AUC Score :
Short-Term Revised1 :
Dominant Strategy : Hold
Time series to forecast n:
Methodology : Modular Neural Network (Emotional Trigger/Responses Analysis)
Hypothesis Testing : Beta
Surveillance : Major exchange and OTC
1The accuracy of the model is being monitored on a regular basis.(15-minute period)
2Time series is updated based on short-term trends.
Summary
SL Green Realty Corporation Preferred Series I prediction model is evaluated with Modular Neural Network (Emotional Trigger/Responses Analysis) and Beta1,2,3,4 and it is concluded that the SLG^I stock is predictable in the short/long term. A modular neural network (MNN) is a type of artificial neural network that can be used for emotional trigger/responses analysis. MNNs are made up of multiple smaller neural networks, called modules. Each module is responsible for learning a specific task, such as identifying sentiment in text or identifying patterns in data. The modules are then combined to form a single neural network that can perform multiple tasks. In the context of emotional trigger/responses analysis, MNNs can be used to identify the emotional triggers that cause people to experience certain emotions, and to identify the responses that people typically exhibit when they experience those emotions. This information can then be used to develop more effective emotional support systems, to improve the accuracy of artificial intelligence systems, and to create more engaging and immersive entertainment experiences. According to price forecasts for 3 Month period, the dominant strategy among neural network is: Hold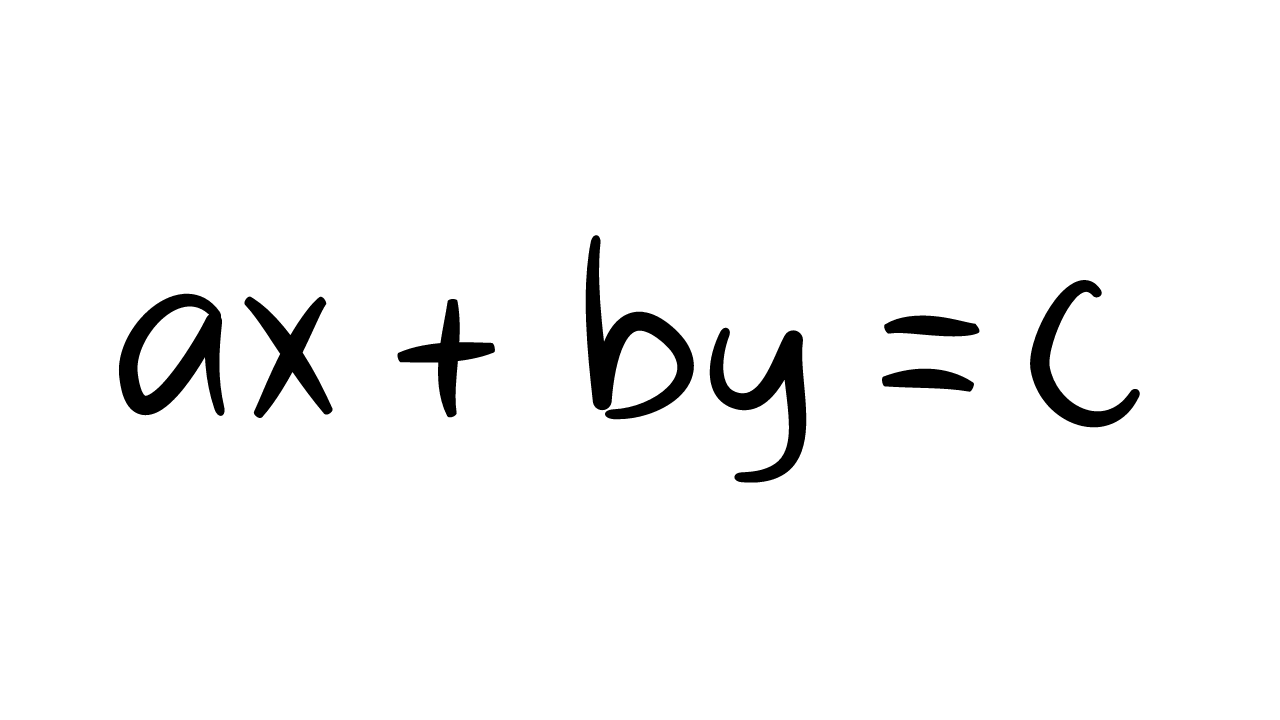
Key Points
- What is the best way to predict stock prices?
- Stock Forecast Based On a Predictive Algorithm
- What is the use of Markov decision process?
SLG^I Target Price Prediction Modeling Methodology
We consider SL Green Realty Corporation Preferred Series I Decision Process with Modular Neural Network (Emotional Trigger/Responses Analysis) where A is the set of discrete actions of SLG^I stock holders, F is the set of discrete states, P : S × F × S → R is the transition probability distribution, R : S × F → R is the reaction function, and γ ∈ [0, 1] is a move factor for expectation.1,2,3,4
F(Beta)5,6,7= X R(Modular Neural Network (Emotional Trigger/Responses Analysis)) X S(n):→ 3 Month
n:Time series to forecast
p:Price signals of SLG^I stock
j:Nash equilibria (Neural Network)
k:Dominated move
a:Best response for target price
Modular Neural Network (Emotional Trigger/Responses Analysis)
A modular neural network (MNN) is a type of artificial neural network that can be used for emotional trigger/responses analysis. MNNs are made up of multiple smaller neural networks, called modules. Each module is responsible for learning a specific task, such as identifying sentiment in text or identifying patterns in data. The modules are then combined to form a single neural network that can perform multiple tasks. In the context of emotional trigger/responses analysis, MNNs can be used to identify the emotional triggers that cause people to experience certain emotions, and to identify the responses that people typically exhibit when they experience those emotions. This information can then be used to develop more effective emotional support systems, to improve the accuracy of artificial intelligence systems, and to create more engaging and immersive entertainment experiences.Beta
In statistics, beta (β) is a measure of the strength of the relationship between two variables. It is calculated as the slope of the line of best fit in a regression analysis. Beta can range from -1 to 1, with a value of 0 indicating no relationship between the two variables. A positive beta indicates that as one variable increases, the other variable also increases. A negative beta indicates that as one variable increases, the other variable decreases. For example, a study might find that there is a positive relationship between height and weight. This means that taller people tend to weigh more. The beta coefficient for this relationship would be positive.
For further technical information as per how our model work we invite you to visit the article below:
How do AC Investment Research machine learning (predictive) algorithms actually work?
SLG^I Stock Forecast (Buy or Sell)
Sample Set: Neural NetworkStock/Index: SLG^I SL Green Realty Corporation Preferred Series I
Time series to forecast: 3 Month
According to price forecasts, the dominant strategy among neural network is: Hold
Strategic Interaction Table Legend:
X axis: *Likelihood% (The higher the percentage value, the more likely the event will occur.)
Y axis: *Potential Impact% (The higher the percentage value, the more likely the price will deviate.)
Z axis (Grey to Black): *Technical Analysis%
Financial Data Adjustments for Modular Neural Network (Emotional Trigger/Responses Analysis) based SLG^I Stock Prediction Model
- An entity may use practical expedients when measuring expected credit losses if they are consistent with the principles in paragraph 5.5.17. An example of a practical expedient is the calculation of the expected credit losses on trade receivables using a provision matrix. The entity would use its historical credit loss experience (adjusted as appropriate in accordance with paragraphs B5.5.51–B5.5.52) for trade receivables to estimate the 12-month expected credit losses or the lifetime expected credit losses on the financial assets as relevant. A provision matrix might, for example, specify fixed provision rates depending on the number of days that a trade receivable is past due (for example, 1 per cent if not past due, 2 per cent if less than 30 days past due, 3 per cent if more than 30 days but less than 90 days past due, 20 per cent if 90–180 days past due etc). Depending on the diversity of its customer base, the entity would use appropriate groupings if its historical credit loss experience shows significantly different loss patterns for different customer segments. Examples of criteria that might be used to group assets include geographical region, product type, customer rating, collateral or trade credit insurance and type of customer (such as wholesale or retail)
- However, in some cases, the time value of money element may be modified (ie imperfect). That would be the case, for example, if a financial asset's interest rate is periodically reset but the frequency of that reset does not match the tenor of the interest rate (for example, the interest rate resets every month to a one-year rate) or if a financial asset's interest rate is periodically reset to an average of particular short- and long-term interest rates. In such cases, an entity must assess the modification to determine whether the contractual cash flows represent solely payments of principal and interest on the principal amount outstanding. In some circumstances, the entity may be able to make that determination by performing a qualitative assessment of the time value of money element whereas, in other circumstances, it may be necessary to perform a quantitative assessment.
- For example, an entity may use this condition to designate financial liabilities as at fair value through profit or loss if it meets the principle in paragraph 4.2.2(b) and the entity has financial assets and financial liabilities that share one or more risks and those risks are managed and evaluated on a fair value basis in accordance with a documented policy of asset and liability management. An example could be an entity that has issued 'structured products' containing multiple embedded derivatives and manages the resulting risks on a fair value basis using a mix of derivative and non-derivative financial instruments
- Annual Improvements to IFRSs 2010–2012 Cycle, issued in December 2013, amended paragraphs 4.2.1 and 5.7.5 as a consequential amendment derived from the amendment to IFRS 3. An entity shall apply that amendment prospectively to business combinations to which the amendment to IFRS 3 applies.
*International Financial Reporting Standards (IFRS) adjustment process involves reviewing the company's financial statements and identifying any differences between the company's current accounting practices and the requirements of the IFRS. If there are any such differences, neural network makes adjustments to financial statements to bring them into compliance with the IFRS.
SLG^I SL Green Realty Corporation Preferred Series I Financial Analysis*
Rating | Short-Term | Long-Term Senior |
---|---|---|
Outlook* | B1 | Ba3 |
Income Statement | Baa2 | Baa2 |
Balance Sheet | Caa2 | Baa2 |
Leverage Ratios | Caa2 | B2 |
Cash Flow | Ba2 | B1 |
Rates of Return and Profitability | Ba3 | B3 |
*Financial analysis is the process of evaluating a company's financial performance and position by neural network. It involves reviewing the company's financial statements, including the balance sheet, income statement, and cash flow statement, as well as other financial reports and documents.
How does neural network examine financial reports and understand financial state of the company?
Conclusions
SL Green Realty Corporation Preferred Series I is assigned short-term B1 & long-term Ba3 estimated rating. SL Green Realty Corporation Preferred Series I prediction model is evaluated with Modular Neural Network (Emotional Trigger/Responses Analysis) and Beta1,2,3,4 and it is concluded that the SLG^I stock is predictable in the short/long term. According to price forecasts for 3 Month period, the dominant strategy among neural network is: Hold
Prediction Confidence Score
References
- Dimakopoulou M, Athey S, Imbens G. 2017. Estimation considerations in contextual bandits. arXiv:1711.07077 [stat.ML]
- J. G. Schneider, W. Wong, A. W. Moore, and M. A. Riedmiller. Distributed value functions. In Proceedings of the Sixteenth International Conference on Machine Learning (ICML 1999), Bled, Slovenia, June 27 - 30, 1999, pages 371–378, 1999.
- Bell RM, Koren Y. 2007. Lessons from the Netflix prize challenge. ACM SIGKDD Explor. Newsl. 9:75–79
- Zeileis A, Hothorn T, Hornik K. 2008. Model-based recursive partitioning. J. Comput. Graph. Stat. 17:492–514 Zhou Z, Athey S, Wager S. 2018. Offline multi-action policy learning: generalization and optimization. arXiv:1810.04778 [stat.ML]
- Meinshausen N. 2007. Relaxed lasso. Comput. Stat. Data Anal. 52:374–93
- Babula, R. A. (1988), "Contemporaneous correlation and modeling Canada's imports of U.S. crops," Journal of Agricultural Economics Research, 41, 33–38.
- H. Kushner and G. Yin. Stochastic approximation algorithms and applications. Springer, 1997.
Frequently Asked Questions
Q: What is the prediction methodology for SLG^I stock?A: SLG^I stock prediction methodology: We evaluate the prediction models Modular Neural Network (Emotional Trigger/Responses Analysis) and Beta
Q: Is SLG^I stock a buy or sell?
A: The dominant strategy among neural network is to Hold SLG^I Stock.
Q: Is SL Green Realty Corporation Preferred Series I stock a good investment?
A: The consensus rating for SL Green Realty Corporation Preferred Series I is Hold and is assigned short-term B1 & long-term Ba3 estimated rating.
Q: What is the consensus rating of SLG^I stock?
A: The consensus rating for SLG^I is Hold.
Q: What is the prediction period for SLG^I stock?
A: The prediction period for SLG^I is 3 Month