AUC Score :
Short-Term Revised1 :
Dominant Strategy : Speculative Trend
Time series to forecast n:
Methodology : Modular Neural Network (DNN Layer)
Hypothesis Testing : Logistic Regression
Surveillance : Major exchange and OTC
1The accuracy of the model is being monitored on a regular basis.(15-minute period)
2Time series is updated based on short-term trends.
Summary
Alvotech Ordinary Shares prediction model is evaluated with Modular Neural Network (DNN Layer) and Logistic Regression1,2,3,4 and it is concluded that the ALVO stock is predictable in the short/long term. In a modular neural network (MNN), a DNN layer is a type of module that is used to learn complex relationships between input and output data. DNN layers are made up of a series of artificial neurons, which are connected to each other by weighted edges. The weights of the edges are adjusted during training to minimize the error between the network's predictions and the desired output. DNN layers are used in a variety of MNN applications, including natural language processing, speech recognition, and machine translation. In natural language processing, DNN layers are used to extract features from text data, such as the sentiment of a sentence or the topic of a conversation. In speech recognition, DNN layers are used to convert audio data into text data. In machine translation, DNN layers are used to translate text from one language to another.5 According to price forecasts for 6 Month period, the dominant strategy among neural network is: Speculative Trend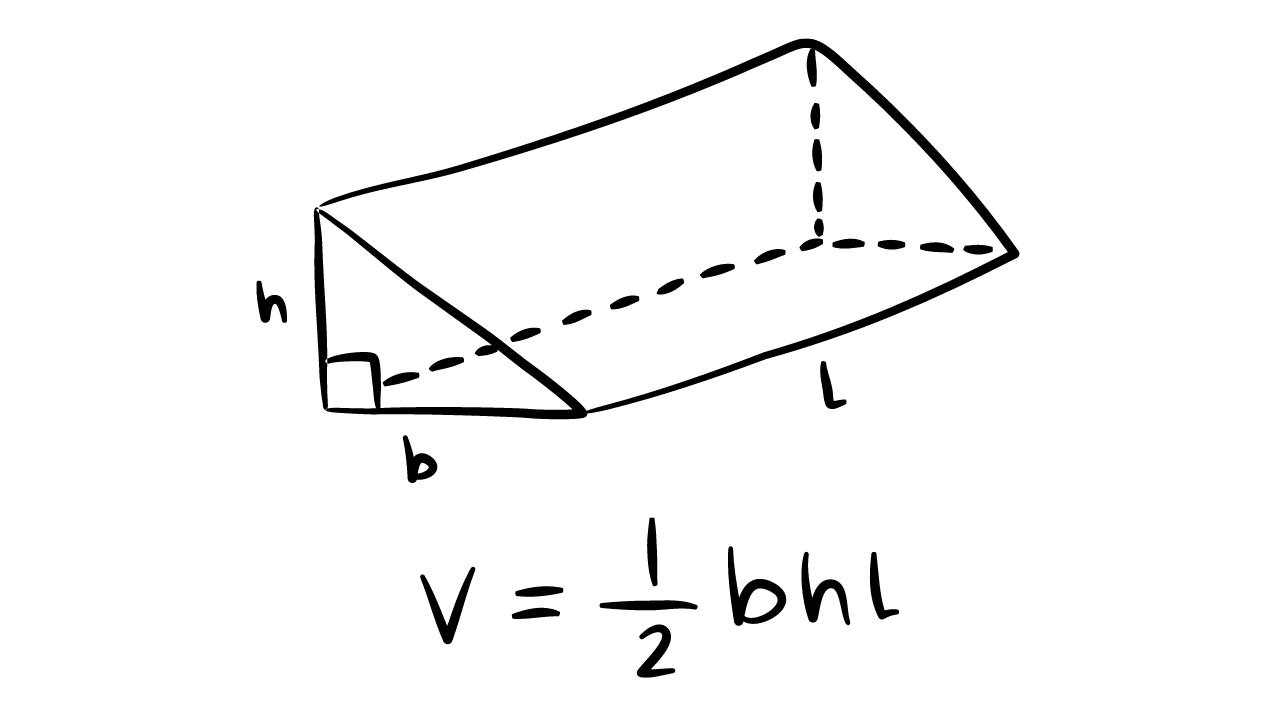
Key Points
- Modular Neural Network (DNN Layer) for ALVO stock price prediction process.
- Logistic Regression
- Can we predict stock market using machine learning?
- Can statistics predict the future?
- What is the best way to predict stock prices?
ALVO Stock Price Forecast
We consider Alvotech Ordinary Shares Decision Process with Modular Neural Network (DNN Layer) where A is the set of discrete actions of ALVO stock holders, F is the set of discrete states, P : S × F × S → R is the transition probability distribution, R : S × F → R is the reaction function, and γ ∈ [0, 1] is a move factor for expectation.1,2,3,4
Sample Set: Neural Network
Stock/Index: ALVO Alvotech Ordinary Shares
Time series to forecast: 6 Month
According to price forecasts, the dominant strategy among neural network is: Speculative Trend
n:Time series to forecast
p:Price signals of ALVO stock
j:Nash equilibria (Neural Network)
k:Dominated move of ALVO stock holders
a:Best response for ALVO target price
In a modular neural network (MNN), a DNN layer is a type of module that is used to learn complex relationships between input and output data. DNN layers are made up of a series of artificial neurons, which are connected to each other by weighted edges. The weights of the edges are adjusted during training to minimize the error between the network's predictions and the desired output. DNN layers are used in a variety of MNN applications, including natural language processing, speech recognition, and machine translation. In natural language processing, DNN layers are used to extract features from text data, such as the sentiment of a sentence or the topic of a conversation. In speech recognition, DNN layers are used to convert audio data into text data. In machine translation, DNN layers are used to translate text from one language to another.5 In statistics, logistic regression is a type of regression analysis used when the dependent variable is categorical. Logistic regression is a probability model that predicts the probability of an event occurring based on a set of independent variables. In logistic regression, the dependent variable is represented as a binary variable, such as "yes" or "no," "true" or "false," or "sick" or "healthy." The independent variables can be continuous or categorical variables.6,7
For further technical information as per how our model work we invite you to visit the article below:
ALVO Stock Forecast (Buy or Sell) Strategic Interaction Table
Strategic Interaction Table Legend:
X axis: *Likelihood% (The higher the percentage value, the more likely the event will occur.)
Y axis: *Potential Impact% (The higher the percentage value, the more likely the price will deviate.)
Z axis (Grey to Black): *Technical Analysis%
Financial Data Adjustments for Modular Neural Network (DNN Layer) based ALVO Stock Prediction Model
- An entity may use practical expedients when measuring expected credit losses if they are consistent with the principles in paragraph 5.5.17. An example of a practical expedient is the calculation of the expected credit losses on trade receivables using a provision matrix. The entity would use its historical credit loss experience (adjusted as appropriate in accordance with paragraphs B5.5.51–B5.5.52) for trade receivables to estimate the 12-month expected credit losses or the lifetime expected credit losses on the financial assets as relevant. A provision matrix might, for example, specify fixed provision rates depending on the number of days that a trade receivable is past due (for example, 1 per cent if not past due, 2 per cent if less than 30 days past due, 3 per cent if more than 30 days but less than 90 days past due, 20 per cent if 90–180 days past due etc). Depending on the diversity of its customer base, the entity would use appropriate groupings if its historical credit loss experience shows significantly different loss patterns for different customer segments. Examples of criteria that might be used to group assets include geographical region, product type, customer rating, collateral or trade credit insurance and type of customer (such as wholesale or retail)
- However, depending on the nature of the financial instruments and the credit risk information available for particular groups of financial instruments, an entity may not be able to identify significant changes in credit risk for individual financial instruments before the financial instrument becomes past due. This may be the case for financial instruments such as retail loans for which there is little or no updated credit risk information that is routinely obtained and monitored on an individual instrument until a customer breaches the contractual terms. If changes in the credit risk for individual financial instruments are not captured before they become past due, a loss allowance based only on credit information at an individual financial instrument level would not faithfully represent the changes in credit risk since initial recognition.
- The business model may be to hold assets to collect contractual cash flows even if the entity sells financial assets when there is an increase in the assets' credit risk. To determine whether there has been an increase in the assets' credit risk, the entity considers reasonable and supportable information, including forward looking information. Irrespective of their frequency and value, sales due to an increase in the assets' credit risk are not inconsistent with a business model whose objective is to hold financial assets to collect contractual cash flows because the credit quality of financial assets is relevant to the entity's ability to collect contractual cash flows. Credit risk management activities that are aimed at minimising potential credit losses due to credit deterioration are integral to such a business model. Selling a financial asset because it no longer meets the credit criteria specified in the entity's documented investment policy is an example of a sale that has occurred due to an increase in credit risk. However, in the absence of such a policy, the entity may demonstrate in other ways that the sale occurred due to an increase in credit risk.
- If a variable-rate financial liability bears interest of (for example) three-month LIBOR minus 20 basis points (with a floor at zero basis points), an entity can designate as the hedged item the change in the cash flows of that entire liability (ie three-month LIBOR minus 20 basis points—including the floor) that is attributable to changes in LIBOR. Hence, as long as the three-month LIBOR forward curve for the remaining life of that liability does not fall below 20 basis points, the hedged item has the same cash flow variability as a liability that bears interest at three-month LIBOR with a zero or positive spread. However, if the three-month LIBOR forward curve for the remaining life of that liability (or a part of it) falls below 20 basis points, the hedged item has a lower cash flow variability than a liability that bears interest at threemonth LIBOR with a zero or positive spread.
*International Financial Reporting Standards (IFRS) adjustment process involves reviewing the company's financial statements and identifying any differences between the company's current accounting practices and the requirements of the IFRS. If there are any such differences, neural network makes adjustments to financial statements to bring them into compliance with the IFRS.
ALVO Alvotech Ordinary Shares Financial Analysis*
Rating | Short-Term | Long-Term Senior |
---|---|---|
Outlook* | B2 | Ba2 |
Income Statement | Ba1 | Baa2 |
Balance Sheet | C | Caa2 |
Leverage Ratios | Caa2 | B3 |
Cash Flow | B2 | Ba2 |
Rates of Return and Profitability | Baa2 | Baa2 |
*Financial analysis is the process of evaluating a company's financial performance and position by neural network. It involves reviewing the company's financial statements, including the balance sheet, income statement, and cash flow statement, as well as other financial reports and documents.
How does neural network examine financial reports and understand financial state of the company?
References
- Bessler, D. A. T. Covey (1991), "Cointegration: Some results on U.S. cattle prices," Journal of Futures Markets, 11, 461–474.
- Bottou L. 2012. Stochastic gradient descent tricks. In Neural Networks: Tricks of the Trade, ed. G Montavon, G Orr, K-R Müller, pp. 421–36. Berlin: Springer
- Athey S, Imbens GW. 2017a. The econometrics of randomized experiments. In Handbook of Economic Field Experiments, Vol. 1, ed. E Duflo, A Banerjee, pp. 73–140. Amsterdam: Elsevier
- Knox SW. 2018. Machine Learning: A Concise Introduction. Hoboken, NJ: Wiley
- P. Artzner, F. Delbaen, J. Eber, and D. Heath. Coherent measures of risk. Journal of Mathematical Finance, 9(3):203–228, 1999
- Athey S, Imbens GW. 2017a. The econometrics of randomized experiments. In Handbook of Economic Field Experiments, Vol. 1, ed. E Duflo, A Banerjee, pp. 73–140. Amsterdam: Elsevier
- Arjovsky M, Bottou L. 2017. Towards principled methods for training generative adversarial networks. arXiv:1701.04862 [stat.ML]
Frequently Asked Questions
Q: Is ALVO stock expected to rise?A: ALVO stock prediction model is evaluated with Modular Neural Network (DNN Layer) and Logistic Regression and it is concluded that dominant strategy for ALVO stock is Speculative Trend
Q: Is ALVO stock a buy or sell?
A: The dominant strategy among neural network is to Speculative Trend ALVO Stock.
Q: Is Alvotech Ordinary Shares stock a good investment?
A: The consensus rating for Alvotech Ordinary Shares is Speculative Trend and is assigned short-term B2 & long-term Ba2 estimated rating.
Q: What is the consensus rating of ALVO stock?
A: The consensus rating for ALVO is Speculative Trend.
Q: What is the forecast for ALVO stock?
A: ALVO target price forecast: Speculative Trend