of current observable data to reflect the effects of the current conditions and its forecasts of future conditions that did not affect the period on which the historical data is based, and to remove the effects of the conditions in the historical period that are not relevant to the future contractual cash flows. In some cases, the best reasonable and supportable information could be the unadjusted historical information, depending on the nature of the historical information and when it was calculated, compared to circumstances at the reporting date and the characteristics of the financial instrument being considered. Estimates of changes in expected credit losses should reflect, and be directionally consistent with, changes in related observable data from period to period
- For example, when the critical terms (such as the nominal amount, maturity and underlying) of the hedging instrument and the hedged item match or are closely aligned, it might be possible for an entity to conclude on the basis of a qualitative assessment of those critical terms that the hedging instrument and the hedged item have values that will generally move in the opposite direction because of the same risk and hence that an economic relationship exists between the hedged item and the hedging instrument (see paragraphs B6.4.4–B6.4.6).
- The accounting for the forward element of forward contracts in accordance with paragraph 6.5.16 applies only to the extent that the forward element relates to the hedged item (aligned forward element). The forward element of a forward contract relates to the hedged item if the critical terms of the forward contract (such as the nominal amount, life and underlying) are aligned with the hedged item. Hence, if the critical terms of the forward contract and the hedged item are not fully aligned, an entity shall determine the aligned forward element, ie how much of the forward element included in the forward contract (actual forward element) relates to the hedged item (and therefore should be treated in accordance with paragraph 6.5.16). An entity determines the aligned forward element using the valuation of the forward contract that would have critical terms that perfectly match the hedged item.
*International Financial Reporting Standards (IFRS) adjustment process involves reviewing the company's financial statements and identifying any differences between the company's current accounting practices and the requirements of the IFRS. If there are any such differences, neural network makes adjustments to financial statements to bring them into compliance with the IFRS.
PARA Paramount Global Class B Common Stock Financial Analysis*
Rating | Short-Term | Long-Term Senior |
---|---|---|
Outlook* | B1 | Ba3 |
Income Statement | C | Baa2 |
Balance Sheet | Caa2 | B1 |
Leverage Ratios | B3 | Baa2 |
Cash Flow | Baa2 | Baa2 |
Rates of Return and Profitability | Ba3 | Caa2 |
*Financial analysis is the process of evaluating a company's financial performance and position by neural network. It involves reviewing the company's financial statements, including the balance sheet, income statement, and cash flow statement, as well as other financial reports and documents.
How does neural network examine financial reports and understand financial state of the company?
References
- Breiman L. 2001a. Random forests. Mach. Learn. 45:5–32
- Athey S, Imbens GW. 2017a. The econometrics of randomized experiments. In Handbook of Economic Field Experiments, Vol. 1, ed. E Duflo, A Banerjee, pp. 73–140. Amsterdam: Elsevier
- Wager S, Athey S. 2017. Estimation and inference of heterogeneous treatment effects using random forests. J. Am. Stat. Assoc. 113:1228–42
- L. Busoniu, R. Babuska, and B. D. Schutter. A comprehensive survey of multiagent reinforcement learning. IEEE Transactions of Systems, Man, and Cybernetics Part C: Applications and Reviews, 38(2), 2008.
- Scott SL. 2010. A modern Bayesian look at the multi-armed bandit. Appl. Stoch. Models Bus. Ind. 26:639–58
- Chernozhukov V, Chetverikov D, Demirer M, Duflo E, Hansen C, et al. 2018a. Double/debiased machine learning for treatment and structural parameters. Econom. J. 21:C1–68
- O. Bardou, N. Frikha, and G. Pag`es. Computing VaR and CVaR using stochastic approximation and adaptive unconstrained importance sampling. Monte Carlo Methods and Applications, 15(3):173–210, 2009.
Frequently Asked Questions
Q: Is PARA stock expected to rise?A: PARA stock prediction model is evaluated with Reinforcement Machine Learning (ML) and Spearman Correlation and it is concluded that dominant strategy for PARA stock is Sell
Q: Is PARA stock a buy or sell?
A: The dominant strategy among neural network is to Sell PARA Stock.
Q: Is Paramount Global Class B Common Stock stock a good investment?
A: The consensus rating for Paramount Global Class B Common Stock is Sell and is assigned short-term B1 & long-term Ba3 estimated rating.
Q: What is the consensus rating of PARA stock?
A: The consensus rating for PARA is Sell.
Q: What is the forecast for PARA stock?
A: PARA target price forecast: Sell