AUC Score :
Short-Term Revised1 :
Dominant Strategy : Sell
Time series to forecast n:
Methodology : Transductive Learning (ML)
Hypothesis Testing : Lasso Regression
Surveillance : Major exchange and OTC
1The accuracy of the model is being monitored on a regular basis.(15-minute period)
2Time series is updated based on short-term trends.
Abstract
LINDIAN RESOURCES LIMITED prediction model is evaluated with Transductive Learning (ML) and Lasso Regression1,2,3,4 and it is concluded that the LIN stock is predictable in the short/long term. Transductive learning is a supervised machine learning (ML) method in which the model is trained on both labeled and unlabeled data. The goal of transductive learning is to predict the labels of the unlabeled data. Transductive learning is a hybrid of inductive and semi-supervised learning. Inductive learning algorithms are trained on labeled data only, while semi-supervised learning algorithms are trained on a combination of labeled and unlabeled data. Transductive learning algorithms can achieve better performance than inductive learning algorithms on tasks where there is a small amount of labeled data. This is because transductive learning algorithms can use the unlabeled data to help them learn the relationships between the features and the labels. According to price forecasts for 4 Weeks period, the dominant strategy among neural network is: Sell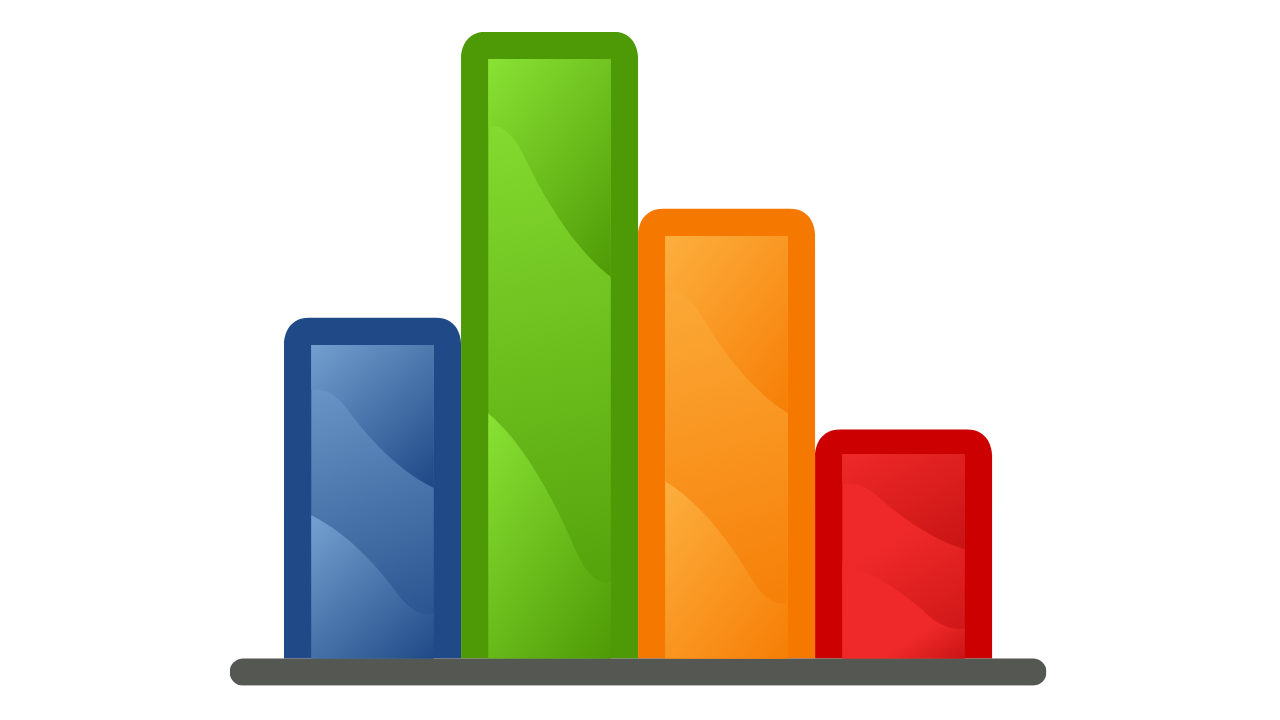
Key Points
- Dominated Move
- What is statistical models in machine learning?
- Why do we need predictive models?
LIN Target Price Prediction Modeling Methodology
We consider LINDIAN RESOURCES LIMITED Decision Process with Transductive Learning (ML) where A is the set of discrete actions of LIN stock holders, F is the set of discrete states, P : S × F × S → R is the transition probability distribution, R : S × F → R is the reaction function, and γ ∈ [0, 1] is a move factor for expectation.1,2,3,4
F(Lasso Regression)5,6,7= X R(Transductive Learning (ML)) X S(n):→ 4 Weeks
n:Time series to forecast
p:Price signals of LIN stock
j:Nash equilibria (Neural Network)
k:Dominated move
a:Best response for target price
Transductive Learning (ML)
Transductive learning is a supervised machine learning (ML) method in which the model is trained on both labeled and unlabeled data. The goal of transductive learning is to predict the labels of the unlabeled data. Transductive learning is a hybrid of inductive and semi-supervised learning. Inductive learning algorithms are trained on labeled data only, while semi-supervised learning algorithms are trained on a combination of labeled and unlabeled data. Transductive learning algorithms can achieve better performance than inductive learning algorithms on tasks where there is a small amount of labeled data. This is because transductive learning algorithms can use the unlabeled data to help them learn the relationships between the features and the labels.Lasso Regression
Lasso regression, also known as L1 regularization, is a type of regression analysis that adds a penalty to the least squares objective function in order to reduce the variance of the estimates and to induce sparsity in the model. This is done by adding a term to the objective function that is proportional to the sum of the absolute values of the coefficients. The penalty term is called the "lasso" penalty, and it is controlled by a parameter called the "lasso constant". Lasso regression can be used to address the problem of multicollinearity in linear regression, as well as the problem of overfitting. Multicollinearity occurs when two or more independent variables are highly correlated. This can cause the standard errors of the coefficients to be large, and it can also cause the coefficients to be unstable. Overfitting occurs when a model is too closely fit to the training data, and as a result, it does not generalize well to new data.
For further technical information as per how our model work we invite you to visit the article below:
How do AC Investment Research machine learning (predictive) algorithms actually work?
LIN Stock Forecast (Buy or Sell)
Sample Set: Neural NetworkStock/Index: LIN LINDIAN RESOURCES LIMITED
Time series to forecast: 4 Weeks
According to price forecasts, the dominant strategy among neural network is: Sell
Strategic Interaction Table Legend:
X axis: *Likelihood% (The higher the percentage value, the more likely the event will occur.)
Y axis: *Potential Impact% (The higher the percentage value, the more likely the price will deviate.)
Z axis (Grey to Black): *Technical Analysis%
Financial Data Adjustments for Transductive Learning (ML) based LIN Stock Prediction Model
- An entity may retain the right to a part of the interest payments on transferred assets as compensation for servicing those assets. The part of the interest payments that the entity would give up upon termination or transfer of the servicing contract is allocated to the servicing asset or servicing liability. The part of the interest payments that the entity would not give up is an interest-only strip receivable. For example, if the entity would not give up any interest upon termination or transfer of the servicing contract, the entire interest spread is an interest-only strip receivable. For the purposes of applying paragraph 3.2.13, the fair values of the servicing asset and interest-only strip receivable are used to allocate the carrying amount of the receivable between the part of the asset that is derecognised and the part that continues to be recognised. If there is no servicing fee specified or the fee to be received is not expected to compensate the entity adequately for performing the servicing, a liability for the servicing obligation is recognised at fair value.
- When designating a risk component as a hedged item, the hedge accounting requirements apply to that risk component in the same way as they apply to other hedged items that are not risk components. For example, the qualifying criteria apply, including that the hedging relationship must meet the hedge effectiveness requirements, and any hedge ineffectiveness must be measured and recognised.
- The credit risk on a financial instrument is considered low for the purposes of paragraph 5.5.10, if the financial instrument has a low risk of default, the borrower has a strong capacity to meet its contractual cash flow obligations in the near term and adverse changes in economic and business conditions in the longer term may, but will not necessarily, reduce the ability of the borrower to fulfil its contractual cash flow obligations. Financial instruments are not considered to have low credit risk when they are regarded as having a low risk of loss simply because of the value of collateral and the financial instrument without that collateral would not be considered low credit risk. Financial instruments are also not considered to have low credit risk simply because they have a lower risk of default than the entity's other financial instruments or relative to the credit risk of the jurisdiction within which an entity operates.
- An entity may use practical expedients when measuring expected credit losses if they are consistent with the principles in paragraph 5.5.17. An example of a practical expedient is the calculation of the expected credit losses on trade receivables using a provision matrix. The entity would use its historical credit loss experience (adjusted as appropriate in accordance with paragraphs B5.5.51–B5.5.52) for trade receivables to estimate the 12-month expected credit losses or the lifetime expected credit losses on the financial assets as relevant. A provision matrix might, for example, specify fixed provision rates depending on the number of days that a trade receivable is past due (for example, 1 per cent if not past due, 2 per cent if less than 30 days past due, 3 per cent if more than 30 days but less than 90 days past due, 20 per cent if 90–180 days past due etc). Depending on the diversity of its customer base, the entity would use appropriate groupings if its historical credit loss experience shows significantly different loss patterns for different customer segments. Examples of criteria that might be used to group assets include geographical region, product type, customer rating, collateral or trade credit insurance and type of customer (such as wholesale or retail)
*International Financial Reporting Standards (IFRS) adjustment process involves reviewing the company's financial statements and identifying any differences between the company's current accounting practices and the requirements of the IFRS. If there are any such differences, neural network makes adjustments to financial statements to bring them into compliance with the IFRS.
LIN LINDIAN RESOURCES LIMITED Financial Analysis*
Rating | Short-Term | Long-Term Senior |
---|---|---|
Outlook* | B3 | B2 |
Income Statement | B2 | Caa2 |
Balance Sheet | Ba3 | Caa2 |
Leverage Ratios | Caa2 | C |
Cash Flow | C | Baa2 |
Rates of Return and Profitability | C | Caa2 |
*Financial analysis is the process of evaluating a company's financial performance and position by neural network. It involves reviewing the company's financial statements, including the balance sheet, income statement, and cash flow statement, as well as other financial reports and documents.
How does neural network examine financial reports and understand financial state of the company?
Conclusions
LINDIAN RESOURCES LIMITED is assigned short-term B3 & long-term B2 estimated rating. LINDIAN RESOURCES LIMITED prediction model is evaluated with Transductive Learning (ML) and Lasso Regression1,2,3,4 and it is concluded that the LIN stock is predictable in the short/long term. According to price forecasts for 4 Weeks period, the dominant strategy among neural network is: Sell
Prediction Confidence Score
References
- M. Ono, M. Pavone, Y. Kuwata, and J. Balaram. Chance-constrained dynamic programming with application to risk-aware robotic space exploration. Autonomous Robots, 39(4):555–571, 2015
- Burgess, D. F. (1975), "Duality theory and pitfalls in the specification of technologies," Journal of Econometrics, 3, 105–121.
- Bertsimas D, King A, Mazumder R. 2016. Best subset selection via a modern optimization lens. Ann. Stat. 44:813–52
- M. Sobel. The variance of discounted Markov decision processes. Applied Probability, pages 794–802, 1982
- K. Boda, J. Filar, Y. Lin, and L. Spanjers. Stochastic target hitting time and the problem of early retirement. Automatic Control, IEEE Transactions on, 49(3):409–419, 2004
- Batchelor, R. P. Dua (1993), "Survey vs ARCH measures of inflation uncertainty," Oxford Bulletin of Economics Statistics, 55, 341–353.
- S. Bhatnagar. An actor-critic algorithm with function approximation for discounted cost constrained Markov decision processes. Systems & Control Letters, 59(12):760–766, 2010
Frequently Asked Questions
Q: What is the prediction methodology for LIN stock?A: LIN stock prediction methodology: We evaluate the prediction models Transductive Learning (ML) and Lasso Regression
Q: Is LIN stock a buy or sell?
A: The dominant strategy among neural network is to Sell LIN Stock.
Q: Is LINDIAN RESOURCES LIMITED stock a good investment?
A: The consensus rating for LINDIAN RESOURCES LIMITED is Sell and is assigned short-term B3 & long-term B2 estimated rating.
Q: What is the consensus rating of LIN stock?
A: The consensus rating for LIN is Sell.
Q: What is the prediction period for LIN stock?
A: The prediction period for LIN is 4 Weeks