AUC Score :
Short-Term Revised :
Dominant Strategy : Buy
Time series to forecast n:
Methodology : Supervised Machine Learning (ML)
Hypothesis Testing : Paired T-Test
Surveillance : Major exchange and OTC
Abstract
FRENKEL TOPPING GROUP PLC prediction model is evaluated with Supervised Machine Learning (ML) and Paired T-Test1,2,3,4 and it is concluded that the LON:FEN stock is predictable in the short/long term. Supervised machine learning (ML) is a type of machine learning where a model is trained on labeled data. This means that the data has been tagged with the correct output for the input data. The model learns to predict the output for new input data based on the labeled data. Supervised ML is a powerful tool that can be used for a variety of tasks, including classification, regression, and forecasting. Classification tasks involve predicting the category of an input data, such as whether an email is spam or not. Regression tasks involve predicting a numerical value for an input data, such as the price of a house. Forecasting tasks involve predicting future values for a time series, such as the sales of a product. According to price forecasts for 6 Month period, the dominant strategy among neural network is: Buy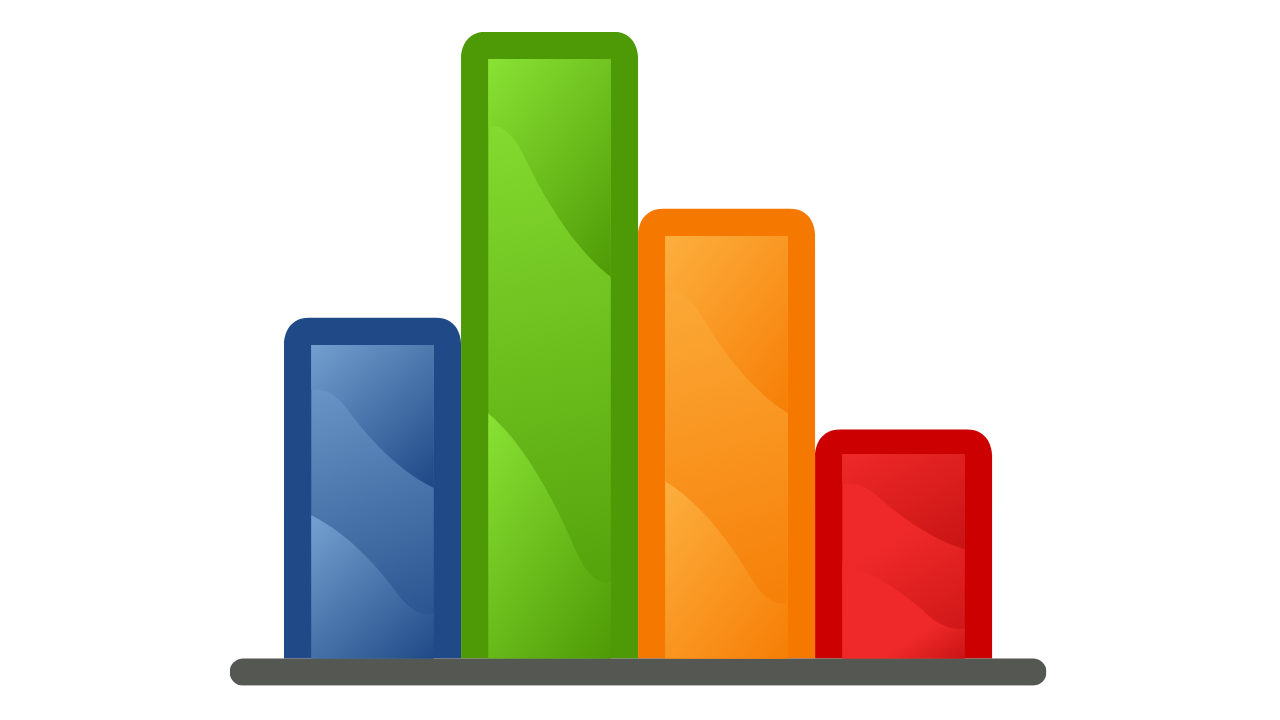
Key Points
- Market Outlook
- Market Signals
- Is Target price a good indicator?
LON:FEN Target Price Prediction Modeling Methodology
We consider FRENKEL TOPPING GROUP PLC Decision Process with Supervised Machine Learning (ML) where A is the set of discrete actions of LON:FEN stock holders, F is the set of discrete states, P : S × F × S → R is the transition probability distribution, R : S × F → R is the reaction function, and γ ∈ [0, 1] is a move factor for expectation.1,2,3,4
F(Paired T-Test)5,6,7= X R(Supervised Machine Learning (ML)) X S(n):→ 6 Month
n:Time series to forecast
p:Price signals of LON:FEN stock
j:Nash equilibria (Neural Network)
k:Dominated move
a:Best response for target price
Supervised Machine Learning (ML)
Supervised machine learning (ML) is a type of machine learning where a model is trained on labeled data. This means that the data has been tagged with the correct output for the input data. The model learns to predict the output for new input data based on the labeled data. Supervised ML is a powerful tool that can be used for a variety of tasks, including classification, regression, and forecasting. Classification tasks involve predicting the category of an input data, such as whether an email is spam or not. Regression tasks involve predicting a numerical value for an input data, such as the price of a house. Forecasting tasks involve predicting future values for a time series, such as the sales of a product.Paired T-Test
A paired t-test is a statistical test that compares the means of two paired samples. In a paired t-test, each data point in one sample is paired with a data point in the other sample. The pairs are typically related in some way, such as before and after measurements, or measurements from the same subject under different conditions. The paired t-test is a parametric test, which means that it assumes that the data is normally distributed. The paired t-test is also a dependent samples test, which means that the data points in each pair are correlated.
For further technical information as per how our model work we invite you to visit the article below:
How do AC Investment Research machine learning (predictive) algorithms actually work?
LON:FEN Stock Forecast (Buy or Sell) for 6 Month
Sample Set: Neural NetworkStock/Index: LON:FEN FRENKEL TOPPING GROUP PLC
Time series to forecast: 6 Month
According to price forecasts for 6 Month period, the dominant strategy among neural network is: Buy
Strategic Interaction Table Legend:
X axis: *Likelihood% (The higher the percentage value, the more likely the event will occur.)
Y axis: *Potential Impact% (The higher the percentage value, the more likely the price will deviate.)
Z axis (Grey to Black): *Technical Analysis%
Financial Data Adjustments for Supervised Machine Learning (ML) based LON:FEN Stock Prediction Model
- If a put option obligation written by an entity or call option right held by an entity prevents a transferred asset from being derecognised and the entity measures the transferred asset at amortised cost, the associated liability is measured at its cost (ie the consideration received) adjusted for the amortisation of any difference between that cost and the gross carrying amount of the transferred asset at the expiration date of the option. For example, assume that the gross carrying amount of the asset on the date of the transfer is CU98 and that the consideration received is CU95. The gross carrying amount of the asset on the option exercise date will be CU100. The initial carrying amount of the associated liability is CU95 and the difference between CU95 and CU100 is recognised in profit or loss using the effective interest method. If the option is exercised, any difference between the carrying amount of the associated liability and the exercise price is recognised in profit or loss.
- If, in applying paragraph 7.2.44, an entity reinstates a discontinued hedging relationship, the entity shall read references in paragraphs 6.9.11 and 6.9.12 to the date the alternative benchmark rate is designated as a noncontractually specified risk component for the first time as referring to the date of initial application of these amendments (ie the 24-month period for that alternative benchmark rate designated as a non-contractually specified risk component begins from the date of initial application of these amendments).
- When an entity discontinues measuring the financial instrument that gives rise to the credit risk, or a proportion of that financial instrument, at fair value through profit or loss, that financial instrument's fair value at the date of discontinuation becomes its new carrying amount. Subsequently, the same measurement that was used before designating the financial instrument at fair value through profit or loss shall be applied (including amortisation that results from the new carrying amount). For example, a financial asset that had originally been classified as measured at amortised cost would revert to that measurement and its effective interest rate would be recalculated based on its new gross carrying amount on the date of discontinuing measurement at fair value through profit or loss.
- For the purpose of applying paragraph 6.5.11, at the point when an entity amends the description of a hedged item as required in paragraph 6.9.1(b), the amount accumulated in the cash flow hedge reserve shall be deemed to be based on the alternative benchmark rate on which the hedged future cash flows are determined.
*International Financial Reporting Standards (IFRS) adjustment process involves reviewing the company's financial statements and identifying any differences between the company's current accounting practices and the requirements of the IFRS. If there are any such differences, neural network makes adjustments to financial statements to bring them into compliance with the IFRS.
LON:FEN FRENKEL TOPPING GROUP PLC Financial Analysis*
Rating | Short-Term | Long-Term Senior |
---|---|---|
Outlook* | Ba2 | B1 |
Income Statement | Ba3 | Ba3 |
Balance Sheet | Baa2 | Caa2 |
Leverage Ratios | Ba1 | Ba3 |
Cash Flow | B1 | C |
Rates of Return and Profitability | Ba3 | Baa2 |
*Financial analysis is the process of evaluating a company's financial performance and position by neural network. It involves reviewing the company's financial statements, including the balance sheet, income statement, and cash flow statement, as well as other financial reports and documents.
How does neural network examine financial reports and understand financial state of the company?
Conclusions
FRENKEL TOPPING GROUP PLC is assigned short-term Ba2 & long-term B1 estimated rating. FRENKEL TOPPING GROUP PLC prediction model is evaluated with Supervised Machine Learning (ML) and Paired T-Test1,2,3,4 and it is concluded that the LON:FEN stock is predictable in the short/long term. According to price forecasts for 6 Month period, the dominant strategy among neural network is: Buy
Prediction Confidence Score
References
- Athey S. 2017. Beyond prediction: using big data for policy problems. Science 355:483–85
- V. Borkar. An actor-critic algorithm for constrained Markov decision processes. Systems & Control Letters, 54(3):207–213, 2005.
- L. Busoniu, R. Babuska, and B. D. Schutter. A comprehensive survey of multiagent reinforcement learning. IEEE Transactions of Systems, Man, and Cybernetics Part C: Applications and Reviews, 38(2), 2008.
- Athey S, Imbens G, Wager S. 2016a. Efficient inference of average treatment effects in high dimensions via approximate residual balancing. arXiv:1604.07125 [math.ST]
- Burkov A. 2019. The Hundred-Page Machine Learning Book. Quebec City, Can.: Andriy Burkov
- Abadir, K. M., K. Hadri E. Tzavalis (1999), "The influence of VAR dimensions on estimator biases," Econometrica, 67, 163–181.
- Breiman L. 1993. Better subset selection using the non-negative garotte. Tech. Rep., Univ. Calif., Berkeley
Frequently Asked Questions
Q: What is the prediction methodology for LON:FEN stock?A: LON:FEN stock prediction methodology: We evaluate the prediction models Supervised Machine Learning (ML) and Paired T-Test
Q: Is LON:FEN stock a buy or sell?
A: The dominant strategy among neural network is to Buy LON:FEN Stock.
Q: Is FRENKEL TOPPING GROUP PLC stock a good investment?
A: The consensus rating for FRENKEL TOPPING GROUP PLC is Buy and is assigned short-term Ba2 & long-term B1 estimated rating.
Q: What is the consensus rating of LON:FEN stock?
A: The consensus rating for LON:FEN is Buy.
Q: What is the prediction period for LON:FEN stock?
A: The prediction period for LON:FEN is 6 Month