AUC Score :
Dominant Strategy :
Time series to forecast n:
Methodology : Supervised Machine Learning (ML)
Hypothesis Testing : Stepwise Regression
Surveillance : Major exchange and OTC
Summary
A2Z Smart Technologies Corp. prediction model is evaluated with Supervised Machine Learning (ML) and Stepwise Regression1,2,3,4 and it is concluded that the AZ:TSXV stock is predictable in the short/long term. Supervised machine learning (ML) is a type of machine learning where a model is trained on labeled data. This means that the data has been tagged with the correct output for the input data. The model learns to predict the output for new input data based on the labeled data. Supervised ML is a powerful tool that can be used for a variety of tasks, including classification, regression, and forecasting. Classification tasks involve predicting the category of an input data, such as whether an email is spam or not. Regression tasks involve predicting a numerical value for an input data, such as the price of a house. Forecasting tasks involve predicting future values for a time series, such as the sales of a product. According to price forecasts for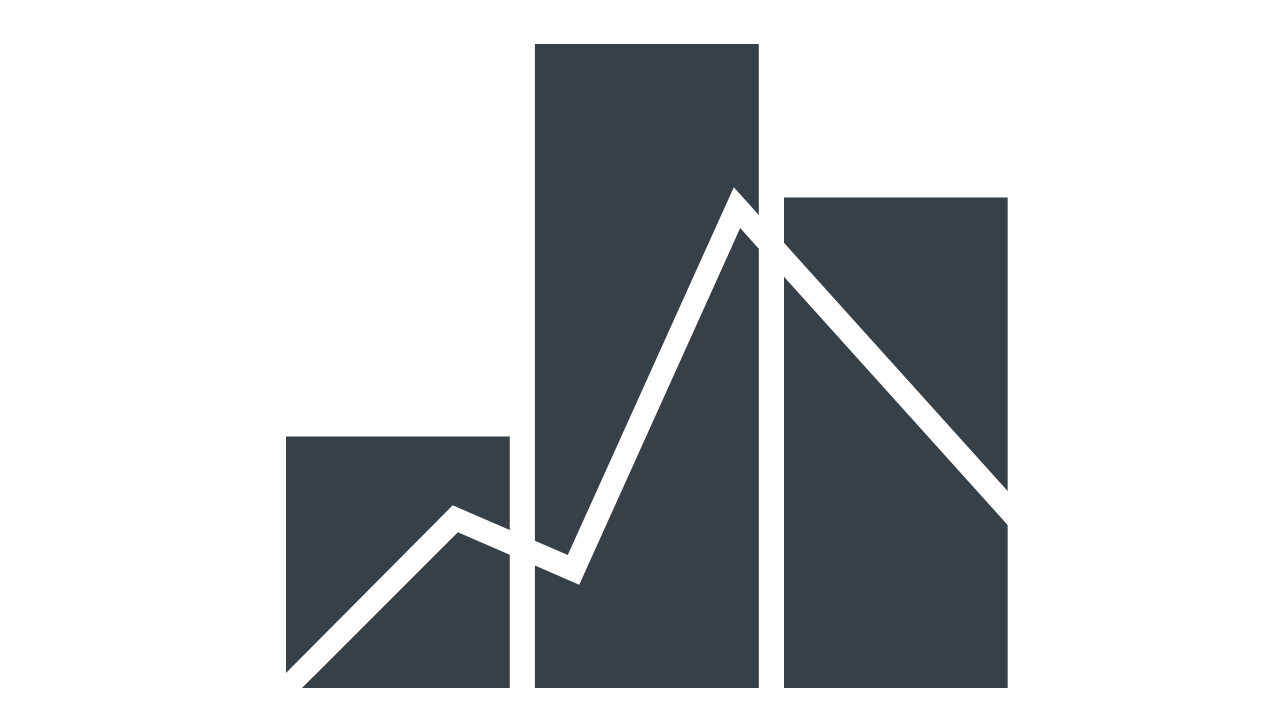
Key Points
- Short/Long Term Stocks
- Prediction Modeling
- Reaction Function
AZ:TSXV Target Price Prediction Modeling Methodology
We consider A2Z Smart Technologies Corp. Decision Process with Supervised Machine Learning (ML) where A is the set of discrete actions of AZ:TSXV stock holders, F is the set of discrete states, P : S × F × S → R is the transition probability distribution, R : S × F → R is the reaction function, and γ ∈ [0, 1] is a move factor for expectation.1,2,3,4
F(Stepwise Regression)5,6,7= X R(Supervised Machine Learning (ML)) X S(n):→ 4 Weeks
n:Time series to forecast
p:Price signals of AZ:TSXV stock
j:Nash equilibria (Neural Network)
k:Dominated move
a:Best response for target price
Supervised Machine Learning (ML)
Supervised machine learning (ML) is a type of machine learning where a model is trained on labeled data. This means that the data has been tagged with the correct output for the input data. The model learns to predict the output for new input data based on the labeled data. Supervised ML is a powerful tool that can be used for a variety of tasks, including classification, regression, and forecasting. Classification tasks involve predicting the category of an input data, such as whether an email is spam or not. Regression tasks involve predicting a numerical value for an input data, such as the price of a house. Forecasting tasks involve predicting future values for a time series, such as the sales of a product.Stepwise Regression
Stepwise regression is a method of variable selection in which variables are added or removed from a model one at a time, based on their statistical significance. There are two main types of stepwise regression: forward selection and backward elimination. In forward selection, variables are added to the model one at a time, starting with the variable with the highest F-statistic. The F-statistic is a measure of how much improvement in the model is gained by adding the variable. Variables are added to the model until no variable adds a statistically significant improvement to the model.
For further technical information as per how our model work we invite you to visit the article below:
How do AC Investment Research machine learning (predictive) algorithms actually work?
AZ:TSXV Stock Forecast (Buy or Sell)
Sample Set: Neural NetworkStock/Index: AZ:TSXV A2Z Smart Technologies Corp.
Time series to forecast:
According to price forecasts,the dominant strategy among neural network is:
Strategic Interaction Table Legend:
X axis: *Likelihood% (The higher the percentage value, the more likely the event will occur.)
Y axis: *Potential Impact% (The higher the percentage value, the more likely the price will deviate.)
Z axis (Grey to Black): *Technical Analysis%
Financial Data Adjustments for Supervised Machine Learning (ML) based AZ:TSXV Stock Prediction Model
- The fact that a derivative is in or out of the money when it is designated as a hedging instrument does not in itself mean that a qualitative assessment is inappropriate. It depends on the circumstances whether hedge ineffectiveness arising from that fact could have a magnitude that a qualitative assessment would not adequately capture.
- In some circumstances, the renegotiation or modification of the contractual cash flows of a financial asset can lead to the derecognition of the existing financial asset in accordance with this Standard. When the modification of a financial asset results in the derecognition of the existing financial asset and the subsequent recognition of the modified financial asset, the modified asset is considered a 'new' financial asset for the purposes of this Standard.
- If the underlyings are not the same but are economically related, there can be situations in which the values of the hedging instrument and the hedged item move in the same direction, for example, because the price differential between the two related underlyings changes while the underlyings themselves do not move significantly. That is still consistent with an economic relationship between the hedging instrument and the hedged item if the values of the hedging instrument and the hedged item are still expected to typically move in the opposite direction when the underlyings move.
- Adjusting the hedge ratio by decreasing the volume of the hedged item does not affect how the changes in the fair value of the hedging instrument are measured. The measurement of the changes in the value of the hedged item related to the volume that continues to be designated also remains unaffected. However, from the date of rebalancing, the volume by which the hedged item was decreased is no longer part of the hedging relationship. For example, if an entity originally hedged a volume of 100 tonnes of a commodity at a forward price of CU80 and reduces that volume by 10 tonnes on rebalancing, the hedged item after rebalancing would be 90 tonnes hedged at CU80. The 10 tonnes of the hedged item that are no longer part of the hedging relationship would be accounted for in accordance with the requirements for the discontinuation of hedge accounting (see paragraphs 6.5.6–6.5.7 and B6.5.22–B6.5.28).
*International Financial Reporting Standards (IFRS) adjustment process involves reviewing the company's financial statements and identifying any differences between the company's current accounting practices and the requirements of the IFRS. If there are any such differences, neural network makes adjustments to financial statements to bring them into compliance with the IFRS.
AZ:TSXV A2Z Smart Technologies Corp. Financial Analysis*
Rating | Short-Term | Long-Term Senior |
---|---|---|
Outlook* | Caa2 | Ba3 |
Income Statement | C | B3 |
Balance Sheet | Ba2 | B2 |
Leverage Ratios | Caa2 | Caa2 |
Cash Flow | Caa2 | Baa2 |
Rates of Return and Profitability | C | Baa2 |
*Financial analysis is the process of evaluating a company's financial performance and position by neural network. It involves reviewing the company's financial statements, including the balance sheet, income statement, and cash flow statement, as well as other financial reports and documents.
How does neural network examine financial reports and understand financial state of the company?
Conclusions
A2Z Smart Technologies Corp. is assigned short-term Caa2 & long-term Ba3 estimated rating. A2Z Smart Technologies Corp. prediction model is evaluated with Supervised Machine Learning (ML) and Stepwise Regression1,2,3,4 and it is concluded that the AZ:TSXV stock is predictable in the short/long term. According to price forecasts for
Prediction Confidence Score
References
- Wu X, Kumar V, Quinlan JR, Ghosh J, Yang Q, et al. 2008. Top 10 algorithms in data mining. Knowl. Inform. Syst. 14:1–37
- A. K. Agogino and K. Tumer. Analyzing and visualizing multiagent rewards in dynamic and stochastic environments. Journal of Autonomous Agents and Multi-Agent Systems, 17(2):320–338, 2008
- Burkov A. 2019. The Hundred-Page Machine Learning Book. Quebec City, Can.: Andriy Burkov
- Christou, C., P. A. V. B. Swamy G. S. Tavlas (1996), "Modelling optimal strategies for the allocation of wealth in multicurrency investments," International Journal of Forecasting, 12, 483–493.
- Friedberg R, Tibshirani J, Athey S, Wager S. 2018. Local linear forests. arXiv:1807.11408 [stat.ML]
- A. Tamar and S. Mannor. Variance adjusted actor critic algorithms. arXiv preprint arXiv:1310.3697, 2013.
- Li L, Chu W, Langford J, Moon T, Wang X. 2012. An unbiased offline evaluation of contextual bandit algo- rithms with generalized linear models. In Proceedings of 4th ACM International Conference on Web Search and Data Mining, pp. 297–306. New York: ACM
Frequently Asked Questions
Q: What is the prediction methodology for AZ:TSXV stock?A: AZ:TSXV stock prediction methodology: We evaluate the prediction models Supervised Machine Learning (ML) and Stepwise Regression
Q: Is AZ:TSXV stock a buy or sell?
A: The dominant strategy among neural network is to Buy AZ:TSXV Stock.
Q: Is A2Z Smart Technologies Corp. stock a good investment?
A: The consensus rating for A2Z Smart Technologies Corp. is Buy and is assigned short-term Caa2 & long-term Ba3 estimated rating.
Q: What is the consensus rating of AZ:TSXV stock?
A: The consensus rating for AZ:TSXV is Buy.
Q: What is the prediction period for AZ:TSXV stock?
A: The prediction period for AZ:TSXV is 4 Weeks