AUC Score :
Short-Term Revised1 :
Dominant Strategy : Sell
Time series to forecast n:
Methodology : Multi-Instance Learning (ML)
Hypothesis Testing : Linear Regression
Surveillance : Major exchange and OTC
1The accuracy of the model is being monitored on a regular basis.(15-minute period)
2Time series is updated based on short-term trends.
Summary
GSE Systems Inc. Common Stock prediction model is evaluated with Multi-Instance Learning (ML) and Linear Regression1,2,3,4 and it is concluded that the GVP stock is predictable in the short/long term. Multi-instance learning (MIL) is a machine learning (ML) problem where a dataset consists of multiple instances, and each instance is associated with a single label. The goal of MIL is to learn a model that can predict the label of a new instance based on the labels of the instances that it is similar to. MIL is a challenging problem because the instances in a dataset are not labeled individually. This means that the model cannot simply learn a mapping from the features of an instance to its label. Instead, the model must learn a way to combine the features of multiple instances to predict the label of a new instance.5 According to price forecasts for 16 Weeks period, the dominant strategy among neural network is: Sell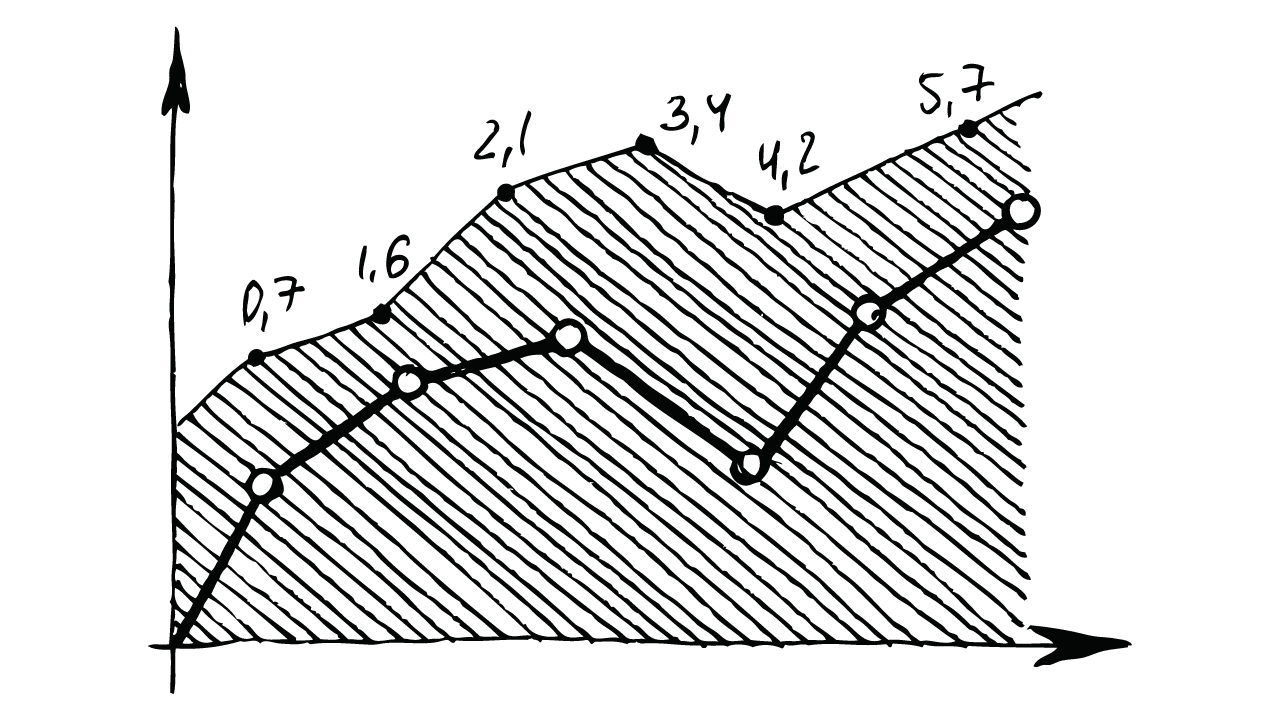
Key Points
- Multi-Instance Learning (ML) for GVP stock price prediction process.
- Linear Regression
- How do you pick a stock?
- Reaction Function
- What is prediction in deep learning?
GVP Stock Price Forecast
We consider GSE Systems Inc. Common Stock Decision Process with Multi-Instance Learning (ML) where A is the set of discrete actions of GVP stock holders, F is the set of discrete states, P : S × F × S → R is the transition probability distribution, R : S × F → R is the reaction function, and γ ∈ [0, 1] is a move factor for expectation.1,2,3,4
Sample Set: Neural Network
Stock/Index: GVP GSE Systems Inc. Common Stock
Time series to forecast: 16 Weeks
According to price forecasts, the dominant strategy among neural network is: Sell
n:Time series to forecast
p:Price signals of GVP stock
j:Nash equilibria (Neural Network)
k:Dominated move of GVP stock holders
a:Best response for GVP target price
Multi-instance learning (MIL) is a machine learning (ML) problem where a dataset consists of multiple instances, and each instance is associated with a single label. The goal of MIL is to learn a model that can predict the label of a new instance based on the labels of the instances that it is similar to. MIL is a challenging problem because the instances in a dataset are not labeled individually. This means that the model cannot simply learn a mapping from the features of an instance to its label. Instead, the model must learn a way to combine the features of multiple instances to predict the label of a new instance.5 In statistics, linear regression is a method for estimating the relationship between a dependent variable and one or more independent variables. The dependent variable is the variable that is being predicted, and the independent variables are the variables that are used to predict the dependent variable. Linear regression assumes that the relationship between the dependent variable and the independent variables is linear. This means that the dependent variable can be represented as a straight line function of the independent variables.6,7
For further technical information as per how our model work we invite you to visit the article below:
GVP Stock Forecast (Buy or Sell) Strategic Interaction Table
Strategic Interaction Table Legend:
X axis: *Likelihood% (The higher the percentage value, the more likely the event will occur.)
Y axis: *Potential Impact% (The higher the percentage value, the more likely the price will deviate.)
Z axis (Grey to Black): *Technical Analysis%
Financial Data Adjustments for Multi-Instance Learning (ML) based GVP Stock Prediction Model
- The fair value of a financial instrument at initial recognition is normally the transaction price (ie the fair value of the consideration given or received, see also paragraph B5.1.2A and IFRS 13). However, if part of the consideration given or received is for something other than the financial instrument, an entity shall measure the fair value of the financial instrument. For example, the fair value of a long-term loan or receivable that carries no interest can be measured as the present value of all future cash receipts discounted using the prevailing market rate(s) of interest for a similar instrument (similar as to currency, term, type of interest rate and other factors) with a similar credit rating. Any additional amount lent is an expense or a reduction of income unless it qualifies for recognition as some other type of asset.
- An entity shall apply this Standard for annual periods beginning on or after 1 January 2018. Earlier application is permitted. If an entity elects to apply this Standard early, it must disclose that fact and apply all of the requirements in this Standard at the same time (but see also paragraphs 7.1.2, 7.2.21 and 7.3.2). It shall also, at the same time, apply the amendments in Appendix C.
- When an entity, consistent with its hedge documentation, frequently resets (ie discontinues and restarts) a hedging relationship because both the hedging instrument and the hedged item frequently change (ie the entity uses a dynamic process in which both the hedged items and the hedging instruments used to manage that exposure do not remain the same for long), the entity shall apply the requirement in paragraphs 6.3.7(a) and B6.3.8—that the risk component is separately identifiable—only when it initially designates a hedged item in that hedging relationship. A hedged item that has been assessed at the time of its initial designation in the hedging relationship, whether it was at the time of the hedge inception or subsequently, is not reassessed at any subsequent redesignation in the same hedging relationship.
- For some types of fair value hedges, the objective of the hedge is not primarily to offset the fair value change of the hedged item but instead to transform the cash flows of the hedged item. For example, an entity hedges the fair value interest rate risk of a fixed-rate debt instrument using an interest rate swap. The entity's hedge objective is to transform the fixed-interest cash flows into floating interest cash flows. This objective is reflected in the accounting for the hedging relationship by accruing the net interest accrual on the interest rate swap in profit or loss. In the case of a hedge of a net position (for example, a net position of a fixed-rate asset and a fixed-rate liability), this net interest accrual must be presented in a separate line item in the statement of profit or loss and other comprehensive income. This is to avoid the grossing up of a single instrument's net gains or losses into offsetting gross amounts and recognising them in different line items (for example, this avoids grossing up a net interest receipt on a single interest rate swap into gross interest revenue and gross interest expense).
*International Financial Reporting Standards (IFRS) adjustment process involves reviewing the company's financial statements and identifying any differences between the company's current accounting practices and the requirements of the IFRS. If there are any such differences, neural network makes adjustments to financial statements to bring them into compliance with the IFRS.
GVP GSE Systems Inc. Common Stock Financial Analysis*
Rating | Short-Term | Long-Term Senior |
---|---|---|
Outlook* | B3 | Ba3 |
Income Statement | Caa2 | B2 |
Balance Sheet | C | Baa2 |
Leverage Ratios | Ba1 | B2 |
Cash Flow | Caa2 | Caa2 |
Rates of Return and Profitability | B3 | B2 |
*Financial analysis is the process of evaluating a company's financial performance and position by neural network. It involves reviewing the company's financial statements, including the balance sheet, income statement, and cash flow statement, as well as other financial reports and documents.
How does neural network examine financial reports and understand financial state of the company?
References
- L. Busoniu, R. Babuska, and B. D. Schutter. A comprehensive survey of multiagent reinforcement learning. IEEE Transactions of Systems, Man, and Cybernetics Part C: Applications and Reviews, 38(2), 2008.
- R. Williams. Simple statistical gradient-following algorithms for connectionist reinforcement learning. Ma- chine learning, 8(3-4):229–256, 1992
- Athey S, Wager S. 2017. Efficient policy learning. arXiv:1702.02896 [math.ST]
- J. G. Schneider, W. Wong, A. W. Moore, and M. A. Riedmiller. Distributed value functions. In Proceedings of the Sixteenth International Conference on Machine Learning (ICML 1999), Bled, Slovenia, June 27 - 30, 1999, pages 371–378, 1999.
- T. Morimura, M. Sugiyama, M. Kashima, H. Hachiya, and T. Tanaka. Nonparametric return distribution ap- proximation for reinforcement learning. In Proceedings of the 27th International Conference on Machine Learning, pages 799–806, 2010
- V. Borkar. Q-learning for risk-sensitive control. Mathematics of Operations Research, 27:294–311, 2002.
- J. Filar, D. Krass, and K. Ross. Percentile performance criteria for limiting average Markov decision pro- cesses. IEEE Transaction of Automatic Control, 40(1):2–10, 1995.
Frequently Asked Questions
Q: Is GVP stock expected to rise?A: GVP stock prediction model is evaluated with Multi-Instance Learning (ML) and Linear Regression and it is concluded that dominant strategy for GVP stock is Sell
Q: Is GVP stock a buy or sell?
A: The dominant strategy among neural network is to Sell GVP Stock.
Q: Is GSE Systems Inc. Common Stock stock a good investment?
A: The consensus rating for GSE Systems Inc. Common Stock is Sell and is assigned short-term B3 & long-term Ba3 estimated rating.
Q: What is the consensus rating of GVP stock?
A: The consensus rating for GVP is Sell.
Q: What is the forecast for GVP stock?
A: GVP target price forecast: Sell