AUC Score :
Short-Term Revised1 :
Dominant Strategy : Buy
Time series to forecast n:
Methodology : Modular Neural Network (News Feed Sentiment Analysis)
Hypothesis Testing : Factor
Surveillance : Major exchange and OTC
1The accuracy of the model is being monitored on a regular basis.(15-minute period)
2Time series is updated based on short-term trends.
Summary
Barfresh Food Group Inc. Common Stock prediction model is evaluated with Modular Neural Network (News Feed Sentiment Analysis) and Factor1,2,3,4 and it is concluded that the BRFH stock is predictable in the short/long term. A modular neural network (MNN) is a type of artificial neural network that can be used for news feed sentiment analysis. MNNs are made up of multiple smaller neural networks, called modules. Each module is responsible for learning a specific task, such as identifying sentiment in text or identifying patterns in data. The modules are then combined to form a single neural network that can perform multiple tasks. In the context of news feed sentiment analysis, MNNs can be used to identify the sentiment of news articles, social media posts, and other forms of online content. This information can then be used to filter out irrelevant or unwanted content, to identify trends in public opinion, and to target users with relevant advertising.5 According to price forecasts for 4 Weeks period, the dominant strategy among neural network is: Buy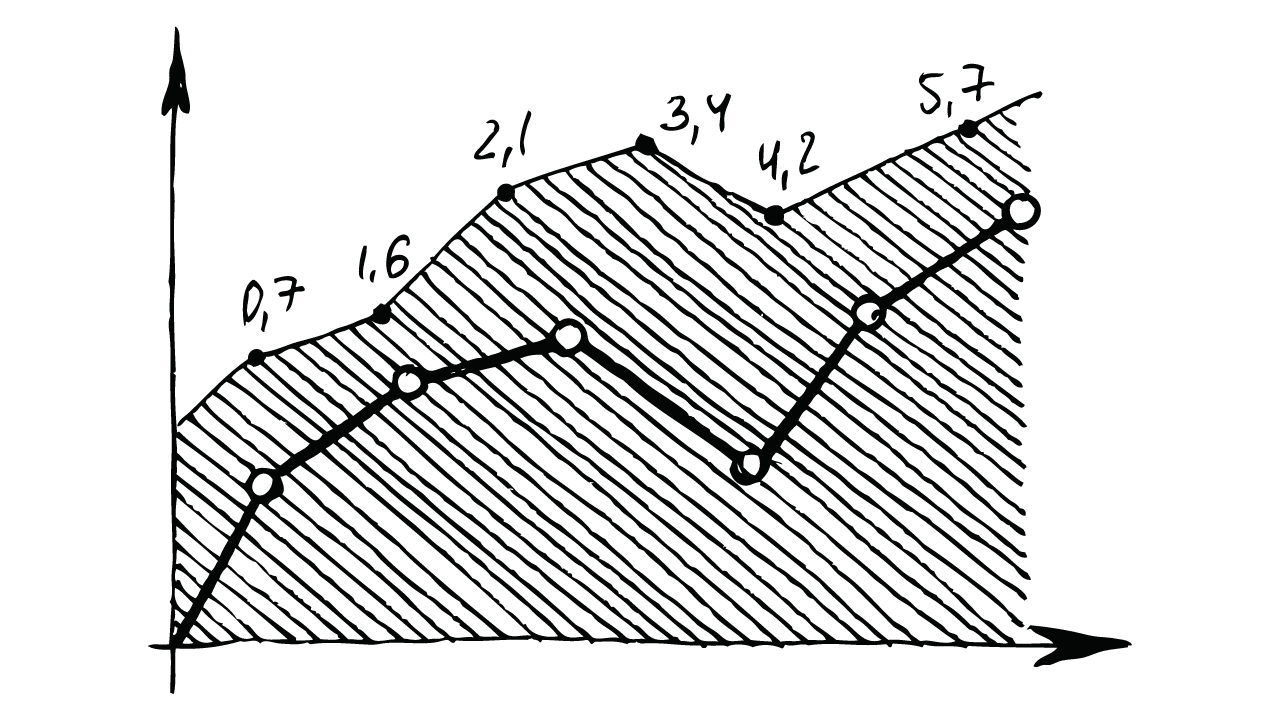
Key Points
- Modular Neural Network (News Feed Sentiment Analysis) for BRFH stock price prediction process.
- Factor
- Should I buy stocks now or wait amid such uncertainty?
- Can neural networks predict stock market?
- What is the best way to predict stock prices?
BRFH Stock Price Forecast
We consider Barfresh Food Group Inc. Common Stock Decision Process with Modular Neural Network (News Feed Sentiment Analysis) where A is the set of discrete actions of BRFH stock holders, F is the set of discrete states, P : S × F × S → R is the transition probability distribution, R : S × F → R is the reaction function, and γ ∈ [0, 1] is a move factor for expectation.1,2,3,4
Sample Set: Neural Network
Stock/Index: BRFH Barfresh Food Group Inc. Common Stock
Time series to forecast: 4 Weeks
According to price forecasts, the dominant strategy among neural network is: Buy
n:Time series to forecast
p:Price signals of BRFH stock
j:Nash equilibria (Neural Network)
k:Dominated move of BRFH stock holders
a:Best response for BRFH target price
A modular neural network (MNN) is a type of artificial neural network that can be used for news feed sentiment analysis. MNNs are made up of multiple smaller neural networks, called modules. Each module is responsible for learning a specific task, such as identifying sentiment in text or identifying patterns in data. The modules are then combined to form a single neural network that can perform multiple tasks. In the context of news feed sentiment analysis, MNNs can be used to identify the sentiment of news articles, social media posts, and other forms of online content. This information can then be used to filter out irrelevant or unwanted content, to identify trends in public opinion, and to target users with relevant advertising.5 In statistics, a factor is a variable that can influence the value of another variable. Factors can be categorical or continuous. Categorical factors have a limited number of possible values, such as gender (male or female) or blood type (A, B, AB, or O). Continuous factors can have an infinite number of possible values, such as height or weight. Factors can be used to explain the variation in a dependent variable. For example, a study might find that there is a relationship between gender and height. In this case, gender would be the independent variable, height would be the dependent variable, and the factor would be gender.6,7
For further technical information as per how our model work we invite you to visit the article below:
BRFH Stock Forecast (Buy or Sell) Strategic Interaction Table
Strategic Interaction Table Legend:
X axis: *Likelihood% (The higher the percentage value, the more likely the event will occur.)
Y axis: *Potential Impact% (The higher the percentage value, the more likely the price will deviate.)
Z axis (Grey to Black): *Technical Analysis%
Financial Data Adjustments for Modular Neural Network (News Feed Sentiment Analysis) based BRFH Stock Prediction Model
- An entity may retain the right to a part of the interest payments on transferred assets as compensation for servicing those assets. The part of the interest payments that the entity would give up upon termination or transfer of the servicing contract is allocated to the servicing asset or servicing liability. The part of the interest payments that the entity would not give up is an interest-only strip receivable. For example, if the entity would not give up any interest upon termination or transfer of the servicing contract, the entire interest spread is an interest-only strip receivable. For the purposes of applying paragraph 3.2.13, the fair values of the servicing asset and interest-only strip receivable are used to allocate the carrying amount of the receivable between the part of the asset that is derecognised and the part that continues to be recognised. If there is no servicing fee specified or the fee to be received is not expected to compensate the entity adequately for performing the servicing, a liability for the servicing obligation is recognised at fair value.
- When a group of items that constitute a net position is designated as a hedged item, an entity shall designate the overall group of items that includes the items that can make up the net position. An entity is not permitted to designate a non-specific abstract amount of a net position. For example, an entity has a group of firm sale commitments in nine months' time for FC100 and a group of firm purchase commitments in 18 months' time for FC120. The entity cannot designate an abstract amount of a net position up to FC20. Instead, it must designate a gross amount of purchases and a gross amount of sales that together give rise to the hedged net position. An entity shall designate gross positions that give rise to the net position so that the entity is able to comply with the requirements for the accounting for qualifying hedging relationships.
- Paragraphs 6.9.7–6.9.13 provide exceptions to the requirements specified in those paragraphs only. An entity shall apply all other hedge accounting requirements in this Standard, including the qualifying criteria in paragraph 6.4.1, to hedging relationships that were directly affected by interest rate benchmark reform.
- IFRS 15, issued in May 2014, amended paragraphs 3.1.1, 4.2.1, 5.1.1, 5.2.1, 5.7.6, B3.2.13, B5.7.1, C5 and C42 and deleted paragraph C16 and its related heading. Paragraphs 5.1.3 and 5.7.1A, and a definition to Appendix A, were added. An entity shall apply those amendments when it applies IFRS 15.
*International Financial Reporting Standards (IFRS) adjustment process involves reviewing the company's financial statements and identifying any differences between the company's current accounting practices and the requirements of the IFRS. If there are any such differences, neural network makes adjustments to financial statements to bring them into compliance with the IFRS.
BRFH Barfresh Food Group Inc. Common Stock Financial Analysis*
Rating | Short-Term | Long-Term Senior |
---|---|---|
Outlook* | B1 | B3 |
Income Statement | Baa2 | B3 |
Balance Sheet | C | Caa2 |
Leverage Ratios | Ba3 | Baa2 |
Cash Flow | B1 | C |
Rates of Return and Profitability | B2 | C |
*Financial analysis is the process of evaluating a company's financial performance and position by neural network. It involves reviewing the company's financial statements, including the balance sheet, income statement, and cash flow statement, as well as other financial reports and documents.
How does neural network examine financial reports and understand financial state of the company?
References
- Chen X. 2007. Large sample sieve estimation of semi-nonparametric models. In Handbook of Econometrics, Vol. 6B, ed. JJ Heckman, EE Learner, pp. 5549–632. Amsterdam: Elsevier
- M. L. Littman. Markov games as a framework for multi-agent reinforcement learning. In Ma- chine Learning, Proceedings of the Eleventh International Conference, Rutgers University, New Brunswick, NJ, USA, July 10-13, 1994, pages 157–163, 1994
- V. Borkar. An actor-critic algorithm for constrained Markov decision processes. Systems & Control Letters, 54(3):207–213, 2005.
- Bessler, D. A. S. W. Fuller (1993), "Cointegration between U.S. wheat markets," Journal of Regional Science, 33, 481–501.
- Ruiz FJ, Athey S, Blei DM. 2017. SHOPPER: a probabilistic model of consumer choice with substitutes and complements. arXiv:1711.03560 [stat.ML]
- Nie X, Wager S. 2019. Quasi-oracle estimation of heterogeneous treatment effects. arXiv:1712.04912 [stat.ML]
- Arora S, Li Y, Liang Y, Ma T. 2016. RAND-WALK: a latent variable model approach to word embeddings. Trans. Assoc. Comput. Linguist. 4:385–99
Frequently Asked Questions
Q: Is BRFH stock expected to rise?A: BRFH stock prediction model is evaluated with Modular Neural Network (News Feed Sentiment Analysis) and Factor and it is concluded that dominant strategy for BRFH stock is Buy
Q: Is BRFH stock a buy or sell?
A: The dominant strategy among neural network is to Buy BRFH Stock.
Q: Is Barfresh Food Group Inc. Common Stock stock a good investment?
A: The consensus rating for Barfresh Food Group Inc. Common Stock is Buy and is assigned short-term B1 & long-term B3 estimated rating.
Q: What is the consensus rating of BRFH stock?
A: The consensus rating for BRFH is Buy.
Q: What is the forecast for BRFH stock?
A: BRFH target price forecast: Buy